Hyperparameter optimization of hp-greedy reduced basis for gravitational wave surrogates
CoRR(2023)
摘要
In a previous work we introduced, in the context of gravitational wave science, an initial study on an automated domain-decomposition approach for reduced basis through hp-greedy refinement. The approach constructs local reduced bases of lower dimensionality than global ones, with the same or higher accuracy. These ``light'' local bases should imply both faster evaluations when predicting new waveforms and faster data analysis, in particular faster statistical inference (the forward and inverse problems, respectively). In this approach, however, we have previously found important dependence on several hyperparameters, which do not appear in global reduced basis. This naturally leads to the problem of hyperparameter optimization (HPO), which is the subject of this paper. We tackle the problem through a Bayesian optimization, and show its superiority when compared to grid or random searches. We find that for gravitational waves from the collision of two spinning but non-precessing black holes, for the same accuracy, local hp-greedy reduced bases with HPO have a lower dimensionality of up to $4 \times$ for the cases here studied, depending on the desired accuracy. This factor should directly translate in a parameter estimation speedup, for instance. Such acceleration might help in the near real-time requirements for electromagnetic counterparts of gravitational waves from compact binary coalescences. In addition, we find that the Bayesian approach used in this paper for HPO is two orders of magnitude faster than, for example, a grid search, with about a $100 \times$ acceleration. The code developed for this project is available as open source from public repositories.
更多查看译文
关键词
gravitational wave surrogates,reduced basis,machine learning
AI 理解论文
溯源树
样例
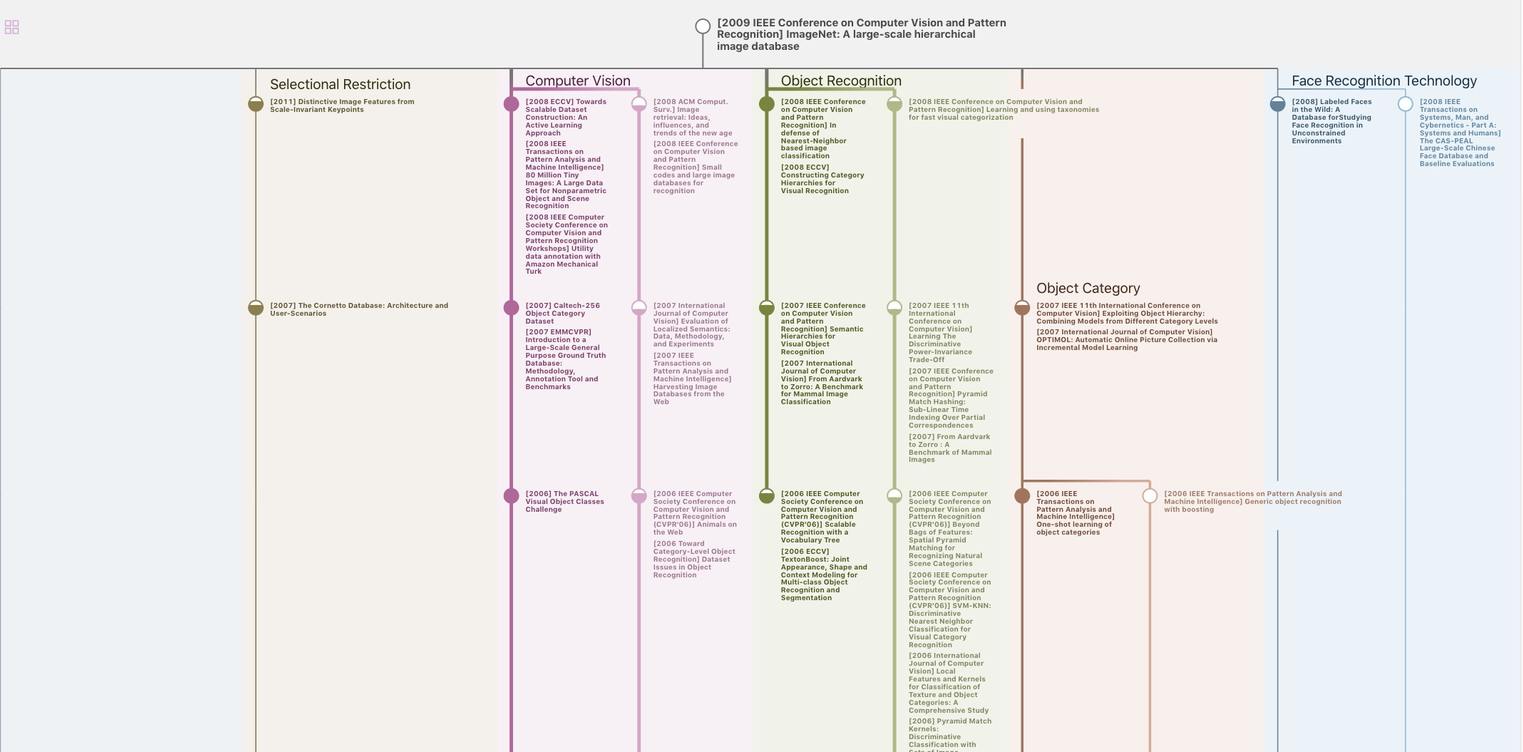
生成溯源树,研究论文发展脉络
Chat Paper
正在生成论文摘要