Multi-class classification of brain tumour magnetic resonance images using multi-branch network with inception block and five-fold cross validation deep learning framework
BIOMEDICAL SIGNAL PROCESSING AND CONTROL(2024)
摘要
The expertise of radiologists plays a pivotal role in the intricate task of diagnosing brain tumors. However, the escalating number of patients has rendered traditional diagnostic methods increasingly formidable and expensive. Consequently, researchers have diligently delved into the realm of medical image analysis to seek more efficient and precise approaches for the detection and classification of brain malignancies. In recent times, the surge in interest surrounding Deep Learning (DL) techniques has been notable, particularly in the development of automated systems with the capability to swiftly and reliably categorize brain tumors. The essence of this study revolves around the endeavor to confront the technical challenges inherent in this domain, with a specific focus on the intricate task of classifying brain tumors through the analysis of magnetic resonance images (MRI). The crux of the proposed model for brain tumor classification rests upon the foundation of Convolutional Neural Networks (CNNs), further fortified by a multi-branch network enriched with an inception block, elegantly incorporating a five-fold cross-validation methodology. This comprehensive approach was meticulously cali-brated and evaluated using the Br35H dataset, a repository encompassing 395 images devoid of tumors, 826 images depicting gliomas, 822 portraying meningiomas, and 827 capturing pituitary tumors. This research diligently concentrates on discerning among three tumor types, including scenarios where no tumors are present, and the outcomes are meticulously juxtaposed against an array of cutting-edge classification models, such as the Support Vector Classifier (SVC), Decision Tree Classifier, Logistic Regression, and Gaussian Naive Bayes. To provide a thorough assessment of the proposed model's prowess, a battery of performance metrics was subjected to intense scrutiny, spanning accuracy, loss, and variance. The meticulous analysis culminated in the revelation that the CNN with Multi-Branch Network and Inception block exhibited unparalleled excellence, achieving an awe-inspiring accuracy rate of 99.30% and a scarcely perceptible variance of 0.0005 in its classification of the four distinct tumor classes, firmly establishing its supremacy over the existing state-of-the-art models in the field.
更多查看译文
关键词
Brain tumor,Convolutional neural network,Classification,Deep learning,Five-fold cross-validation,Multi-branch network,Magnetic resonance imaging
AI 理解论文
溯源树
样例
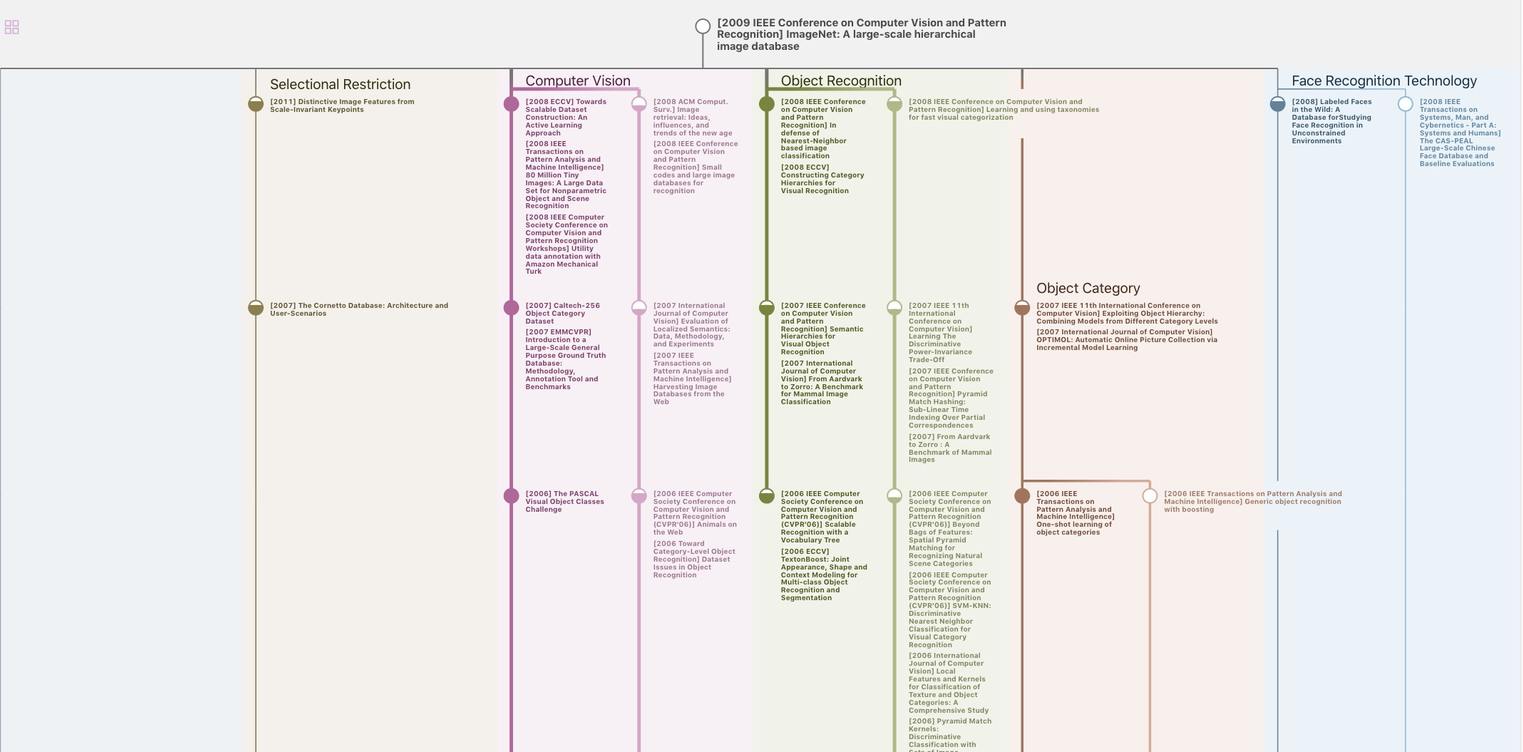
生成溯源树,研究论文发展脉络
Chat Paper
正在生成论文摘要