Federated Cooperative 3D Object Detection for Autonomous Driving
2023 IEEE 33rd International Workshop on Machine Learning for Signal Processing (MLSP)(2023)
摘要
Federated learning has shown great potential in improving the accuracy of models designed for connected autonomous vehicles (CAVs). However, existing approaches only focus on data collected by CAVs, ignoring the valuable insights provided by other types of clients, such as road-side units (RSUs). In this paper, we propose an approach that combines federated learning with cooperative perception to create a more comprehensive and robust global model. Our approach adopts a multi-layered structure that partitions CAVs and RSUs into local clusters. By incorporating the data captured by both CAVs and RSUs, this novel approach can lead to a more accurate and comprehensive global model that reflects the collective knowledge of all agents. We evaluate the proposed approach on the V2X-Set benchmark. The overall average precision of our approach using RSUs reaches 68.62% at Intersection-over-Union threshold of 0.5, significantly outperforming traditional CAV-based federated learning.
更多查看译文
关键词
Cooperative perception,federated learning,autonomous driving,vision transformer
AI 理解论文
溯源树
样例
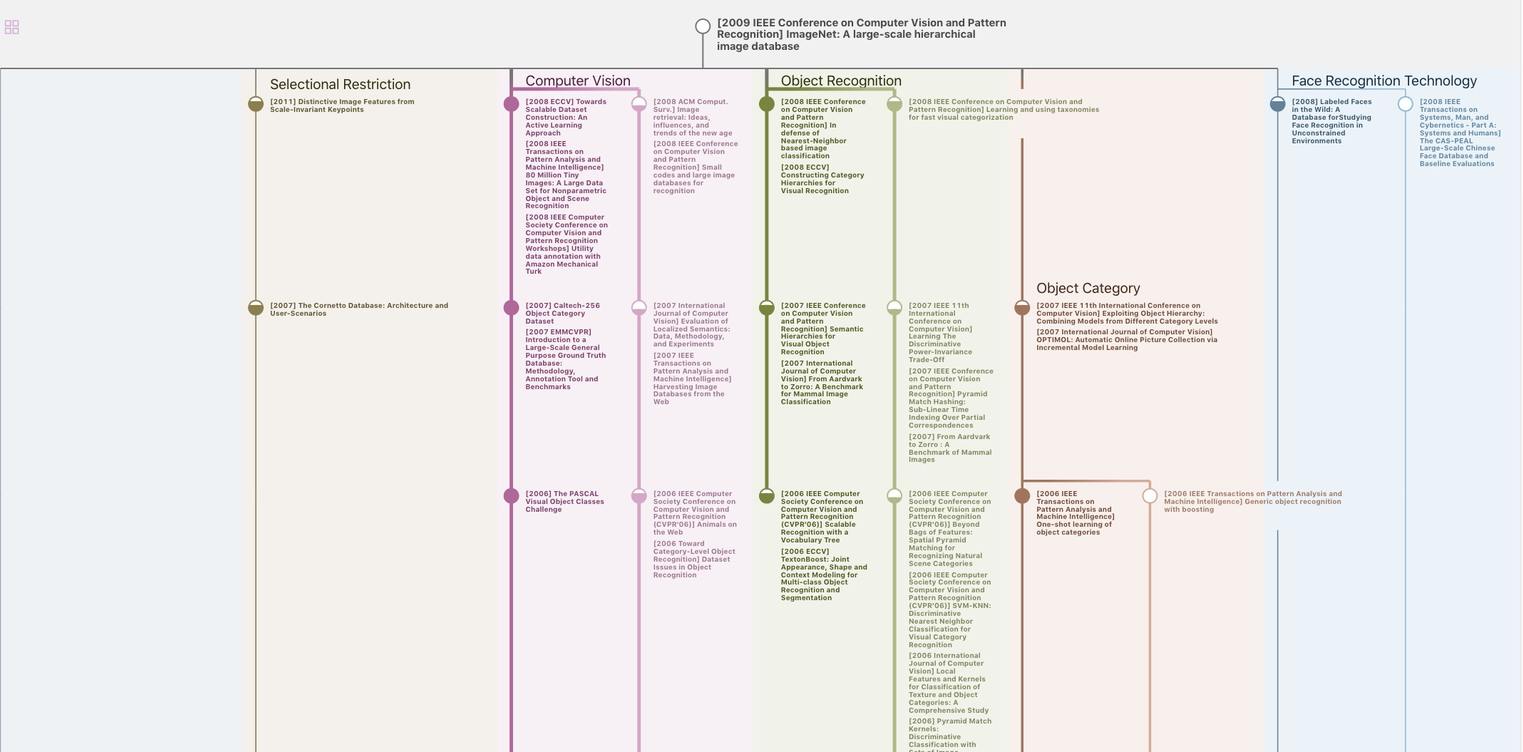
生成溯源树,研究论文发展脉络
Chat Paper
正在生成论文摘要