Neural Potential Field for Obstacle-Aware Local Motion Planning
ICRA 2024(2024)
摘要
Model predictive control (MPC) may provide local motion planning for mobile robotic platforms. The challenging aspect is the analytic representation of collision cost for the case when both the obstacle map and robot footprint are arbitrary. We propose a Neural Potential Field: a neural network model that returns a differentiable collision cost based on robot pose, obstacle map, and robot footprint. The differentiability of our model allows its usage within the MPC solver. It is computationally hard to solve problems with a very high number of parameters. Therefore, our architecture includes neural image encoders, which transform obstacle maps and robot footprints into embeddings, which reduce problem dimensionality by two orders of magnitude. The reference data for network training are generated based on algorithmic calculation of a signed distance function. Comparative experiments showed that the proposed approach is comparable with existing local planners: it provides trajectories with outperforming smoothness, comparable path length, and safe distance from obstacles.
更多查看译文
关键词
Collision Avoidance,Machine Learning for Robot Control,Motion and Path Planning
AI 理解论文
溯源树
样例
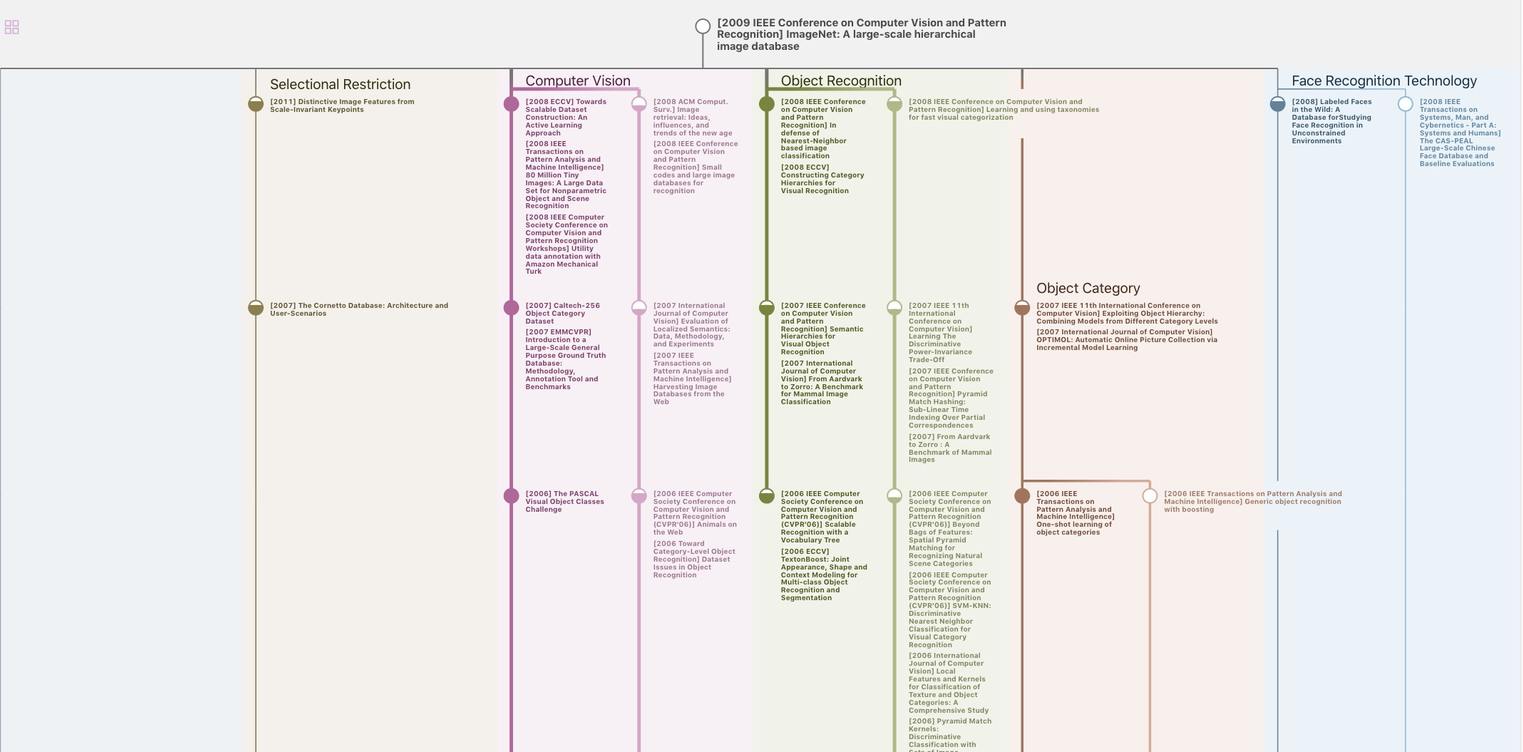
生成溯源树,研究论文发展脉络
Chat Paper
正在生成论文摘要