Performance-Based Generative Design for Parametric Modeling of Engineering Structures Using Deep Conditional Generative Models
AUTOMATION IN CONSTRUCTION(2023)
摘要
Parametric Modeling, Generative Design, and Performance-Based Design have gained increasing attention in the AEC field as a way to create a wide range of design variants while focusing on performance attributes rather than building codes. However, the relationships between design parameters and performance attributes are often very complex, resulting in a highly iterative and unguided process. In this paper, we argue that a more goal-oriented design process is enabled by an inverse formulation that starts with performance attributes instead of design parameters. A Deep Conditional Generative Design workflow is proposed that takes a set of performance attributes and partially defined design features as input and produces a complete set of design parameters as output. A model architecture based on a Conditional Variational Autoencoder is presented along with different approximate posteriors, and evaluated on four different case studies. Compared to Genetic Algorithms, our method proves superior when utilizing a pre-trained model.
更多查看译文
关键词
Deep generative modeling,Performance-based design,Generative design,Variational autoencoder,Deep generative design,Artificial intelligence
AI 理解论文
溯源树
样例
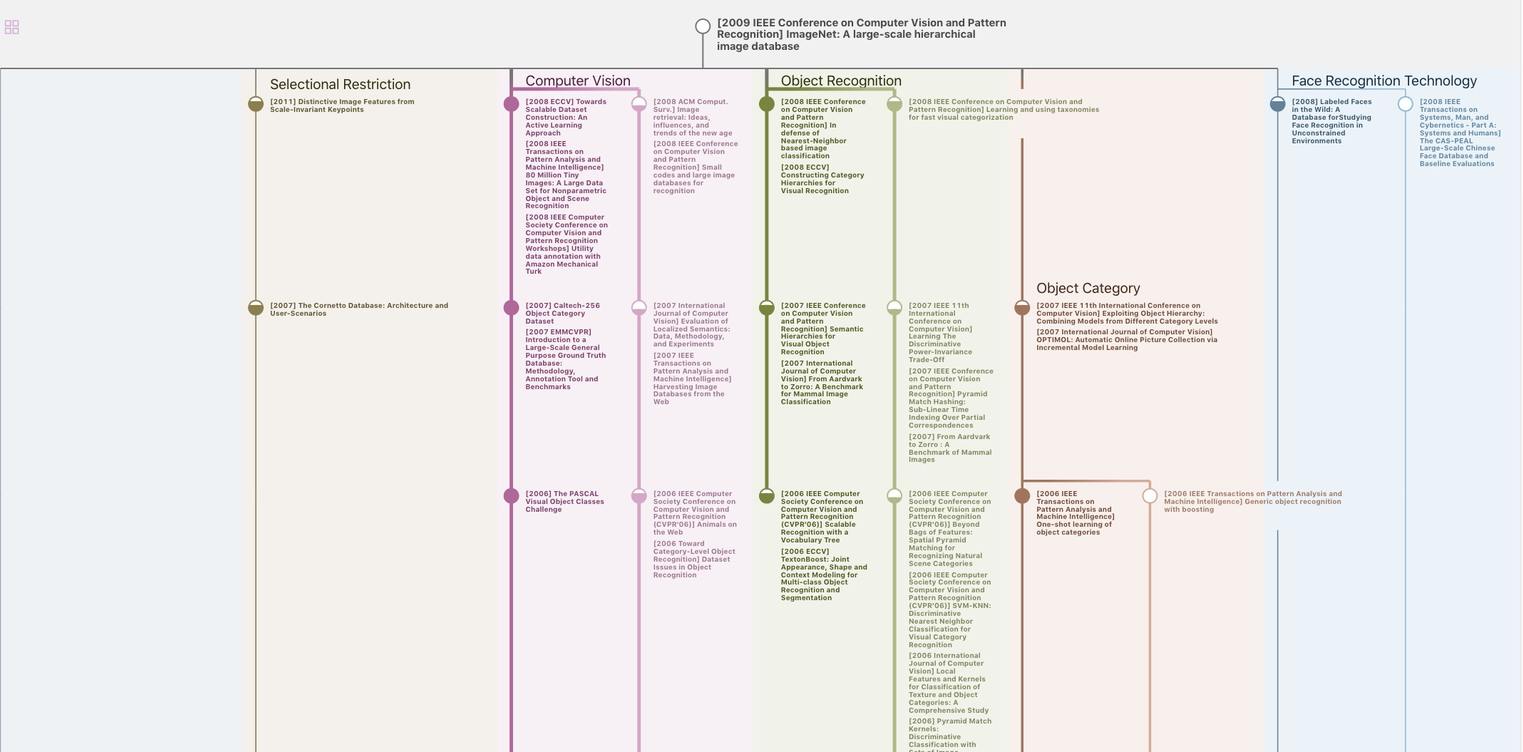
生成溯源树,研究论文发展脉络
Chat Paper
正在生成论文摘要