Machine-learning analysis of cross-study samples according to the gut microbiome in 12 infant cohorts
MSYSTEMS(2023)
摘要
Combining and comparing microbiome data from distinct infant cohorts has been challenging because such data are inherently multidimensional and complex. Here, we used an ensemble of machine-learning (ML) models and studied 16S rRNA amplicon sequencing data from 4,099 gut microbiome samples representing 12 prospectively collected infant cohorts. We chose the childbirth delivery mode as a starting point for such analysis because it has previously been associated with alterations in the gut microbiome in infants. In cross-study ensemble models, Bacteroides was the most important feature in all machine-learning models. The predictive capacity by taxonomy varied with age. At the age of 1-2 months, gut microbiome data were able to predict delivery mode with an area under the curve of 0.72 to 0.83. In contrast, ML models trained on taxa were not able to differentiatedifferentiate between the modes of delivery, in any of the cohorts, when the infants were between 3 and 12 months of age. Moreover, no ML model, alternately trained on the functional pathways of the infant gut microbiome, could consistently predict mode of delivery at any infant age. This study shows that infant gut microbiome data sets can be effectivelyeffectively combined with the application of ML analysis across differentdifferent study populations.
更多查看译文
关键词
machine learning,bioinformatics,human microbiome,gut microbiome,random forest,infant,children,cross-study,ensemble
AI 理解论文
溯源树
样例
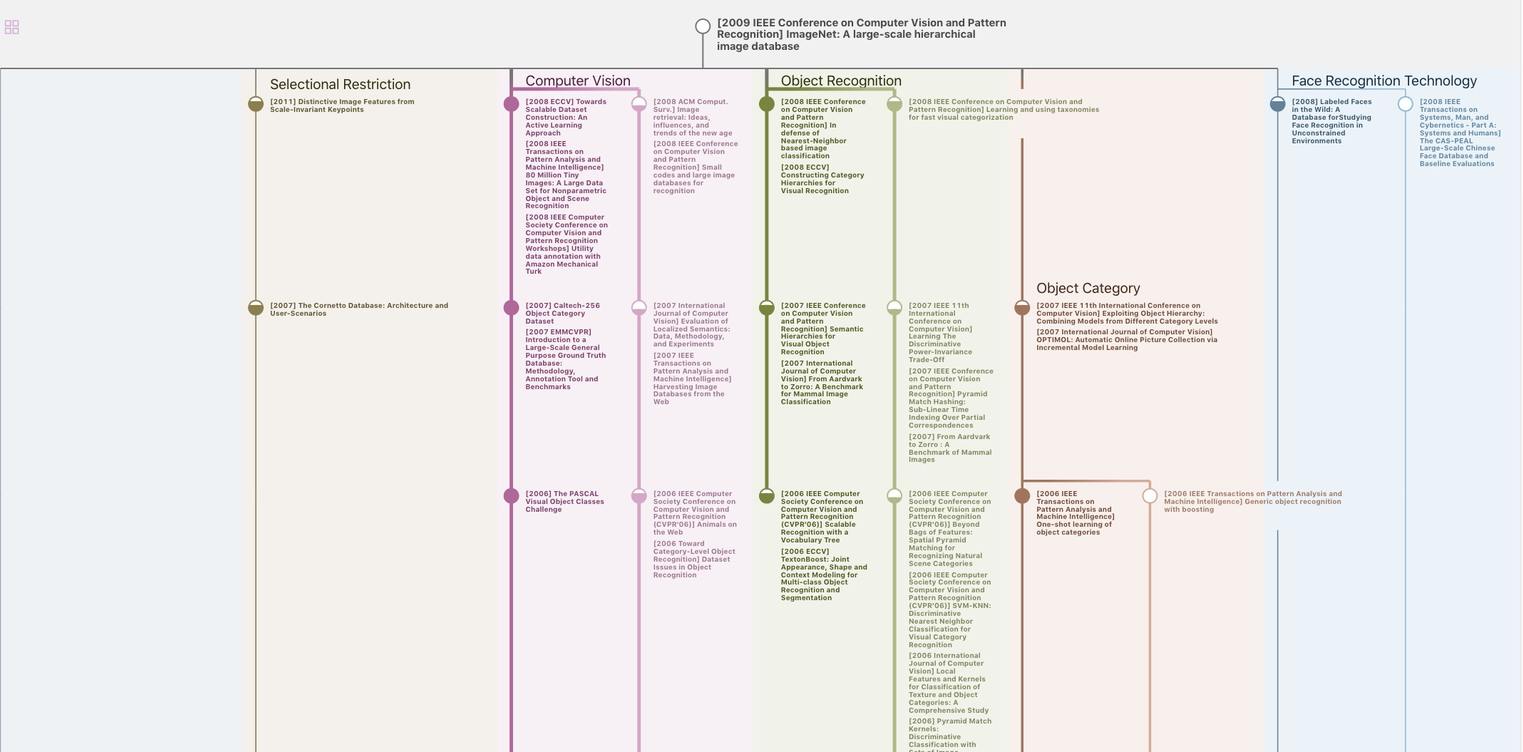
生成溯源树,研究论文发展脉络
Chat Paper
正在生成论文摘要