A Trust-Region-like Algorithm for Expensive Multi-Objective Optimization
Applied soft computing(2023)
摘要
In solving expensive multi-objective optimization problems, surrogate models have been widely investigated. The existing multi-objective algorithms adopting surrogate models can be classified into two categories: surrogate-assisted evolutionary algorithms (SAEAs) and surrogate-based optimization algorithms (SBAs). However, their efficiency and convergence remain considerably inadequate. In this work, we propose a trust-region-like algorithm for dealing with expensive multi-objective problems, where only a small number of expensive objective function evaluations are allowed. The optimization process of the proposed algorithm mainly includes two stages. The first stage is the surrogate-assisted stage, in which a promising population with the consideration of convergence and diversity is obtained by reference vector-guided evolutionary algorithms (RVEA). The promising population is considered the preliminary trust-region. Moreover, a new adaptive sampling selection criterion switching between two different sampling strategies is used to further narrow the trust-region. The second stage is the surrogate-based stage, wherein the selection of the most promising individuals is carried out from the ultimate trust-region using the pseudo hypervolume-based expected improvement matrix (PEIM) criterion. Comparison results on the benchmark functions demonstrate that the proposed algorithm is competitive with five state-of-art algorithms in a limited computational budget. Finally, the proposed algorithm is used in the design optimization of a variable stiffness composite cylinder.
更多查看译文
关键词
Expensive multi-objective optimization,Surrogate models,Sampling strategies,Trust -region,Reference vectors
AI 理解论文
溯源树
样例
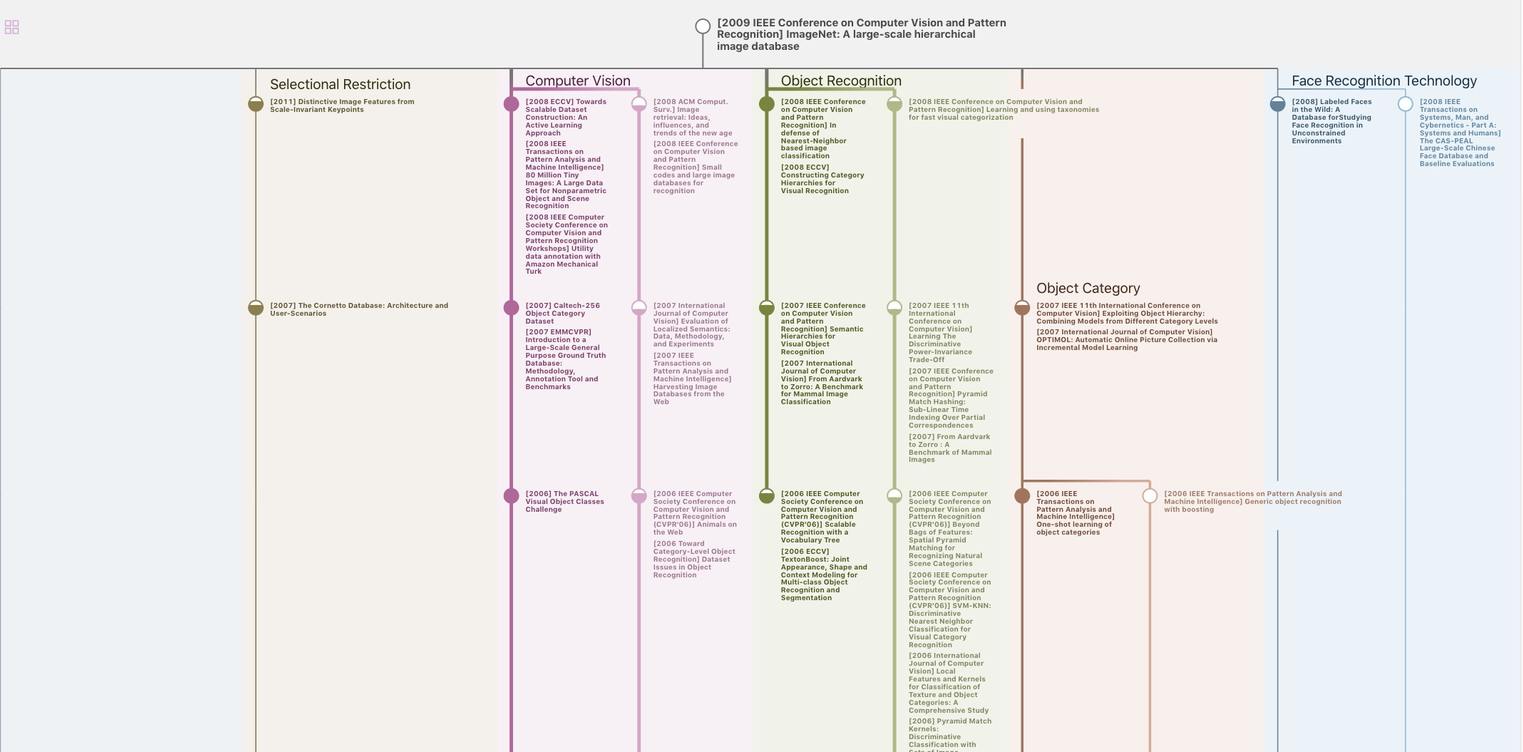
生成溯源树,研究论文发展脉络
Chat Paper
正在生成论文摘要