Graph Tasks Offloading and Resource Allocation in Multi-Access Edge Computing: A DRL-and-Optimization-Aided Approach
IEEE Transactions on Network Science and Engineering(2023)
摘要
Timely and appropriate task offloading is difficult to achieve due to strong coupling between tasks, uncertainty of computing resources and the dynamics of environment. In this article, a multi-access edge computing (MEC) system based on vehicle-to-infrastructure (V2I) communication is considered, where a group of correlated tasks are generated by task initiators (TIs) and then offloaded to different task executors (TEs). This study aims to jointly optimize the graph task assignment and resource allocation in MEC systems to minimize the weighted sum of delay and energy consumption (WDEC). The problem is formulated as a mixed-integer nonlinear programming problem (MINLP). To derive a feasible solution, the optimization process is decomposed into two stages. First, an off-policy algorithm based on deep reinforcement learning (DRL) framework is proposed to solve task offloading problem. Notably, the proposed algorithm relies on neither complete communication status nor prior knowledge of computing resources; Second, given the offloading decisions, the optimal transmission power is obtained by relaxing the convex optimization problem. Extensive experiments verify the superiority of the proposed method, compared with existing algorithms.
更多查看译文
关键词
Multi-access edge computing,deep reinforcement learning,directed acyclic graph,internet-of-vehicles,resource allocation
AI 理解论文
溯源树
样例
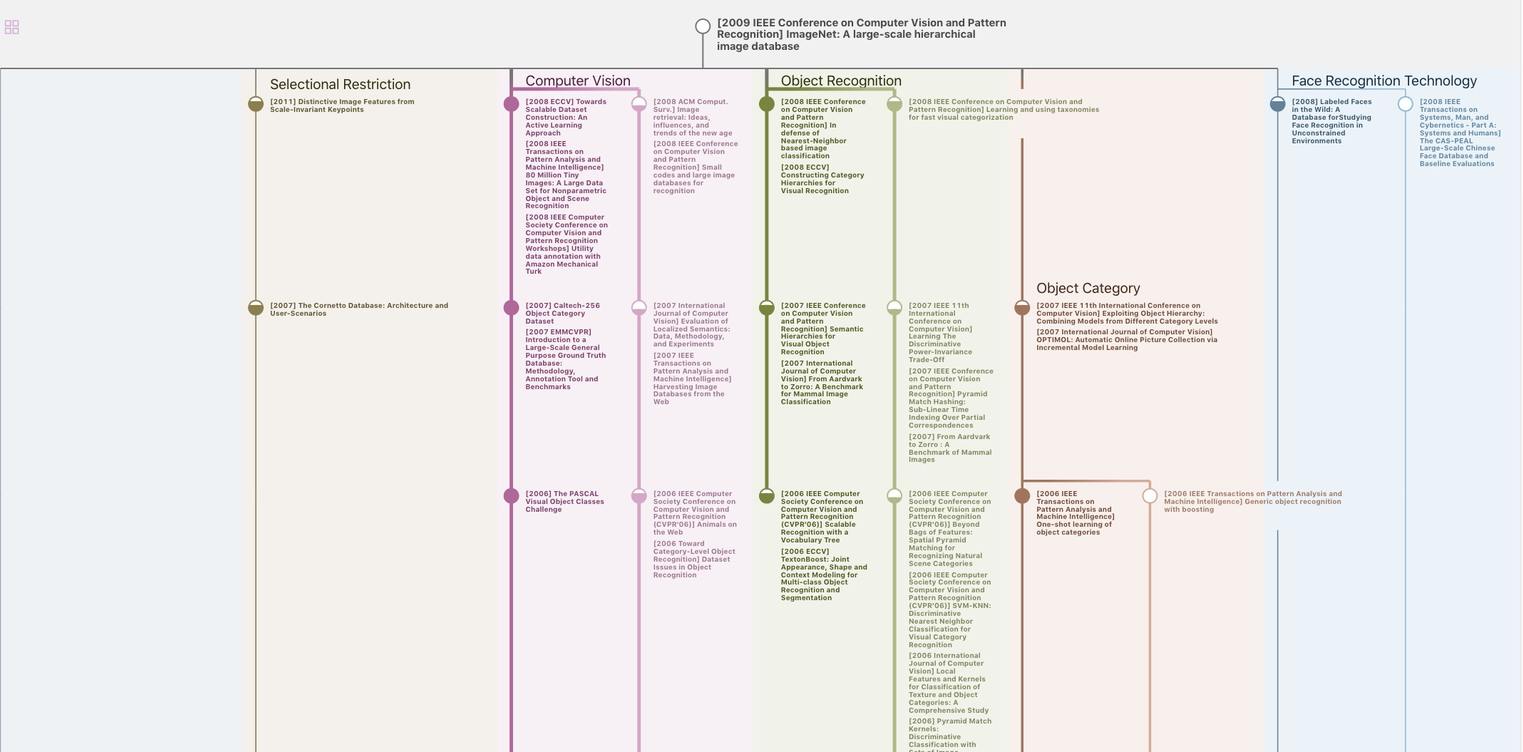
生成溯源树,研究论文发展脉络
Chat Paper
正在生成论文摘要