CloverNet: A Real-Time Network for Semantic Segmentation Onboard Edge Devices Towards Planetary Exploration
2023 9th International Conference on Control, Decision and Information Technologies (CoDIT)(2023)
摘要
This paper introduces CloverNet, a novel convolutional neural network (CNN) designed for real-time semantic segmentation capabilities onboard planetary exploration rovers. Teleoperating planetary rovers is a tedious task as it requires close to real-time monitoring and planning, while distance and communication delay further cripple the efficiency. Future up-scaling of off-world robotic systems deployment makes it increasingly difficult to monitor and command each agent in real-time. Thus, at least an autonomous maneuvering capability is essential to reduce the operational workload at the ground station. In order to achieve this objective, the rover is required to understand its operational environment. The rover then needs to process the information at real-time and efficiently respond in order to complete the allocated tasks or to recover from hazards. An innovative CNN for real-time semantic segmentation is proposed, which can be run on limited memory and graphics processing unit (GPU). The solution is benchmarked on an RGB-D visual sensor and on an NVIDIA Jetson AGX Xavier mounted on a skid-steering micro-rover platform. CloverNet consists of a light encoder-decoder architecture that is optimized and quantized using the NVIDIA TensorRT framework, enabling it to operate at high frame rate and low GPU latency. The network is trained using a new data set curated for developing semantic segmentation of rocks and rovers belonging to Lunar and Martian analogue environments. Experimental results showed it can reach high performance in terms of accuracy and intersection over the union, as well as achieving a maximum inference speed of ∼ 55 FPS on a GPU board at the edge. As such, the results prove CloverNet suitable for planetary missions.
更多查看译文
AI 理解论文
溯源树
样例
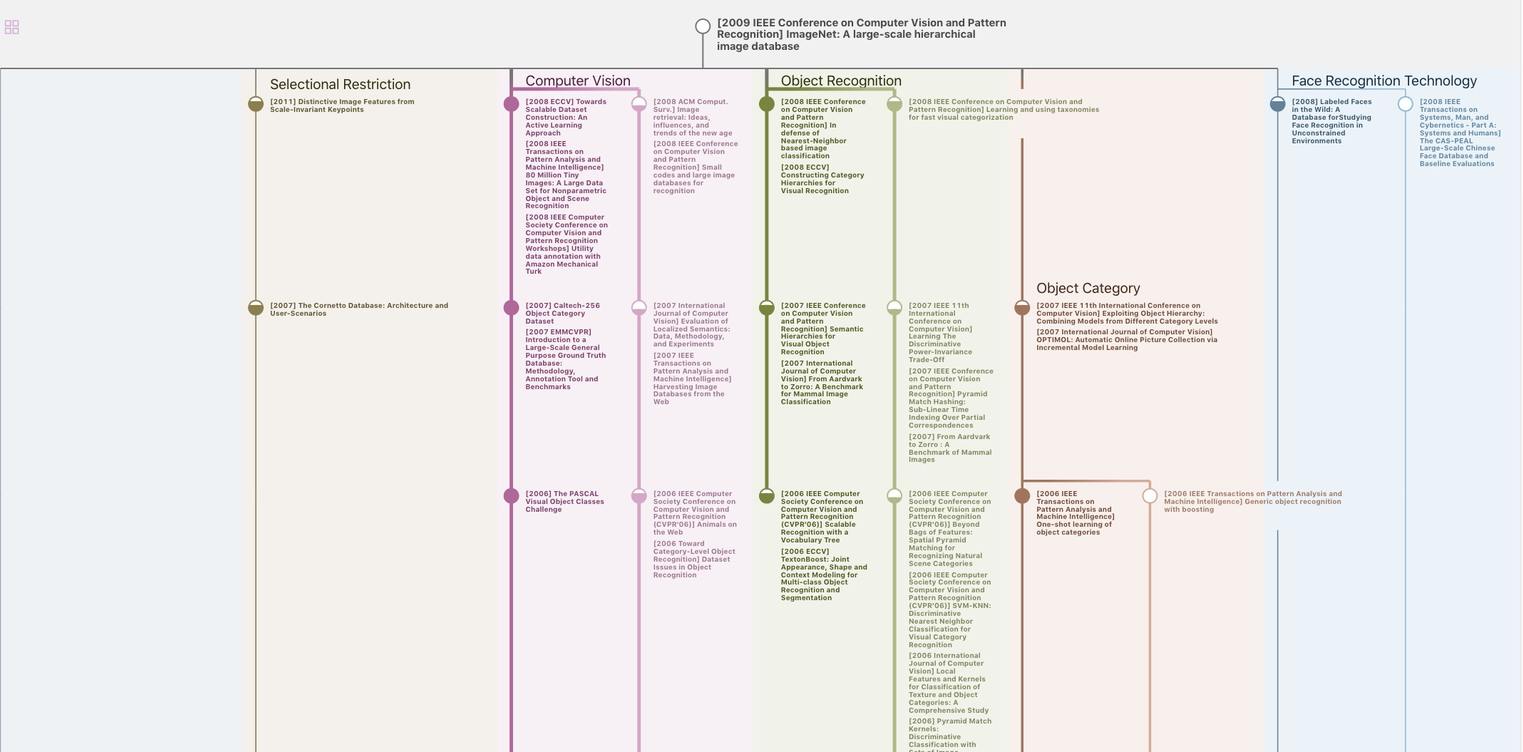
生成溯源树,研究论文发展脉络
Chat Paper
正在生成论文摘要