A General Theory for Softmax Gating Multinomial Logistic Mixture of Experts
CoRR(2023)
摘要
Mixture-of-experts (MoE) model incorporates the power of multiple submodels via gating functions to achieve greater performance in numerous regression and classification applications. From a theoretical perspective, while there have been previous attempts to comprehend the behavior of that model under the regression settings through the convergence analysis of maximum likelihood estimation in the Gaussian MoE model, such analysis under the setting of a classification problem has remained missing in the literature. We close this gap by establishing the convergence rates of density estimation and parameter estimation in the softmax gating multinomial logistic MoE model. Notably, when part of the expert parameters vanish, these rates are shown to be slower than polynomial rates owing to an inherent interaction between the softmax gating and expert functions via partial differential equations. To address this issue, we propose using a novel class of modified softmax gating functions which transform the input value before delivering them to the gating functions. As a result, the previous interaction disappears and the parameter estimation rates are significantly improved.
更多查看译文
关键词
experts,mixture,general theory
AI 理解论文
溯源树
样例
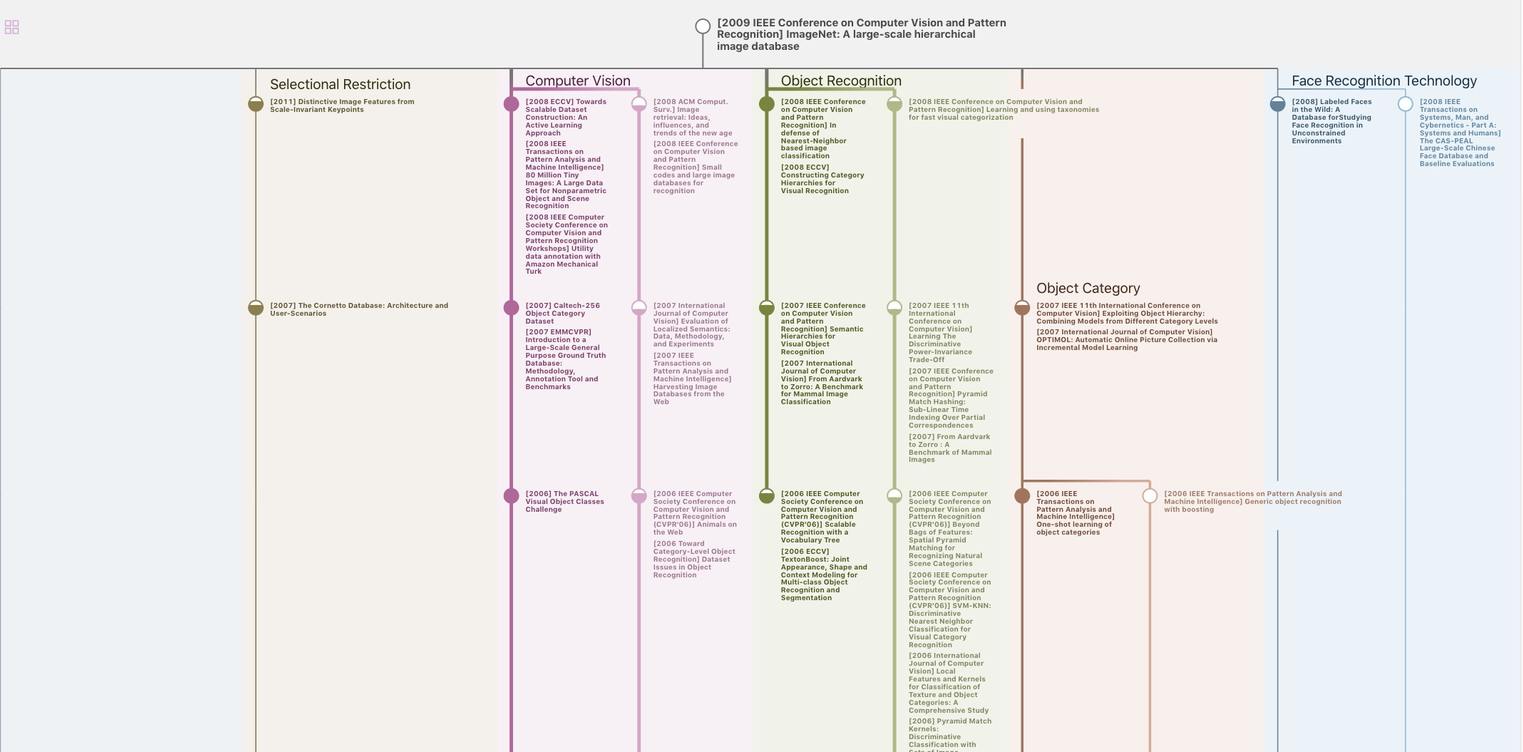
生成溯源树,研究论文发展脉络
Chat Paper
正在生成论文摘要