Active teacher selection for reinforcement learning from human feedback
CoRR(2023)
摘要
Reinforcement learning from human feedback (RLHF) enables machine learning systems to learn objectives from human feedback. A core limitation of these systems is their assumption that all feedback comes from a single human teacher, despite querying a range of distinct teachers. We propose the Hidden Utility Bandit (HUB) framework to model differences in teacher rationality, expertise, and costliness, formalizing the problem of learning from multiple teachers. We develop a variety of solution algorithms and apply them to two real-world domains: paper recommendation systems and COVID-19 vaccine testing. We find that the Active Teacher Selection (ATS) algorithm outperforms baseline algorithms by actively selecting when and which teacher to query. The HUB framework and ATS algorithm demonstrate the importance of leveraging differences between teachers to learn accurate reward models, facilitating future research on active teacher selection for robust reward modeling.
更多查看译文
关键词
active teacher selection,reinforcement learning
AI 理解论文
溯源树
样例
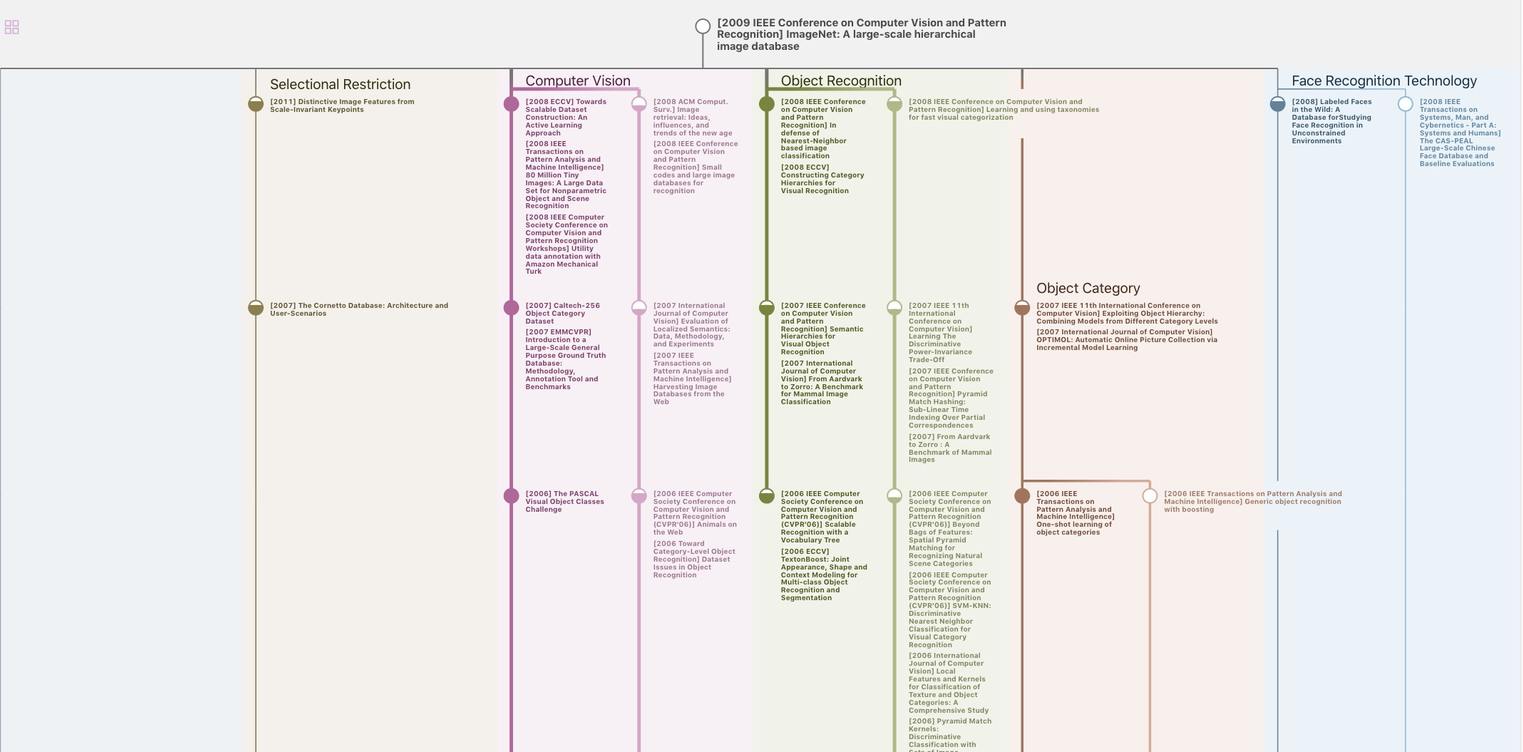
生成溯源树,研究论文发展脉络
Chat Paper
正在生成论文摘要