Laser welding defects detection in lithium-ion battery poles
ENGINEERING SCIENCE AND TECHNOLOGY-AN INTERNATIONAL JOURNAL-JESTECH(2023)
摘要
Strong demand for electric vehicles and energy storage applications has led to a rapid expansion of the battery sector. Laser welding is widely used in lithium-ion batteries and manufacturing companies due to its high energy density and capability to join different materials. Welding quality plays a vital role in the durability and effectiveness of welding structures. Therefore, it is essential to monitor welding defects to ensure welds quality. Manual inspection, analysis and evaluation of welding defect images is difficult due to the non-uniformity in their shape, position, and size. Hence the use of deep learning techniques to identify welding defects is more accurate and reliable due to the adequate training data samples, which helps to identify welding defects with greater accuracy. In this study, we present a novel collection of 3,736 laser welding images which are labeled with eight classes. This dataset contains both normal and defective classes collected from a Dade Laser Chinese production line. Moreover, we introduce a modified loss function that integrates cross entropy and complement objective training. The EfficientNetB6 model is trained with the newly introduced loss function that performed efficiently and achieved a promising accuracy during the classification phase. The experimental results demonstrate the significance of the proposed loss function and the sufficiency of EfficientNetB6 model on the laser welding dataset. Compared to other models, EfficientNetB6 performs better.
更多查看译文
关键词
Laser welding,Data sampling,Complement objective training,Multi-classification
AI 理解论文
溯源树
样例
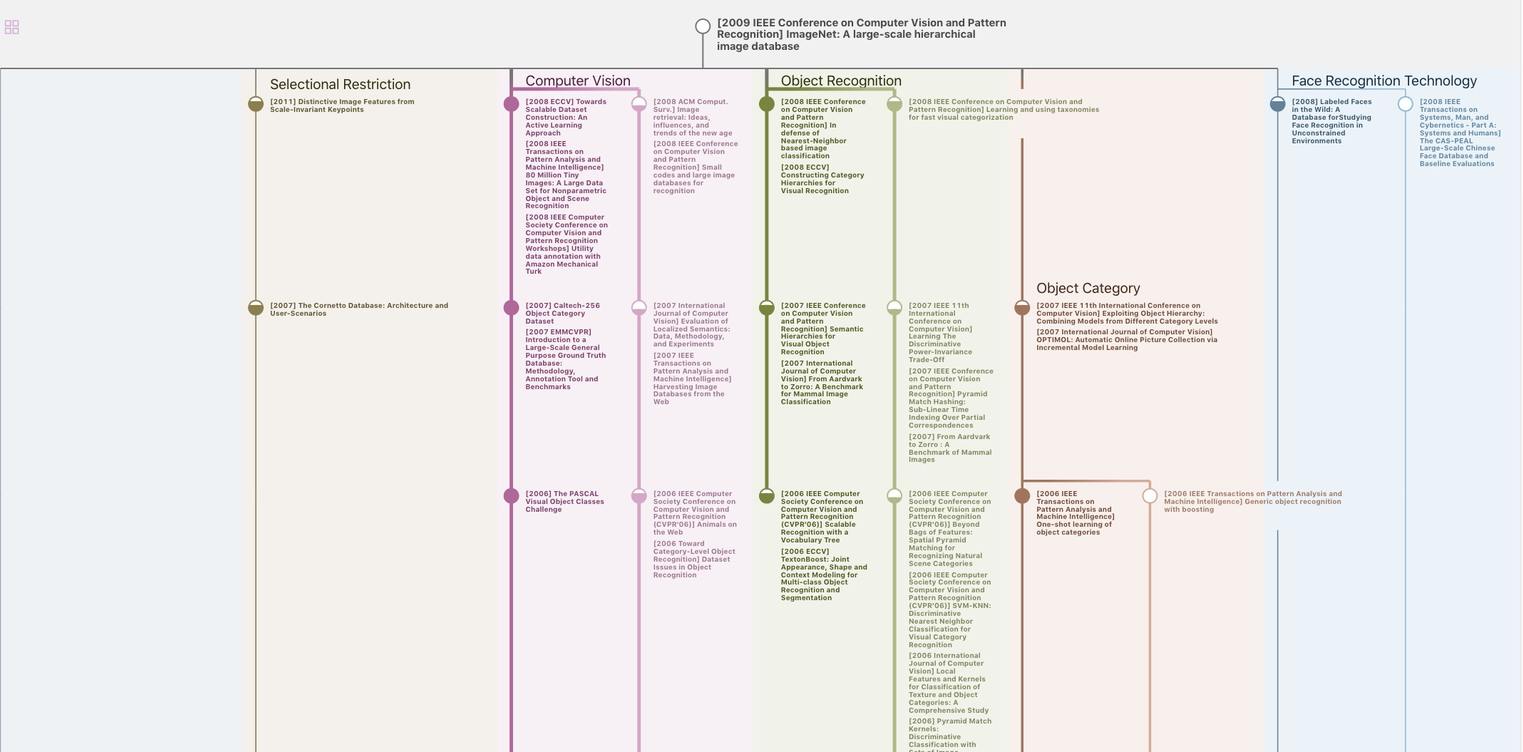
生成溯源树,研究论文发展脉络
Chat Paper
正在生成论文摘要