Weak relationship indicator-based evolutionary algorithm for multimodal multi-objective optimization
INFORMATION SCIENCES(2024)
摘要
Multimodal multi-objective problems (MMOPs) have multiple equivalent Pareto sets (PSs) that map to the same Pareto optimal front (PF). Traditional multimodal multiobjective algorithms (MMEAs) use strong relationships to guide population convergence, but this can lead to two problems: the population may explore easier-to-search PSs and lose more difficult-to-search PSs, and it may not retain local PSs well. To address these issues, we propose a weak relationship indicator-based MMEA that includes weak convergence indicators and density evaluation indicators. The weak convergence indicator considers the relationship between an individual and its neighbors, while the density evaluation indicator considers the density information of the individual and its neighbors. This allows the population to retain solutions from different PSs during exploration. An archive based on weak convergence indicators also retains excellent solutions generated during the evolution of the population. Experimental results show that our algorithm ranked first in terms of overall score when compared with seven stateof-the-art algorithms using the Friedman Test.
更多查看译文
关键词
Evolutionary algorithms,Multimodal,Multi-objective optimization,Weak relationship indicators
AI 理解论文
溯源树
样例
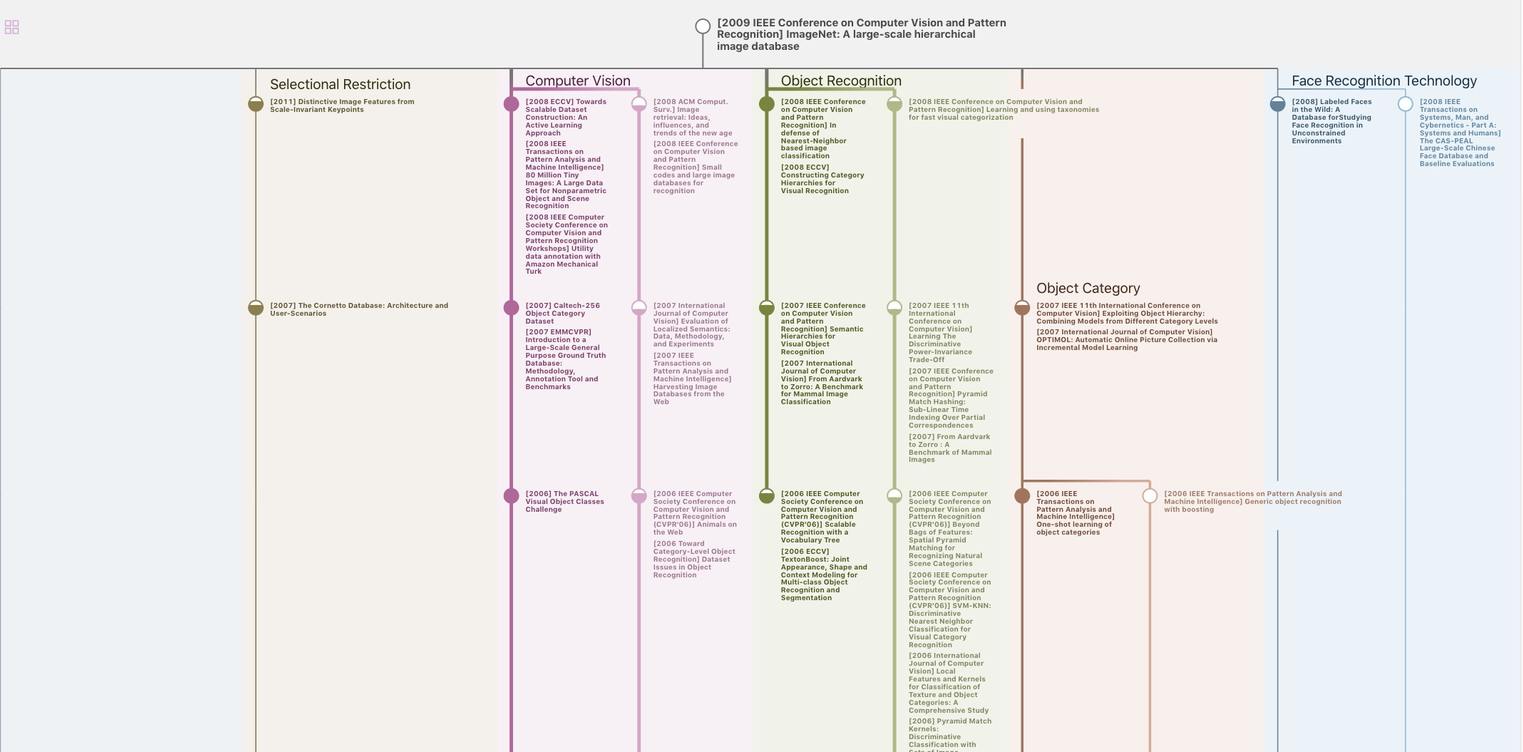
生成溯源树,研究论文发展脉络
Chat Paper
正在生成论文摘要