Digital Twin Assisted DAG Task Scheduling via Evolutionary Selection MARL in Large-Scale Mobile Edge Network
2023 IEEE INTERNATIONAL CONFERENCE ON COMMUNICATIONS WORKSHOPS, ICC WORKSHOPS(2023)
Abstract
6G is envisioned to serve many compute-intensive and latency-sensitive applications which are now usually formed by functional components in the directed acyclic graphic (DAG) form. Mobile edge computing (MEC) is proven a promising way to offload computing tasks and reduce execution latency. However, the ever-increasing number and heterogeneity of edge computing nodes, as well as the dynamically changing resource state, hinder any centralized scheduler from getting the accurate and timely resource state in the huge number of edge nodes. In this paper, facing a large-scale MEC scenario, we propose a digital twin (DT) assisted multiagent-based task scheduling scheme to jointly optimize task offloading and resource allocation strategy for DAG tasks. The scheme exploits the evolutionary selection multi-agent reinforcement learning (ES-MARL) framework to expedite the training of a large number of agents, while the DT of the mobile edge network keeps the latest state data and enables the centralized training of these distributed agents. The simulation result shows that the proposed scheme can achieve the goals of reducing latency and energy consumption while improving the scheduling success rate, load balancing index, and training efficiency.
MoreTranslated text
Key words
Mobile Edge Computing,Digital Twin,Task Scheduling,Evolutionary Selection,Multi-Agent Reinforcement Learning
AI Read Science
Must-Reading Tree
Example
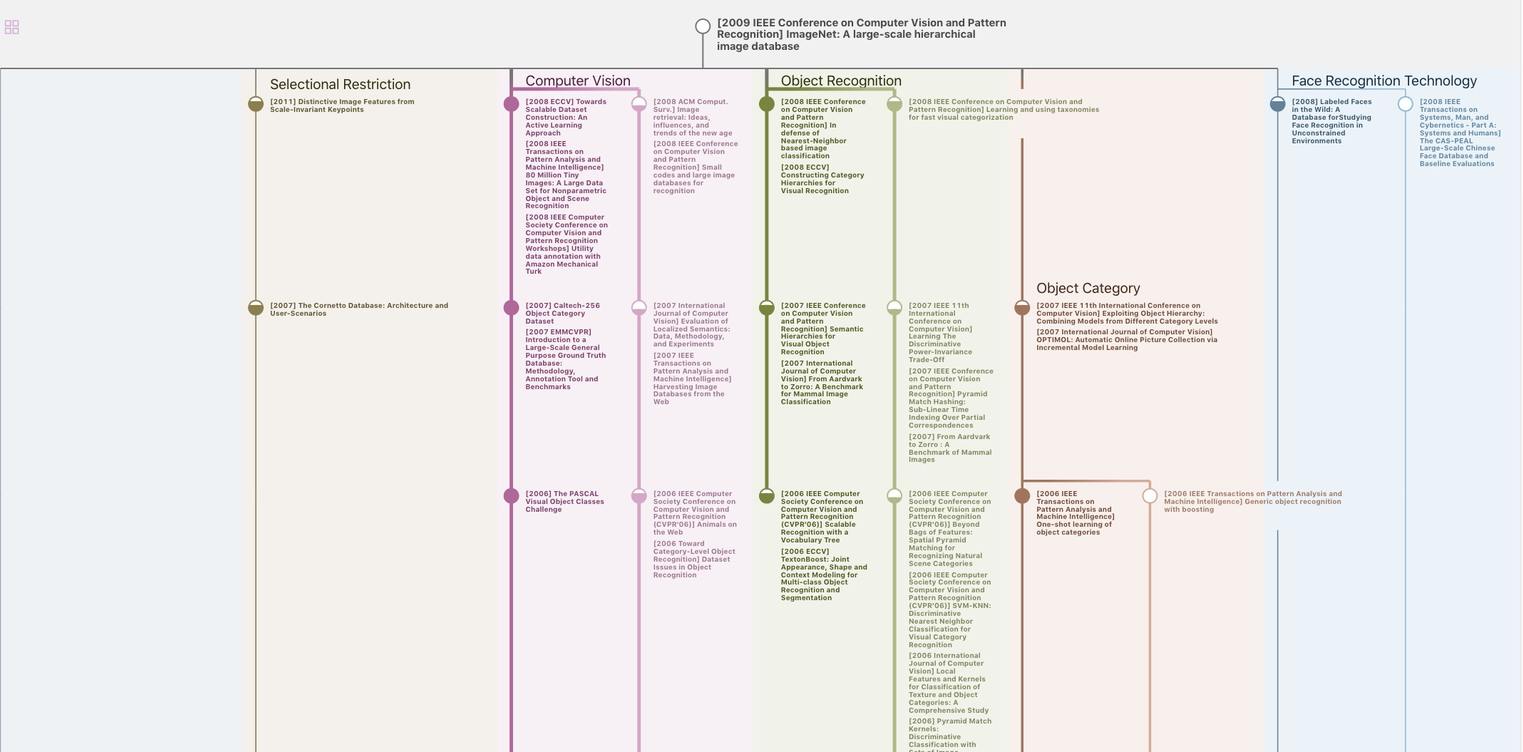
Generate MRT to find the research sequence of this paper
Chat Paper
Summary is being generated by the instructions you defined