Fast Propagation is Better: Accelerating Single-Step Adversarial Training via Sampling Subnetworks
IEEE Transactions on Information Forensics and Security(2023)
摘要
Adversarial training has shown promise in building robust models against adversarial examples. A major drawback of adversarial training is the computational overhead introduced by the generation of adversarial examples. To overcome this limitation, adversarial training based on single-step attacks has been explored. Previous work improves the single-step adversarial training from different perspectives, e.g., sample initialization, loss regularization, and training strategy. Almost all of them treat the underlying model as a black box. In this work, we propose to exploit the interior building blocks of the model to improve efficiency. Specifically, we propose to dynamically sample lightweight subnetworks as a surrogate model during training. By doing this, both the forward and backward passes can be accelerated for efficient adversarial training. Besides, we provide theoretical analysis to show the model robustness can be improved by the single-step adversarial training with sampled subnetworks. Furthermore, we propose a novel sampling strategy where the sampling varies from layer to layer and from iteration to iteration. Compared with previous methods, our method not only reduces the training cost but also achieves better model robustness. Evaluations on a series of popular datasets demonstrate the effectiveness of the proposed FB-Better. Our code has been released at https://github.com/jiaxiaojunQAQ/FP-Better.
更多查看译文
关键词
adversarial robustness,single-step attack,adversarial training,model subnetworks,training efficiency
AI 理解论文
溯源树
样例
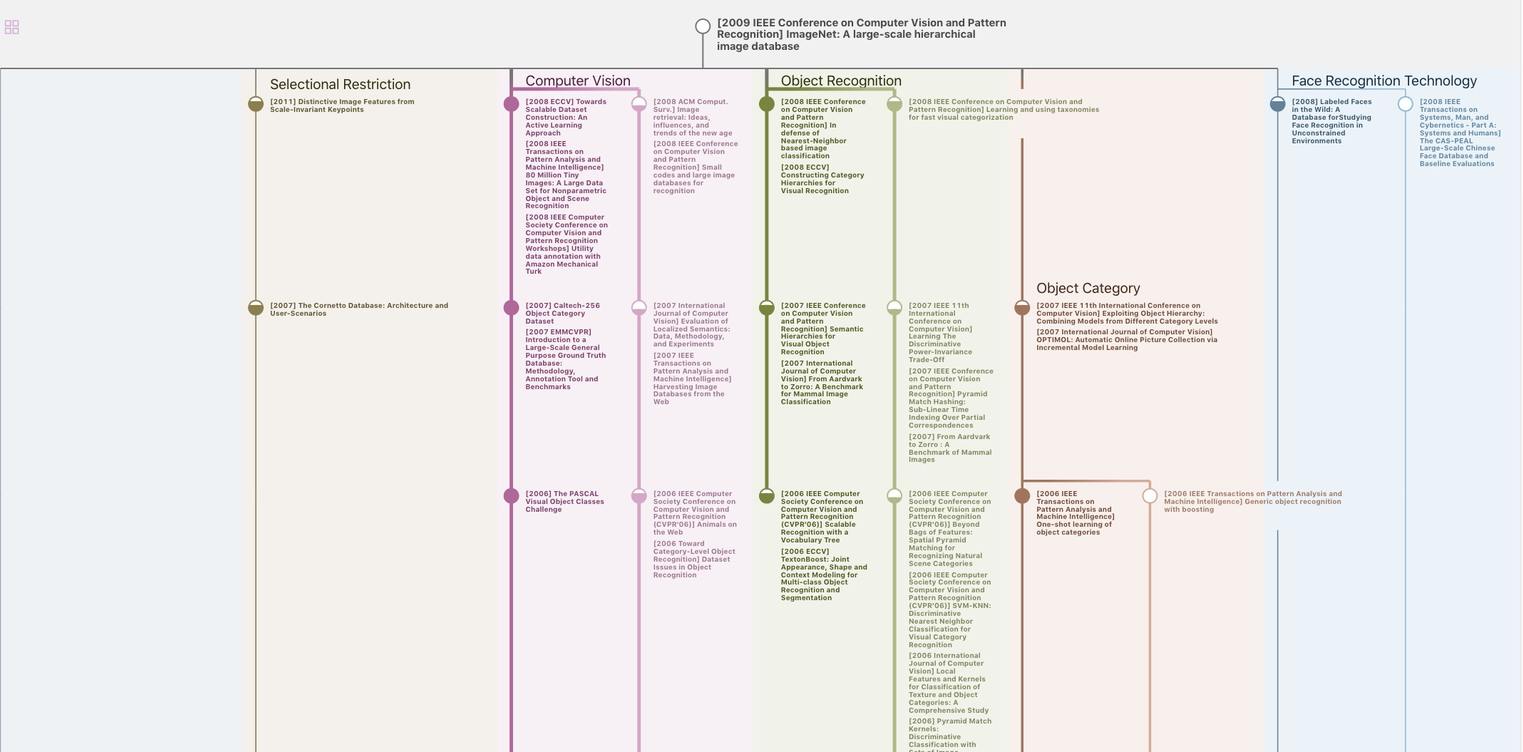
生成溯源树,研究论文发展脉络
Chat Paper
正在生成论文摘要