INTRAGRO: A machine learning approach to predict future growth of trees under climate change.
Ecology and evolution(2023)
摘要
The escalating impact of climate change on global terrestrial ecosystems demands a robust prediction of the trees' growth patterns and physiological adaptation for sustainable forestry and successful conservation efforts. Understanding these dynamics at an intra-annual resolution can offer deeper insights into tree responses under various future climate scenarios. However, the existing approaches to infer cambial or leaf phenological change are mainly focused on certain climatic zones (such as higher latitudes) or species with foliage discolouration during the fall season. In this study, we demonstrated a novel approach (INTRAGRO) to combine intra-annual circumference records generated by dendrometers coupled to the output of climate models to predict future tree growth at intra-annual resolution using a series of supervised and unsupervised machine learning algorithms. INTRAGRO performed well using our dataset, that is dendrometer data of Sarg. from the subtropical mid-elevation belt of Nepal, with robust test statistics. Our growth prediction shows enhanced tree growth at our study site for the middle and end of the 21st century. This result is remarkable since the predicted growing season by INTRAGRO is expected to shorten due to changes in seasonal precipitation. INTRAGRO's key advantage is the opportunity to analyse changes in trees' intra-annual growth dynamics on a global scale, regardless of the investigated tree species, regional climate and geographical conditions. Such information is important to assess tree species' growth performance and physiological adaptation to growing season change under different climate scenarios.
更多查看译文
关键词
dendrometer,growing‐season change,intra‐annual growth,machine learning algorithm,Pinus roxburghii,sub‐tropical Nepal
AI 理解论文
溯源树
样例
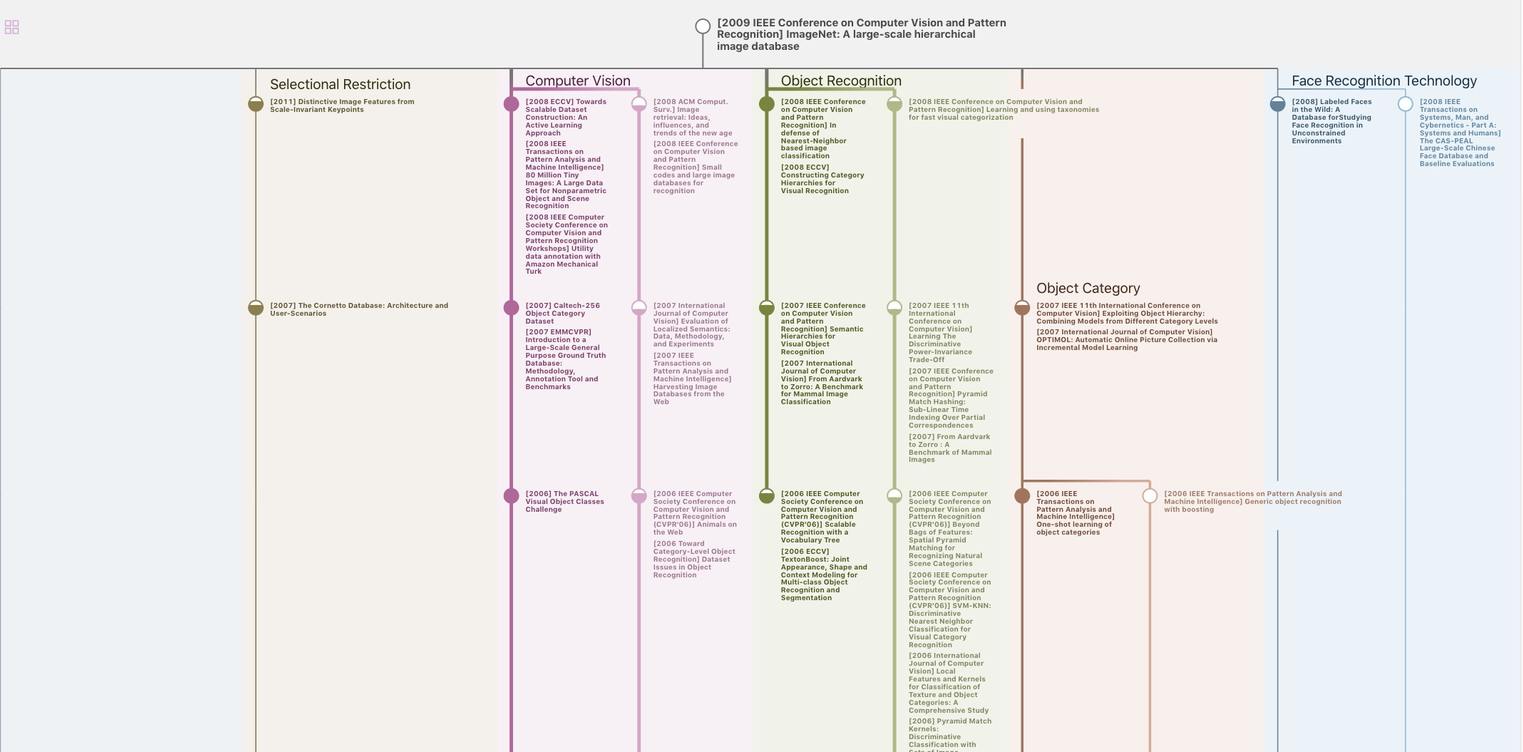
生成溯源树,研究论文发展脉络
Chat Paper
正在生成论文摘要