Personalized agricultural knowledge services: a framework for privacy-protected user portraits and efficient recommendation
JOURNAL OF SUPERCOMPUTING(2023)
摘要
In recent years, the increasing demand for knowledge services and the challenges of information overload have posed significant problems in delivering personalized and efficient agricultural knowledge services. This paper presents a comprehensive framework that addresses the issues of vague user positioning, serious privacy leakage, and low efficiency in personalized knowledge services within the national agricultural knowledge intelligent service cloud platform. The proposed framework utilizes privacy-protected user portraits based on generative adversarial nets (GAN) and leverages the TextCNN-LSTM algorithm for agricultural knowledge service prediction. By embedding labels into the algorithm and employing data obfuscation techniques, the framework achieves accurate inference of user behavior while preserving user privacy. Experimental results demonstrate the effectiveness and accuracy of the proposed framework, highlighting its potential for regional precise positioning and recommendation of personalized agricultural knowledge services. Experimental data shows that the average absolute error and root-mean-square error of this method are 1.1997 and 1.4143, respectively, and compared with MLP, TextCNN, and LSTM models, and it has higher prediction accuracy. In recent years, the increasing demand for knowledge services and the challenges of information overload have posed significant problems in delivering personalized and efficient agricultural knowledge services.
更多查看译文
关键词
User portrait,Privacy protection,Knowledge service,Intelligent recommendation,Service matching
AI 理解论文
溯源树
样例
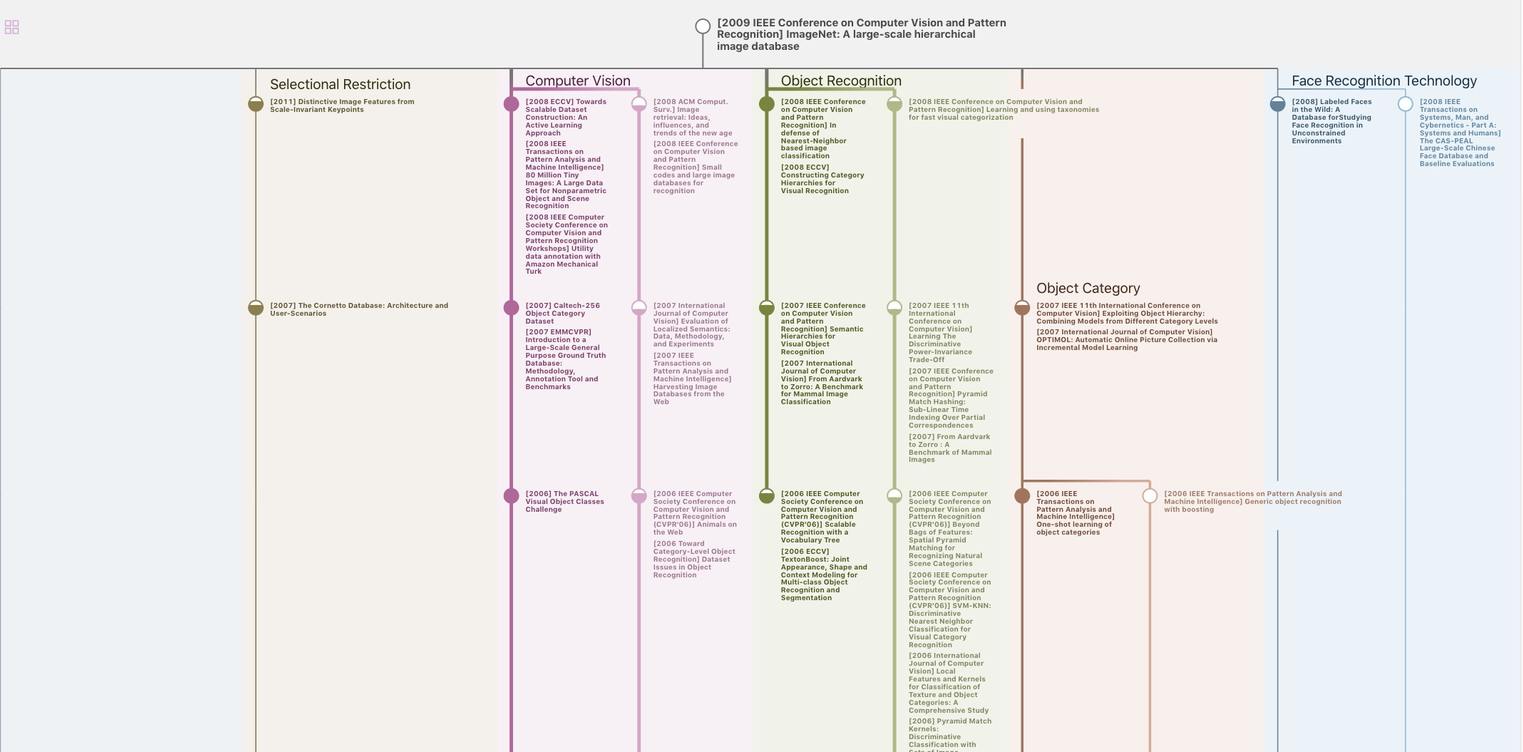
生成溯源树,研究论文发展脉络
Chat Paper
正在生成论文摘要