Privacy-Preserving Mobility-Aware Federated Collaborative Filtering Framework for Caching Prediction in Vehicular Networks
2023 20TH ANNUAL IEEE INTERNATIONAL CONFERENCE ON SENSING, COMMUNICATION, AND NETWORKING, SECON(2023)
摘要
Recommendation algorithm can effectively reduce the difficulty of proactive edge caching prediction by excavating users' preferences among the massive contents, which has drawn great attentions from both academia and industry. The effectiveness of prediction models depends on big data analysis of user information, however, traditional methods based on centralized learning become more and more impractical due to the growing concern on privacy data protection. Recently, implementing the recommendation algorithm in a federated learning (FL) manner has emerged as a promising approach. In an FL manner, users are allowed to keep their private data local and upload the model parameters learned by local training to the server for collaborative training. In this work, we propose a proactive caching prediction algorithm for mobile vehicle users based on differential privacy and federate learning. Our proposed algorithm not only predicts the popular contents with a strong protection for users' private data, but also applies to large-scale networks with massive mobile users. In addition, we also investigate the impact of user mobility on the caching prediction accuracy, and propose an attention-based model aggregation mechanism, which assigns different aggregation weights to each vehicle user and edge server to mitigate the performance degradation caused by user movement. The results show that our proposed model can obtain high caching prediction accuracy and strong privacy protection level in vehicular networks.
更多查看译文
关键词
Caching prediction,recommendation algorithm,federated learning,user mobility,differential privacy
AI 理解论文
溯源树
样例
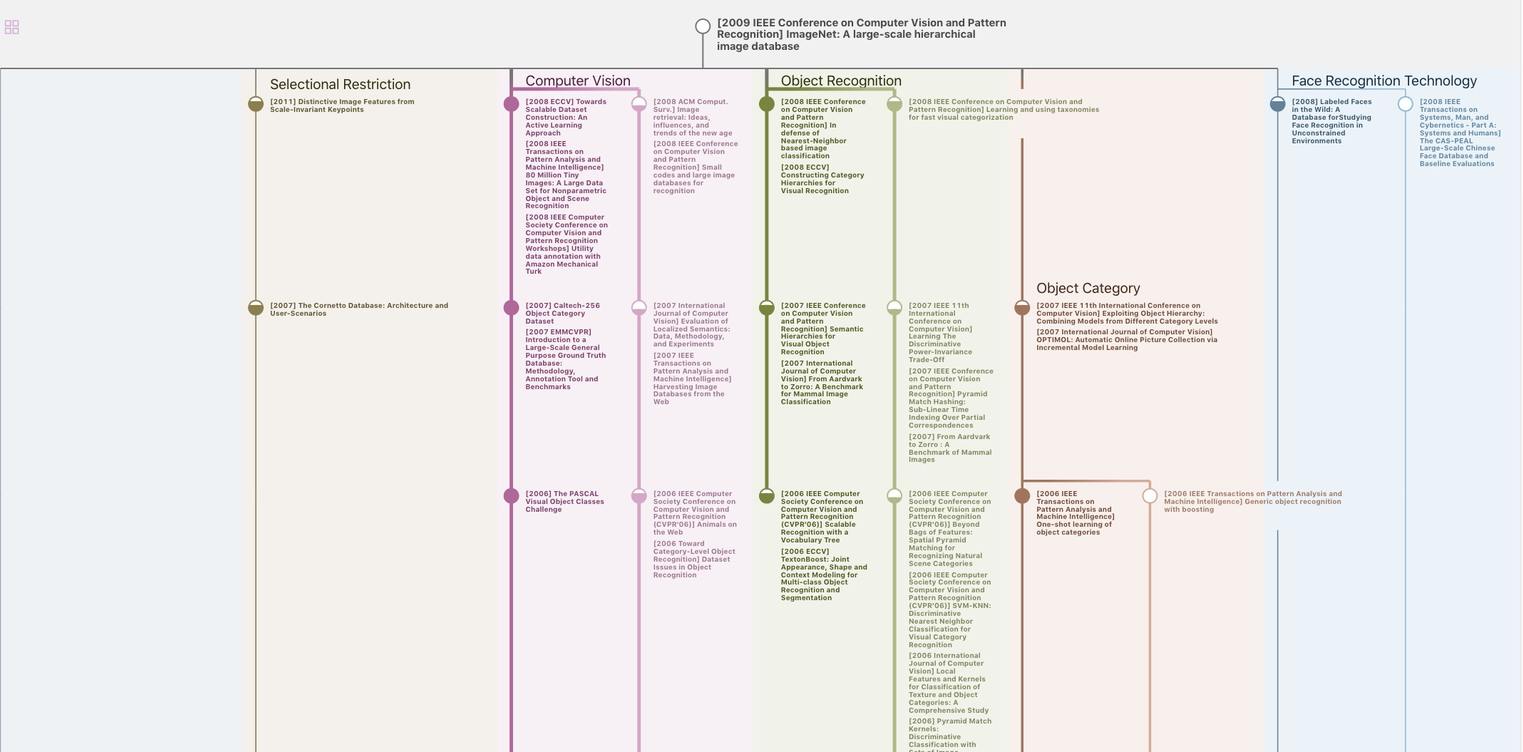
生成溯源树,研究论文发展脉络
Chat Paper
正在生成论文摘要