Feature Selection Based on Fuzzy Combination Entropy Considering Global and Local Feature Correlation
Information sciences(2024)
摘要
Feature selection is a commonly employed method to decrease data processing complexity by discarding unnecessary and repetitive features. An effective feature selection method can mitigate the challenges posed by high-dimensional data, save computing resources and improve learning performance. Combination entropy is a useful tool for assessing feature uncertainty, which provides an intuitive representation of the amount of information. However, classical combination entropy is difficult to be directly used for continuous features. Therefore, we propose the concept of fuzzy combination entropy. Moreover, we put forward an importance metric that comprehensively considers global feature correlation and local feature correlation. Firstly, the fuzzy combination entropy (FCE) is presented based on the fuzzy λ -similarity relation. Secondly, by combining the benefits of fuzzy rough sets and combination entropy, fuzzy combination entropy and its variants are constructed, and their related properties are also discussed. Thirdly, the concepts of global feature correlation and local feature correlation are defined and an importance metric is proposed. Finally, a feature selection method according to fuzzy combination entropy considering global feature correlation and local feature correlation (FSmFCE) is designed. According to the findings from our experiments, it is evident that our algorithm demonstrates a preference for selecting a smaller feature set, yet still achieves commendable classification performance.
更多查看译文
关键词
Feature selection,Mutual information,Fuzzy rough sets,Combination entropy
AI 理解论文
溯源树
样例
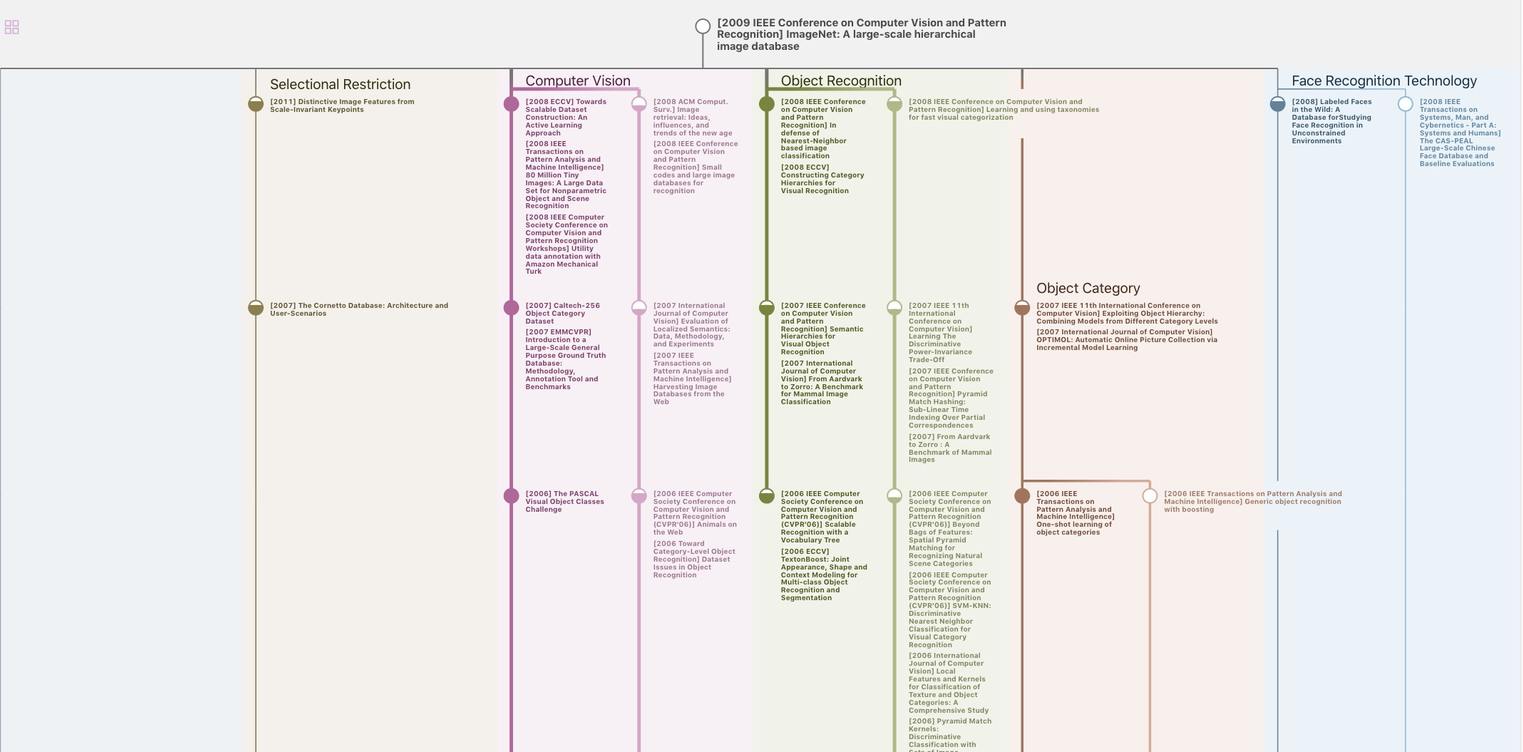
生成溯源树,研究论文发展脉络
Chat Paper
正在生成论文摘要