Unlocking The Mysteries of DNA Adducts with Artificial Intelligence
CHEMBIOCHEM(2024)
摘要
Cellular genome is considered a dynamic blueprint of a cell since it encodes genetic information that gets temporally altered due to various endogenous and exogenous insults. Largely, the extent of genomic dynamicity is controlled by the trade-off between DNA repair processes and the genotoxic potential of the causative agent (genotoxins or potential carcinogens). A subset of genotoxins form DNA adducts by covalently binding to the cellular DNA, triggering structural or functional changes that lead to significant alterations in cellular processes via genetic (e. g., mutations) or non-genetic (e. g., epigenome) routes. Identification, quantification, and characterization of DNA adducts are indispensable for their comprehensive understanding and could expedite the ongoing efforts in predicting carcinogenicity and their mode of action. In this review, we elaborate on using Artificial Intelligence (AI)-based modeling in adducts biology and present multiple computational strategies to gain advancements in decoding DNA adducts. The proposed AI-based strategies encompass predictive modeling for adduct formation via metabolic activation, novel adducts' identification, prediction of biochemical routes for adduct formation, adducts' half-life predictions within biological ecosystems, and, establishing methods to predict the link between adducts chemistry and its location within the genomic DNA. In summary, we discuss some futuristic AI-based approaches in DNA adduct biology. While the experimental approaches are evolving, parallel computational efforts are indispensable to decode DNA adducts. This review accentuates AI's potential in various adduct biology aspects, including novel adduct identification, parent genotoxin associations, formation mechanisms, site of metabolism prediction, and genomic positioning.image
更多查看译文
关键词
carcinogens,electrophiles,machine learning,metabolic enzymes,metabolism
AI 理解论文
溯源树
样例
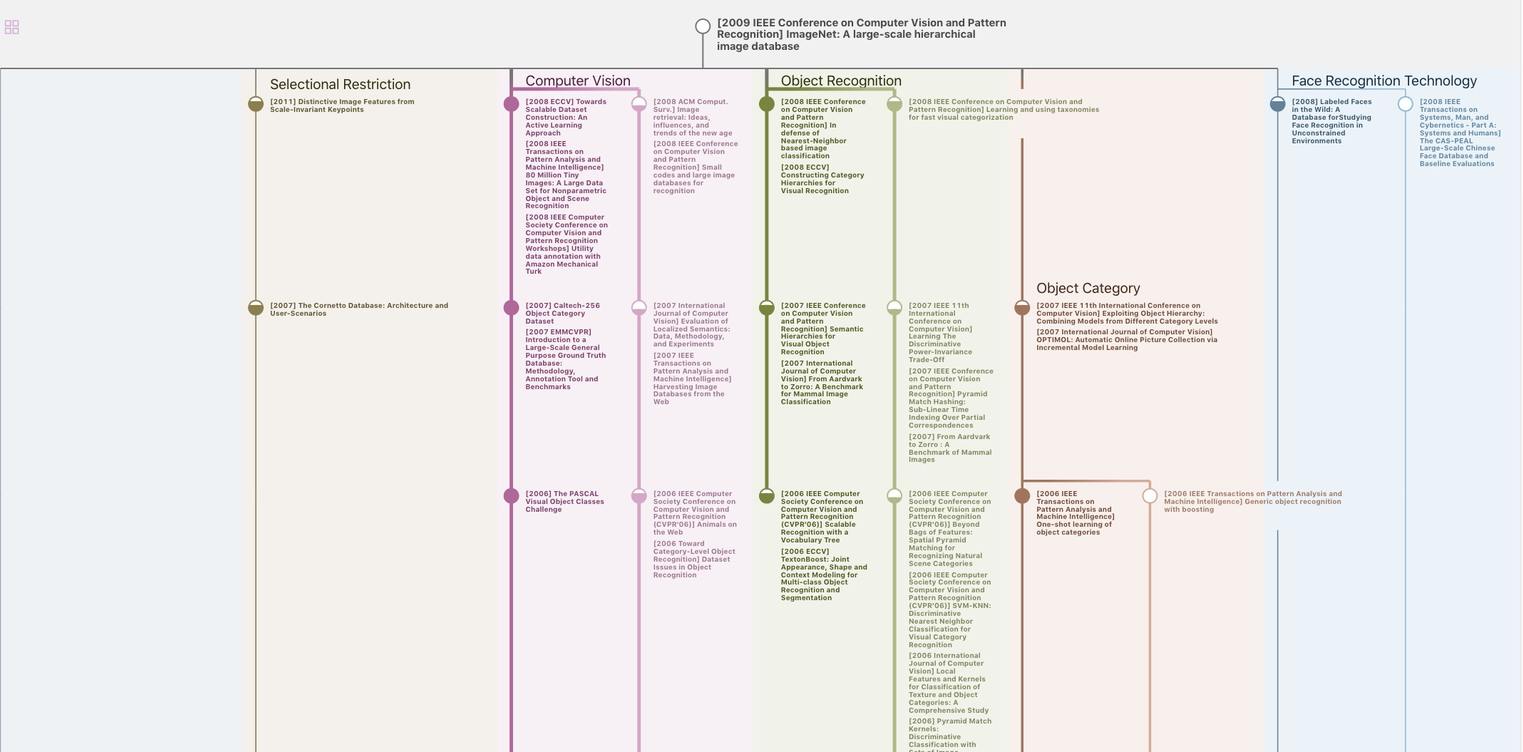
生成溯源树,研究论文发展脉络
Chat Paper
正在生成论文摘要