Reliable Event Generation With Invertible Conditional Normalizing Flow
IEEE TRANSACTIONS ON PATTERN ANALYSIS AND MACHINE INTELLIGENCE(2024)
摘要
Event streams provide a novel paradigm to describe visual scenes by capturing intensity variations above specific thresholds along with various types of noise. Existing event generation methods usually rely on one-way mappings using hand-crafted parameters and noise rates, which may not adequately suit diverse scenarios and event cameras. To address this limitation, we propose a novel approach to learn a bidirectional mapping between the feature space of event streams and their inherent parameters, enabling the generation of reliable event streams with enhanced generalization capabilities. We first randomly generate a vast number of parameters and synthesize massive event streams using an event simulator. Subsequently, an event-based normalizing flow network is proposed to learn the invertible mapping between the representation of a synthetic event stream and its parameters. The invertible mapping is implemented by incorporating an intensity-guided conditional affine simulation mechanism, facilitating better alignment between event features and parameter spaces. Additionally, we impose constraints on event sparsity, edge distribution, and noise distribution through novel event losses, further emphasizing event priors in the bidirectional mapping. Our framework surpasses state-of-the-art methods in video reconstruction, optical flow estimation, and parameter estimation tasks on synthetic and real-world datasets, exhibiting excellent generalization across diverse scenes and cameras.
更多查看译文
关键词
Conditional normalizing flow,contrast threshold,event camera,event generation,event noise rate
AI 理解论文
溯源树
样例
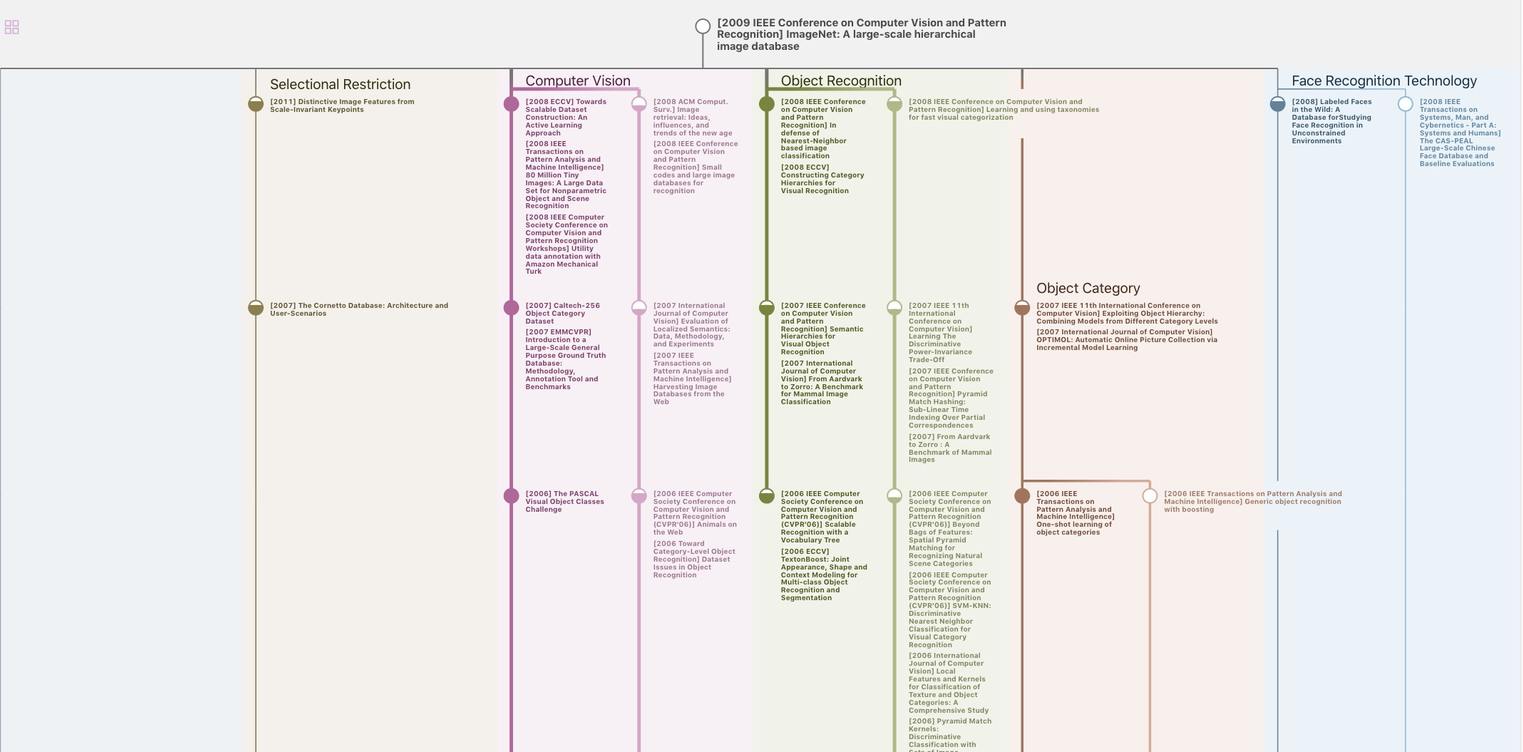
生成溯源树,研究论文发展脉络
Chat Paper
正在生成论文摘要