Incentivizing Edge AI with Accuracy Preserving via Online Randomized Auctions
2023 20th Annual IEEE International Conference on Sensing, Communication, and Networking (SECON)(2023)
摘要
Provisioning machine learning inference near the users at the network’s edge is emerging as a promising area for Edge AI. Due to the excessive energy consumption of edge devices, their owners often lack the motivation to actively contribute to their edge resources. To tackle this issue, we propose an incentive mechanism based on auctions, which enables edge device owners to submit their bids and compensates such bids via reward payments, ensuring the minimization of inference accuracy loss. We formulate a nonlinear mixed-integer program problem with the objective of minimizing the social cost, including accuracy loss cost, edge device cost, and service provider cost in the edge inference system. Then an Online Learning algorithm is devised to find the solutions, based on the primal-dual. To calculate the remuneration, we design a payment allocation algorithm based on the bid-winning probabilities. Our rigorous theoretical analysis shows that our algorithm designed achieves sub-linear growth on dynamic regret and dynamic fit over time while preserving the economic properties of truthfulness and individual rationality. Finally, multiple experiments validate the efficacy of the proposed auction mechanism algorithm from various perspectives compared with three other existing algorithms.
更多查看译文
AI 理解论文
溯源树
样例
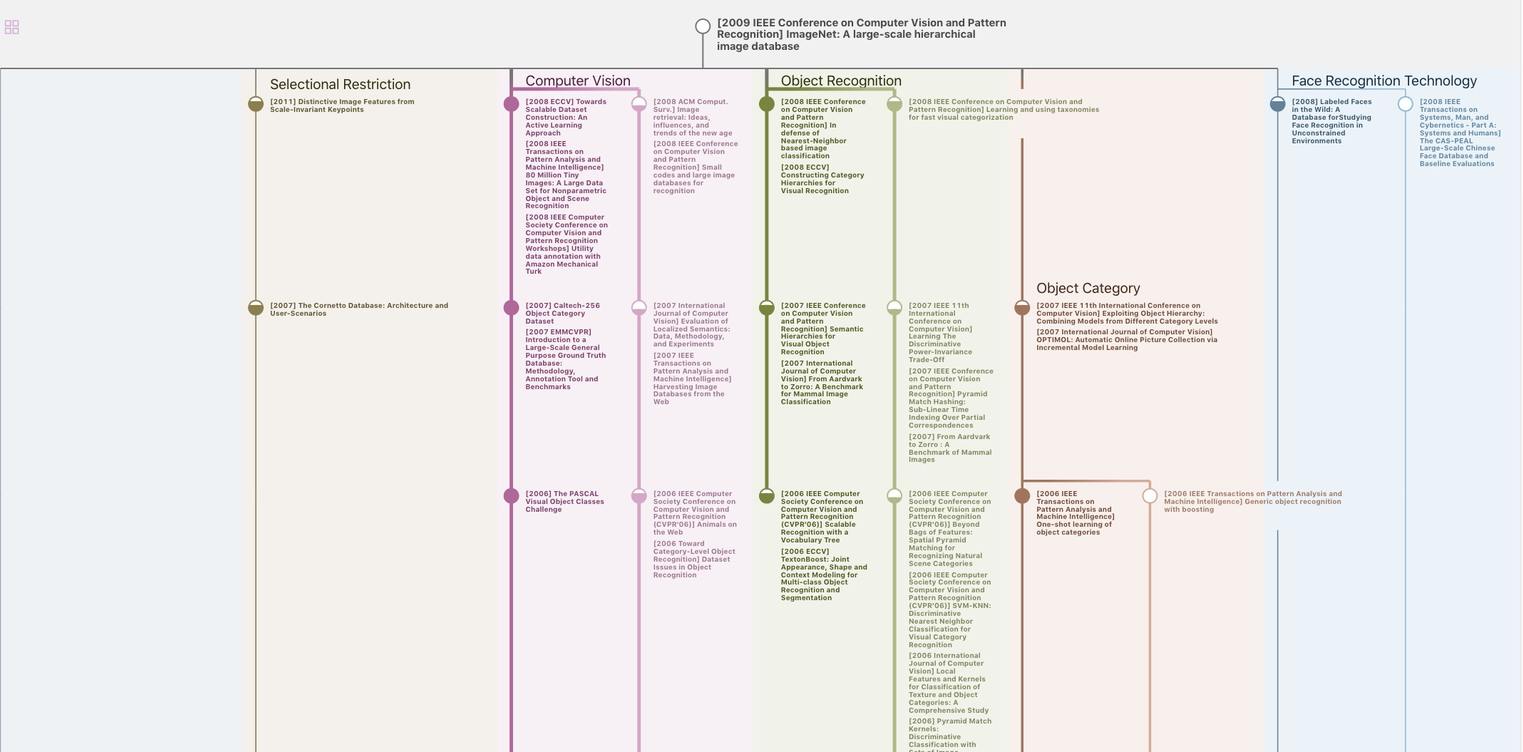
生成溯源树,研究论文发展脉络
Chat Paper
正在生成论文摘要