Advanced learning models for estimating the spatio-temporal variability of reference evapotranspiration using in-situ and ERA5-Land reanalysis data
Modeling Earth Systems and Environment(2024)
摘要
Reference Evapotranspiration (ET0) is a key parameter in hydrology. It has a variety of practical applications, ranging from understanding the hydrological cycle to estimating crop water needs for efficient irrigation in agriculture. The FAO PENMAN–MONTEITH (FAO-PM) method is largely adopted and is the recommended method by the FAO for ET0 estimation. However, it requires multiple measured meteorological parameters that are, in some conditions, difficult to obtain. This work fills this gap and leverages the potential of data-driven methods, namely machine learning and deep learning models, to accurately estimate ET0 while using a limited number of easy-to-obtain parameters. The study uses physical-based models (FAO-PM and Hargreaves) as ET0 learning data providers for data-driven models. First, physical models were fed with meteorological data covering the period 2013–2020 at an hourly scale to estimate ET0. The data were sourced from the in-situ local weather station of our study area in the Tensift basin (center of Morocco) and from the Copernicus ERA5-Land reanalysis data. Next, as a preprocessing step, feature engineering was performed using a decision tree-based approach. We evaluated the predictive importance of weather parameters for ET0 estimation. Scores between 0 and 1 were assigned to each parameter, indicating their efficacy. Notably, mean air temperature and global solar radiation stood out, collectively surpassing an 86% importance threshold. In contrast to the rest of the parameters that have a low importance of 10%. This emphasizes the critical significance of mean air temperature and global solar radiation as essential predictors for accurate ET0 estimation. Finally, during the modeling phase, three deep-learning models Long Short-Term Memory (LSTM), Gated Recurrent Unit, and Convolutional Neural Network are highlighted. Notably, the LSTM model exhibits superior performance, delivering comparable coefficients of determination (R 2 ) and root mean square error (RMSE) results, surpassing its counterparts. In terms of univariate predictions using the Hargreaves and FAO-56 PM methods, the LSTM consistently achieves high R 2 values of 0.90 across all data sources, accompanied by impressive accuracy reflected in low MAE, MSE, and RMSE values ranging from 0.004 to 0.07 mm/day. In addition, the ensemble learning model XGBoost had the best prediction performance with R 2 = 0.93 and RMSE = 0.03 mm/day. This confirms that machine learning models outperform deep learning architectures for small and medium-sized datasets. The proposed models will be integrated into an under-development agricultural decision support system.
更多查看译文
关键词
Evapotranspiration,Deep learning,Smart agriculture,FAO-56 Penman–Monteith,Hargreaves,ERA5-Land reanalysis data
AI 理解论文
溯源树
样例
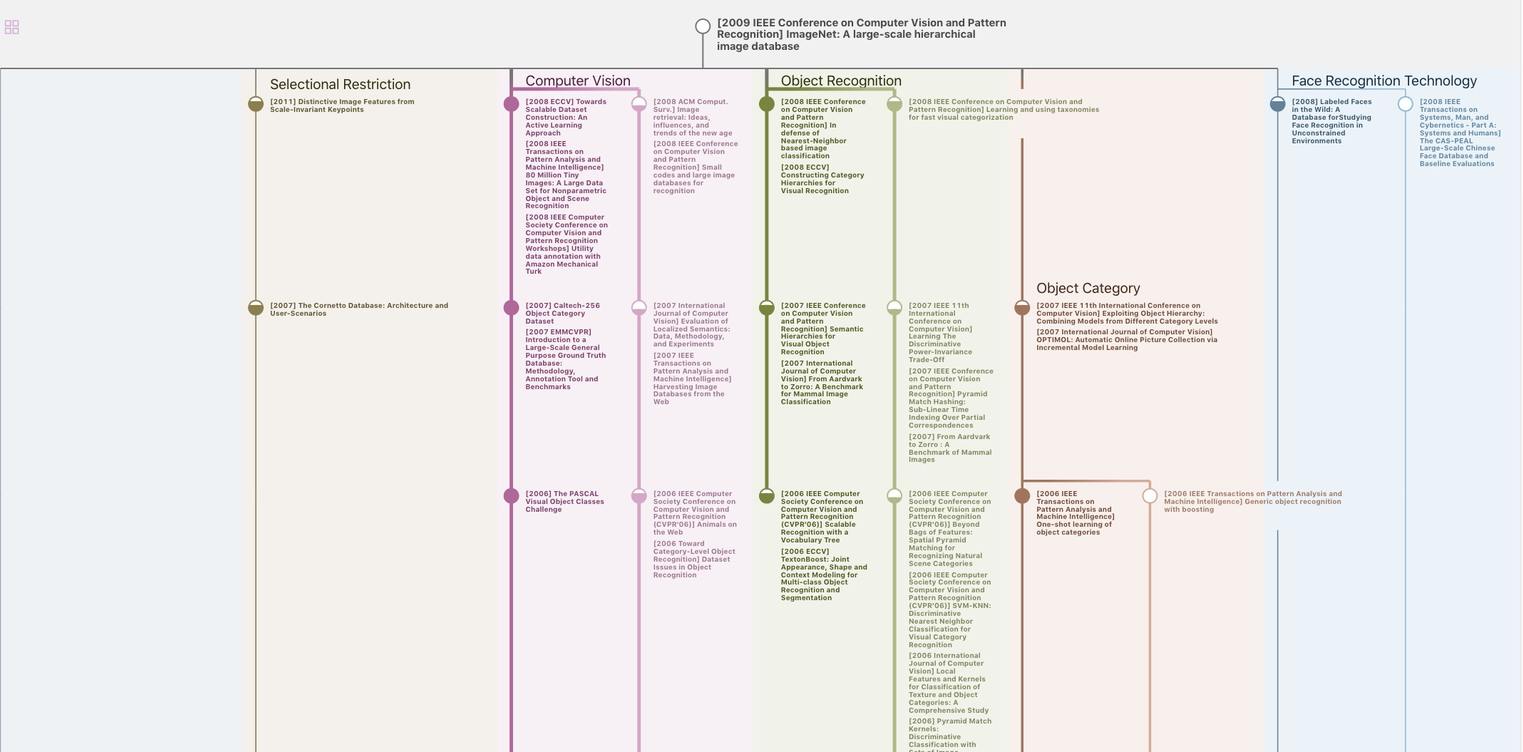
生成溯源树,研究论文发展脉络
Chat Paper
正在生成论文摘要