Automatic spacing inspection of rebar spacers on reinforcement skeletons using vision-based deep learning and computational geometry
Journal of Building Engineering(2023)
摘要
Improper spacing of rebar spacers (RSs) in reinforced concrete structures can lead to corrosion and damage of rebars, ultimately decreasing the strength and durability of the structure. The current method for RSs spacing inspection entails a manual inspection that utilizes a measuring tape, which is both labor-intensive and time-consuming. This paper presents a novel method for automatically inspecting RSs spacing using a vision-based deep learning and computational geometry. The proposed method consists of three modules including the RSs location and classification module, the keypoints detection module and RSs spacing inspection module. The RSs location and classification module employ object detection of faster-region convolutional neural network (Faster-RCNN)-based deep learning to accurately locate and classify RSs. The keypoints detection module uses human pose estimation of cascaded pyramid network (CPN)-based deep learning to detect the three keypoints necessary for accurate RSs spacing calculations. In RSs spacing inspection module, the computational geometry is applied to derive an accurate expression for calculating RSs spacing in the transverse and longitudinal directions, based on the RSs keypoints coordinates and camera parameters. The RSs spacing is subsequently evaluated to check its compliance. In the experiment, the algorithms of the three modules were integrated into a robot that can automatically move and take pictures to accomplish the task of automatically inspecting RSs spacing in a large reinforced concrete component plant. The experimental results indicate that this method yields an average RSs spacing measurement error of 1.35 cm. Finally, the robustness of the proposed method was validated by changing the conditions of image acquisition processes.
更多查看译文
关键词
Rebar spacer, Automatic spacing inspection, Computational geometry, Deep learning, Object detection, Human pose estimation
AI 理解论文
溯源树
样例
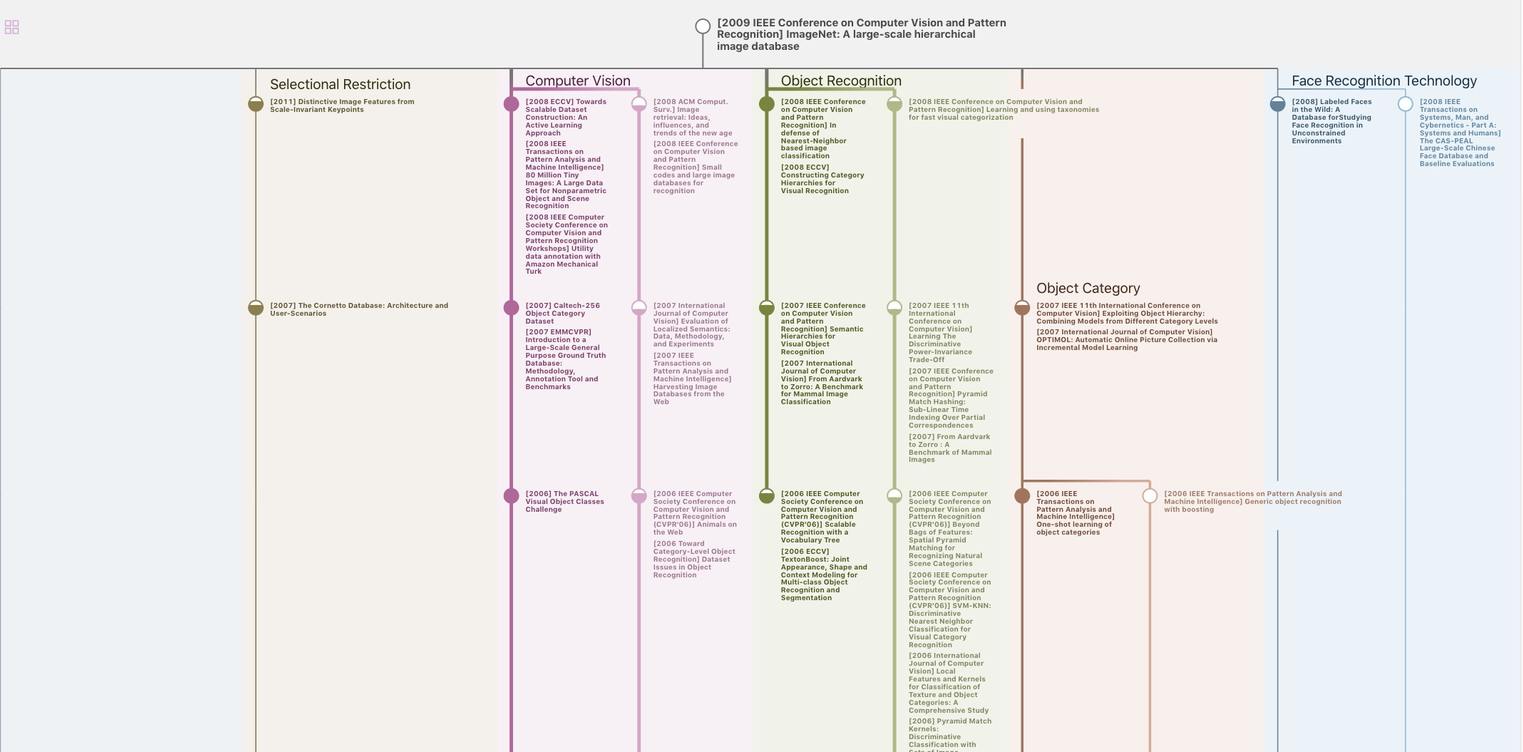
生成溯源树,研究论文发展脉络
Chat Paper
正在生成论文摘要