Gflownets for Sensor Selection
2023 IEEE 33rd International Workshop on Machine Learning for Signal Processing (MLSP)(2023)
摘要
The efficacy of sensor arrays improves with more elements, yet increased number of elements leads to higher computational demands, cost and power consumption. Sparse arrays offer a cost-effective solution by utilizing only a subset of available elements. Each subset has a different effect on the performance properties of the array. This paper presents an unsupervised learning approach for sensor selection based on a deep generative modeling. The selection process is treated as a deterministic Markov Decision Process, where sensor subarrays arise as terminal states. The Generative Flow Network (GFlowNet) paradigm is employed to learn a distribution over actions based on the current state. Sampling from the aforementioned distribution ensures that the cumulative probability of reaching a terminal state is proportional to the sensing performance of the corresponding subset. The approach is applied for transmit beamforming where the performance of a subset is inversely proportional to the error between its corresponding beampattern and a desired beampattern. The method can generate multiple high-performing subsets by being trained on a small percentage of the possible subsets (less than 0.0001% of the possible subsets for the conducted experiments).
更多查看译文
关键词
sensor selection,GFlowNets,deep learning,deep generative modeling
AI 理解论文
溯源树
样例
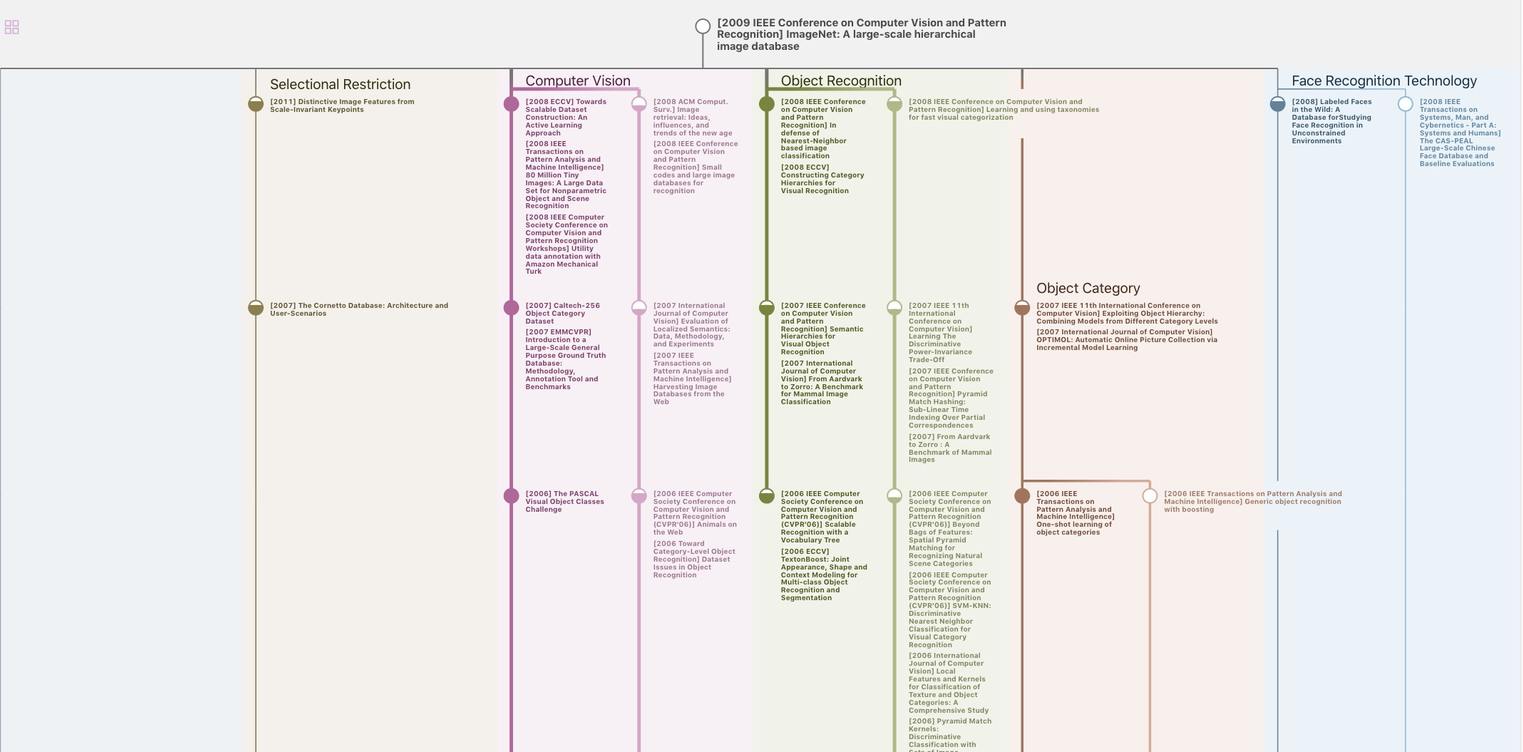
生成溯源树,研究论文发展脉络
Chat Paper
正在生成论文摘要