A statistical significance testing approach for measuring term burstiness with applications to domain-specific terminology extraction
CoRR(2023)
摘要
A term in a corpus is said to be “bursty” (or overdispersed) when its
occurrences are concentrated in few out of many documents. In this paper, we
propose Residual Inverse Collection Frequency (RICF), a statistical
significance test inspired heuristic for quantifying term burstiness. The
chi-squared test is, to our knowledge, the sole test of statistical
significance among existing term burstiness measures. Chi-squared test term
burstiness scores are computed from the collection frequency statistic (i.e.,
the proportion that a specified term constitutes in relation to all terms
within a corpus). However, the document frequency of a term (i.e., the
proportion of documents within a corpus in which a specific term occurs) is
exploited by certain other widely used term burstiness measures. RICF addresses
this shortcoming of the chi-squared test by virtue of its term burstiness
scores systematically incorporating both the collection frequency and document
frequency statistics. We evaluate the RICF measure on a domain-specific
technical terminology extraction task using the GENIA Term corpus benchmark,
which comprises 2,000 annotated biomedical article abstracts. RICF generally
outperformed the chi-squared test in terms of precision at k score with percent
improvements of 0.00
(P@1000), and 1.90
with the performances of other well-established measures of term burstiness.
Based on these findings, we consider our contributions in this paper as a
promising starting point for future exploration in leveraging statistical
significance testing in text analysis.
更多查看译文
AI 理解论文
溯源树
样例
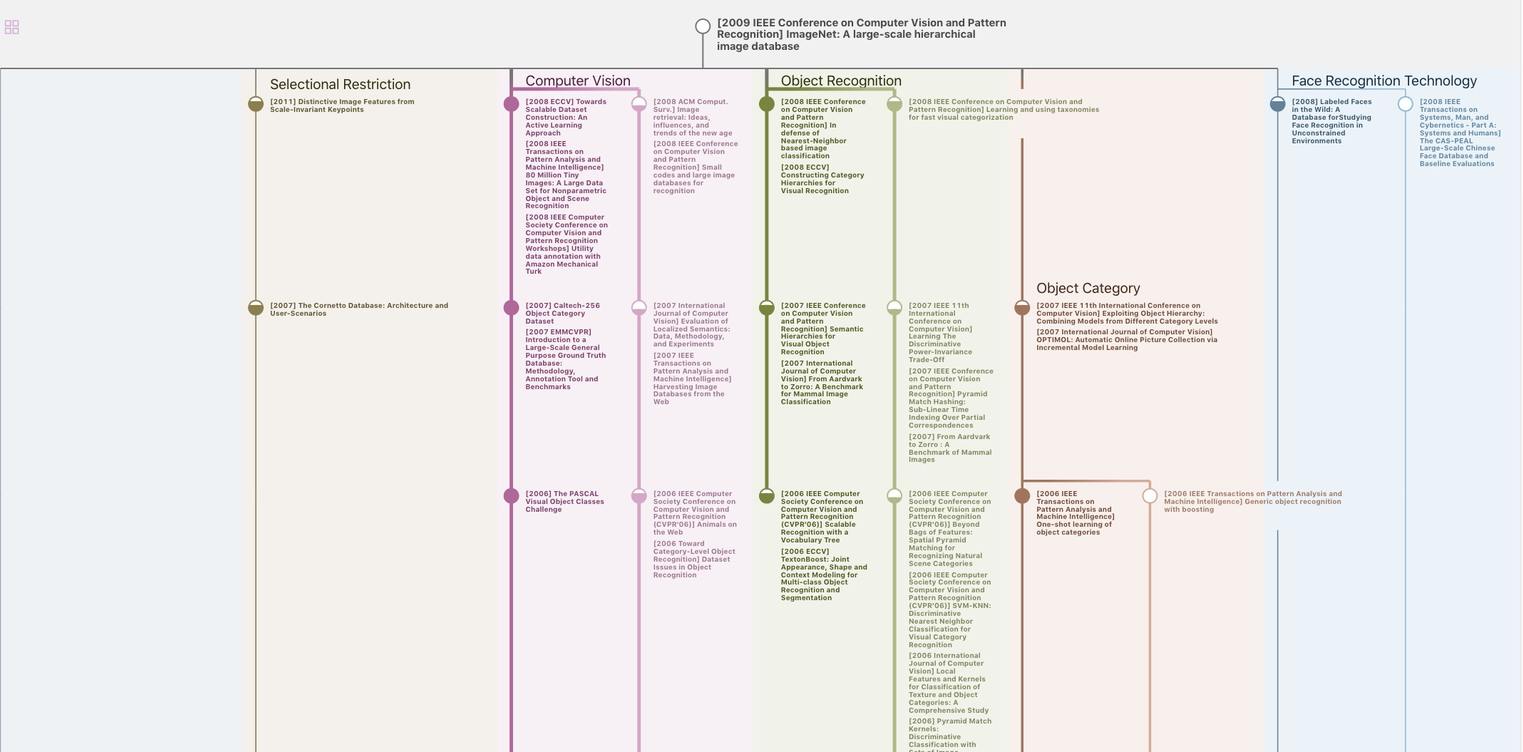
生成溯源树,研究论文发展脉络
Chat Paper
正在生成论文摘要