Poison is Not Traceless: Fully-Agnostic Detection of Poisoning Attacks
CoRR(2023)
摘要
The performance of machine learning models depends on the quality of the underlying data. Malicious actors can attack the model by poisoning the training data. Current detectors are tied to either specific data types, models, or attacks, and therefore have limited applicability in real-world scenarios. This paper presents a novel fully-agnostic framework, DIVA (Detecting InVisible Attacks), that detects attacks solely relying on analyzing the potentially poisoned data set. DIVA is based on the idea that poisoning attacks can be detected by comparing the classifier's accuracy on poisoned and clean data and pre-trains a meta-learner using Complexity Measures to estimate the otherwise unknown accuracy on a hypothetical clean dataset. The framework applies to generic poisoning attacks. For evaluation purposes, in this paper, we test DIVA on label-flipping attacks.
更多查看译文
关键词
traceless,attacks,detection,fully-agnostic
AI 理解论文
溯源树
样例
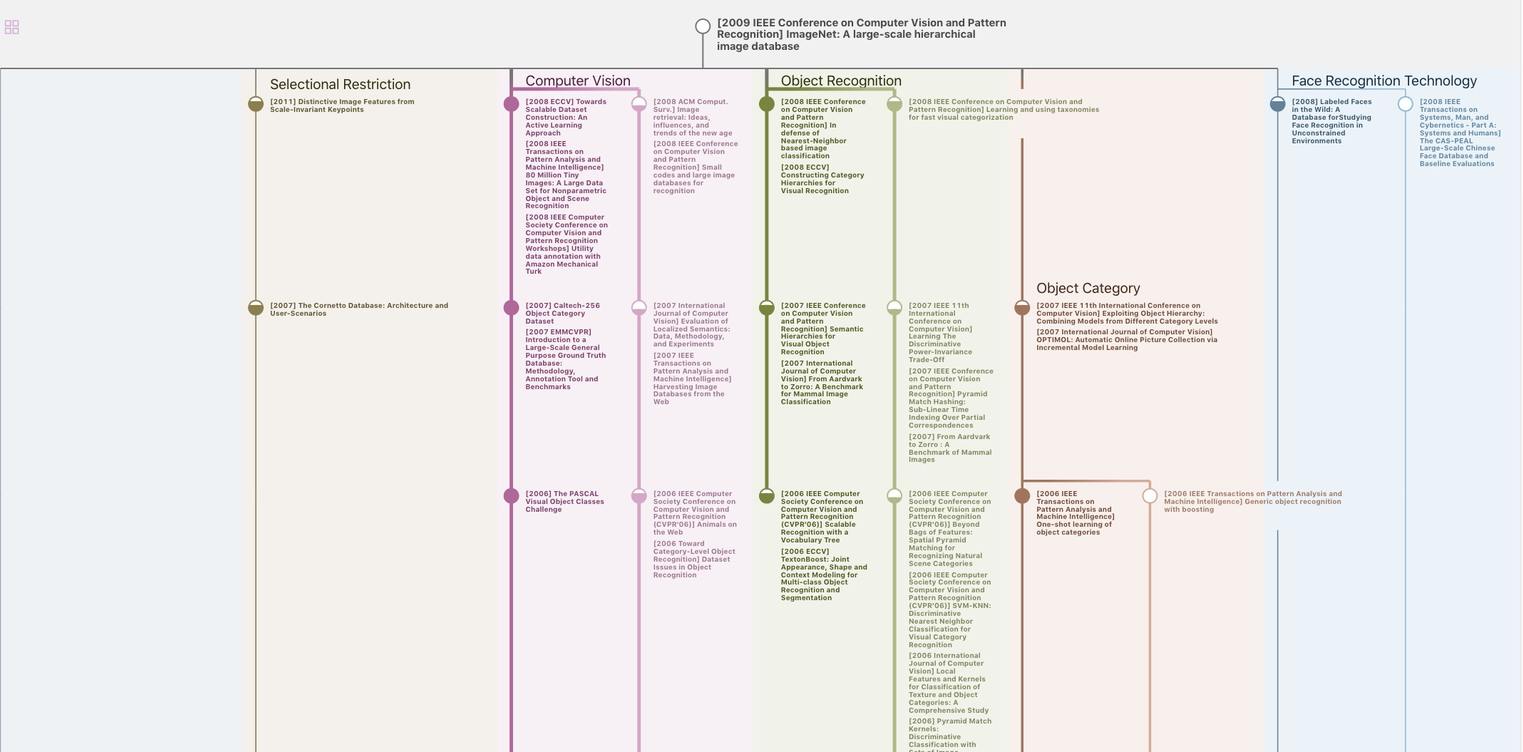
生成溯源树,研究论文发展脉络
Chat Paper
正在生成论文摘要