An attention-based multi-scale convolution network for intelligent underwater acoustic signal recognition
Ocean Engineering(2023)
摘要
Underwater acoustic signal identification is always an extremely challenging task due to the intricate marine environments and background noise interference. Existing techniques typically focus on handling only one type of specific features for recognition, overlooking a comprehensive representation of distinct vessel attributes and struggling to handle intricate scenarios. To address these challenges, we employ a band-pass filter bank for feature extraction. This process extracts multiple representations of the same data, each concentrating on distinct frequency components. Subsequently, the filtered multi-view representation is channeled into an attention-based multi-scale convolution network (AMSC-Net) for recognition purposes. AMSC-Net integrates a sequence of attention-based multi-scale convolution (AMSC) blocks to capture pivotal features tied to identifying diverse ship-radiated audio categories. Specifically, a set of parallel residual convolution layers with different kernels are designed to capture multi-scale features, and an adaptive channel attention module is followed to highlight dominant parts within global features. To enrich training data in terms of quantity and diversity, a data augmentation strategy is implemented during the training phase. Our proposals are evaluated on two real-world underwater acoustic signal datasets. Experimental results firmly establish the superiority of our proposed AMSC-Net, which substantially outperforms competitive DNN-based methods. Moreover, our proposals exhibit robustness across different noise levels, achieving an overall accuracy of 95.87% and 95.39% for the two datasets at 0 dB signal-to-noise ratio (SNR), respectively.
更多查看译文
关键词
Multi-view representation,Channel attention,Multi-scale convolution,Underwater acoustic classification
AI 理解论文
溯源树
样例
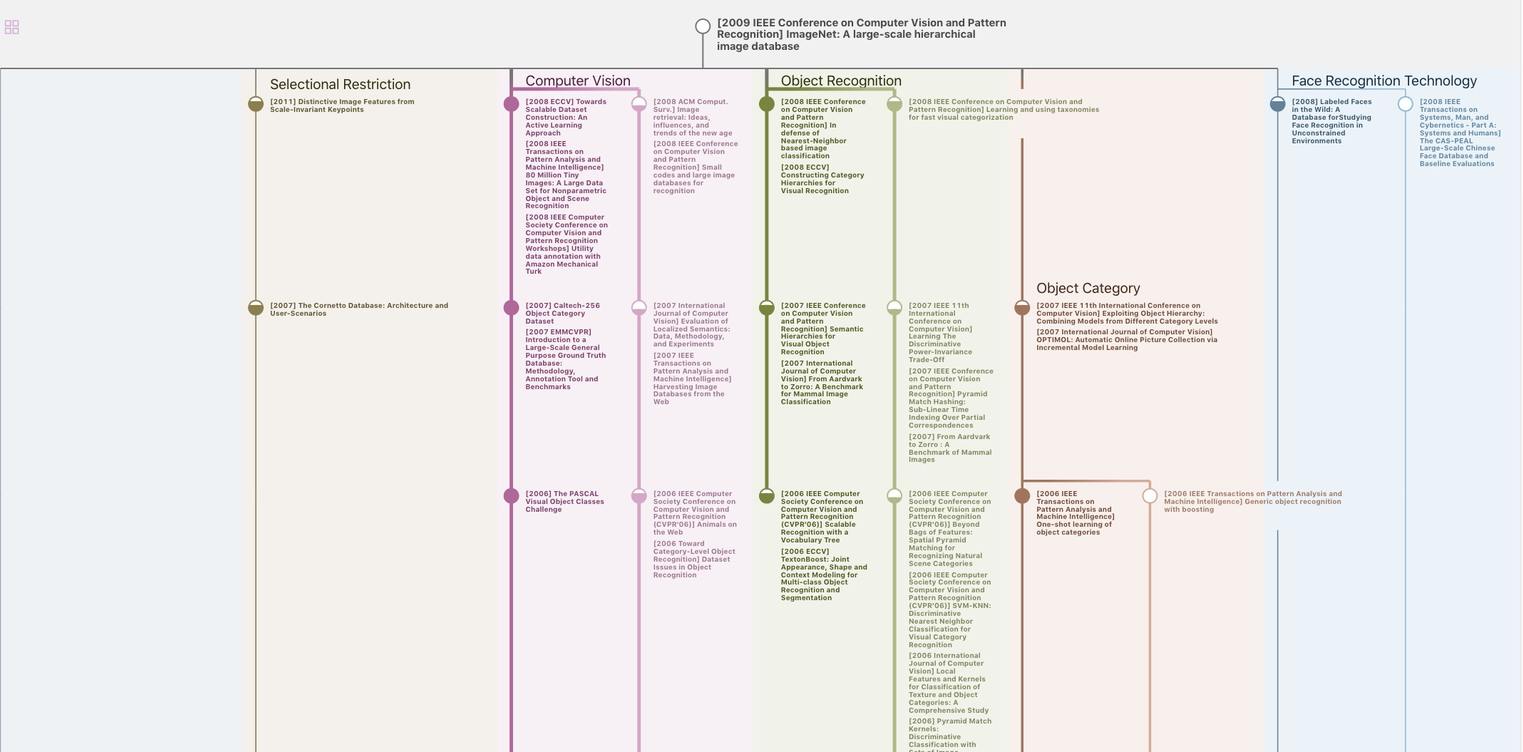
生成溯源树,研究论文发展脉络
Chat Paper
正在生成论文摘要