Deep Learning-Assisted Active Metamaterials with Heat-Enhanced Thermal Transport
ADVANCED MATERIALS(2024)
摘要
Heat management is crucial for state-of-the-art applications such as passive radiative cooling, thermally adjustable wearables, and camouflage systems. Their adaptive versions, to cater to varied requirements, lean on the potential of adaptive metamaterials. Existing efforts, however, feature with highly anisotropic parameters, narrow working-temperature ranges, and the need for manual intervention, which remain long-term and tricky obstacles for the most advanced self-adaptive metamaterials. To surmount these barriers, heat-enhanced thermal diffusion metamaterials powered by deep learning is introduced. Such active metamaterials can automatically sense ambient temperatures and swiftly, as well as continuously, adjust their thermal functions with a high degree of tunability. They maintain robust thermal performance even when external thermal fields change direction, and both simulations and experiments demonstrate exceptional results. Furthermore, two metadevices with on-demand adaptability, performing distinctive features with isotropic materials, wide working temperatures, and spontaneous response are designed. This work offers a framework for the design of intelligent thermal diffusion metamaterials and can be expanded to other diffusion fields, adapting to increasingly complex and dynamic environments. Drawing parallels from nonlinear optics, artificial intelligence assists a configurable nonlinear thermal material whose effective thermal conductivity being responsive to its temperature gradient. Such deep learning-assisted nonlinear thermal material promotes two typical self-adaptive devices, which can perceive their environment deeply. One maintains stable function, and another switches its function, in a changeable environment.image
更多查看译文
关键词
active metamaterials,deep learning,enhanced thermal conduction,self-adaptability
AI 理解论文
溯源树
样例
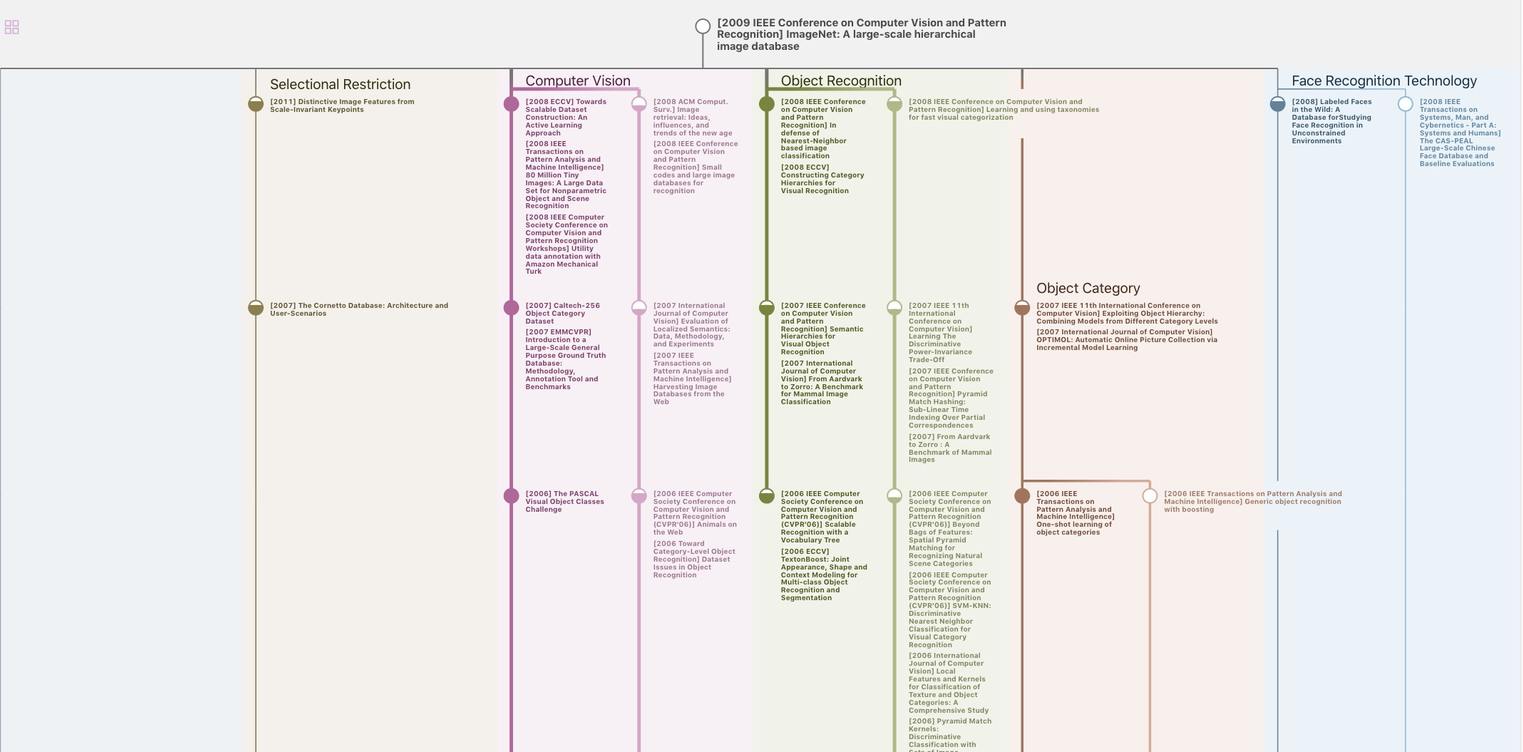
生成溯源树,研究论文发展脉络
Chat Paper
正在生成论文摘要