Solar power generation forecasting by a new hybrid cascaded extreme learning method with maximum relevance interaction gain feature selection
Energy Conversion and Management(2023)
摘要
Today, renewable energies have a key role in sustainable and clean power generation. On the other hand, solar energy is available in most regions in developing economies. Therefore, photovoltaic power forecasting is crucial for low-carbon power operation, planning, and trading. In this paper, the proposed hybrid cascaded forecasters network model combines LSTM, NARX, ELM, MRIG feature selection, and WT techniques, enabling accurate Solar Power Generation Forecasting (SPGF) for the next 24 h. By integrating these diverse models, the hybrid approach capitalizes on the strengths of each model and compensates for their weaknesses. This synergy significantly enhances the efficiency and accuracy of the forecasting method, leading to improved SPGF per-formance. The results of day-ahead SPGF for this case study demonstrate the hybrid model's impressive accuracy. For instance, the average prediction error for SPGF of the Mahan PV power plant resulted in a MAPE of 4.7213%, MAE of 0.0100 MW, and RMSE of 0.0198 MW.
更多查看译文
关键词
Feature selection, Cascaded forecasters, Solar power generation, Long short-term memory, Nonlinear autoregressive model with, eXogenous inputs, Extreme learning machine, Maximum relevance interaction gain
AI 理解论文
溯源树
样例
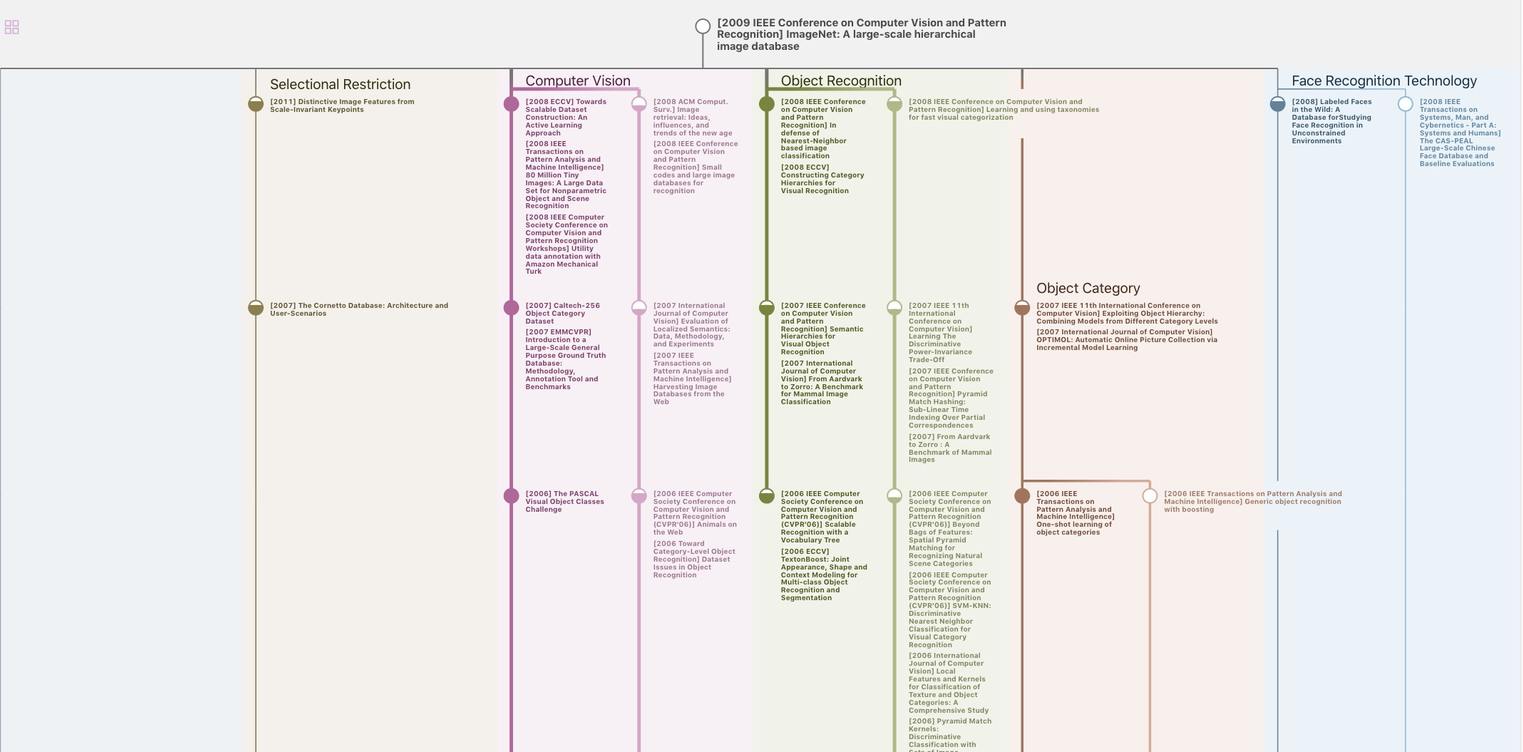
生成溯源树,研究论文发展脉络
Chat Paper
正在生成论文摘要