Learning Fair Representations with High-Confidence Guarantees
CoRR(2023)
摘要
Representation learning is increasingly employed to generate representations that are predictive across multiple downstream tasks. The development of representation learning algorithms that provide strong fairness guarantees is thus important because it can prevent unfairness towards disadvantaged groups for all downstream prediction tasks. To prevent unfairness towards disadvantaged groups in all downstream tasks, it is crucial to provide representation learning algorithms that provide fairness guarantees. In this paper, we formally define the problem of learning representations that are fair with high confidence. We then introduce the Fair Representation learning with high-confidence Guarantees (FRG) framework, which provides high-confidence guarantees for limiting unfairness across all downstream models and tasks, with user-defined upper bounds. After proving that FRG ensures fairness for all downstream models and tasks with high probability, we present empirical evaluations that demonstrate FRG's effectiveness at upper bounding unfairness for multiple downstream models and tasks.
更多查看译文
关键词
representations,learning,high-confidence
AI 理解论文
溯源树
样例
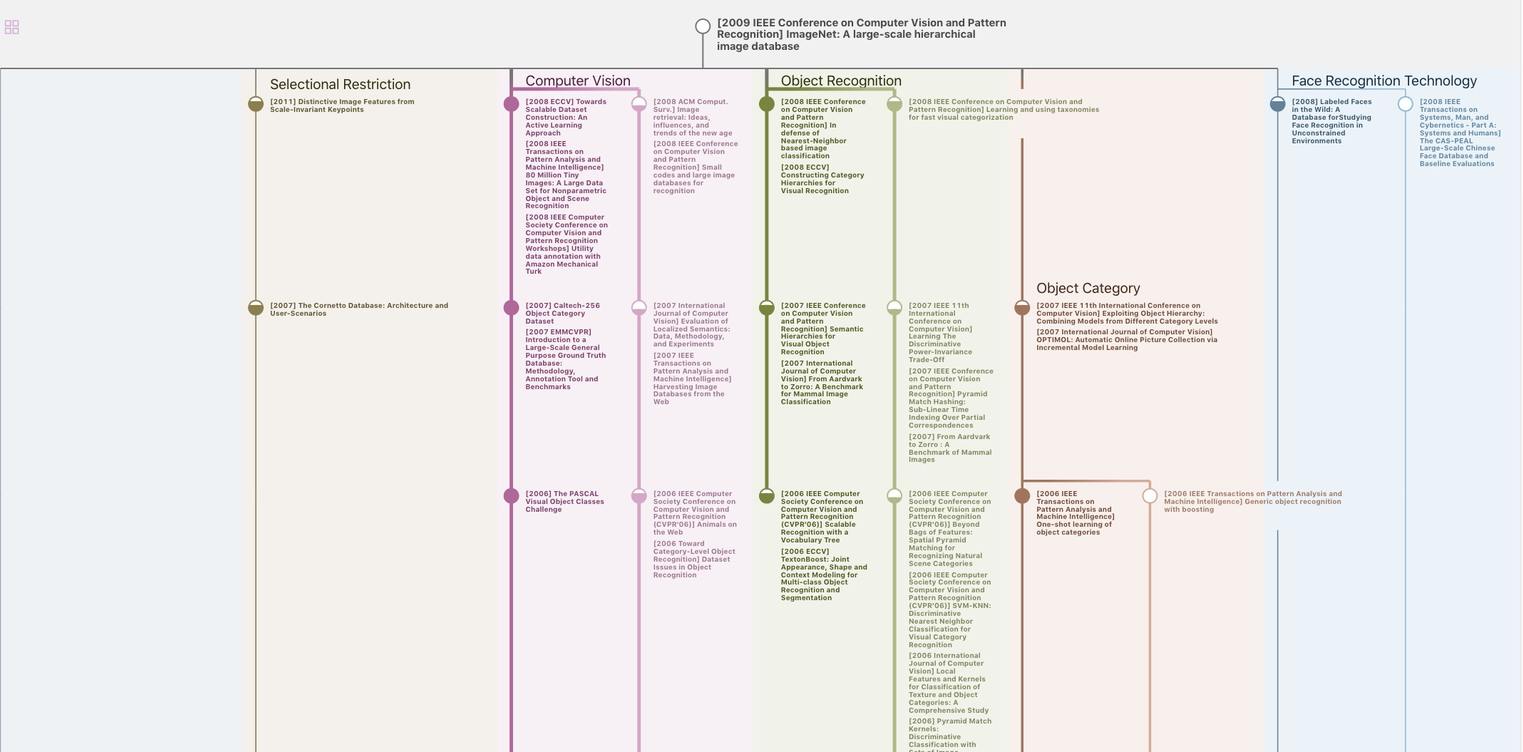
生成溯源树,研究论文发展脉络
Chat Paper
正在生成论文摘要