A Primary task driven adaptive loss function for multi-task speech emotion recognition
ENGINEERING APPLICATIONS OF ARTIFICIAL INTELLIGENCE(2024)
摘要
Although Speech Emotion Recognition (SER) is becoming an active research area, the state-of-the-art per-formance is limited by the scarcity of emotional datasets. The introduction of Multi-Task Learning (MTL) which jointly trains multiple relative tasks, can utilize more emotion-related information within limited data, enhancing the SER performance but also increasing the training difficulty. In this paper, we propose a primary-task driven adaptive loss function named as PTDA-Loss to adaptively adjust the task weights in the loss function, aiming to more reasonably allocate the learning resources in the training process and further improve the SER performance. We observe that excessive concern with the auxiliary task can lead to insufficient learning resources for SER, not conducive to SER performance improvement. Inspired by this finding, in PTDA-Loss, we retain the advantage of SER in the whole training process. Meanwhile, the learning resources for the auxiliary task will be appropriately but not over compressed, ensuring the effective learning of useful information from the auxiliary task. To improve the model's learning ability, furthermore, we first employ several dynamic convolutional layers as the backbone network. Regarding gender recognition and speaker recognition as the auxiliary task respectively, ablation experiments on the IEMOCAP, MSP-IMPROV and MELD datasets prove the rationality and effectiveness of PTDA-Loss. Additionally, comparative experiments demonstrate that our method achieves state-of-the-art performance on the three datasets, further enhancing the SER accuracy.
更多查看译文
关键词
Human-computer interaction,Multi-task learning,Adaptive training,Experimental analysis
AI 理解论文
溯源树
样例
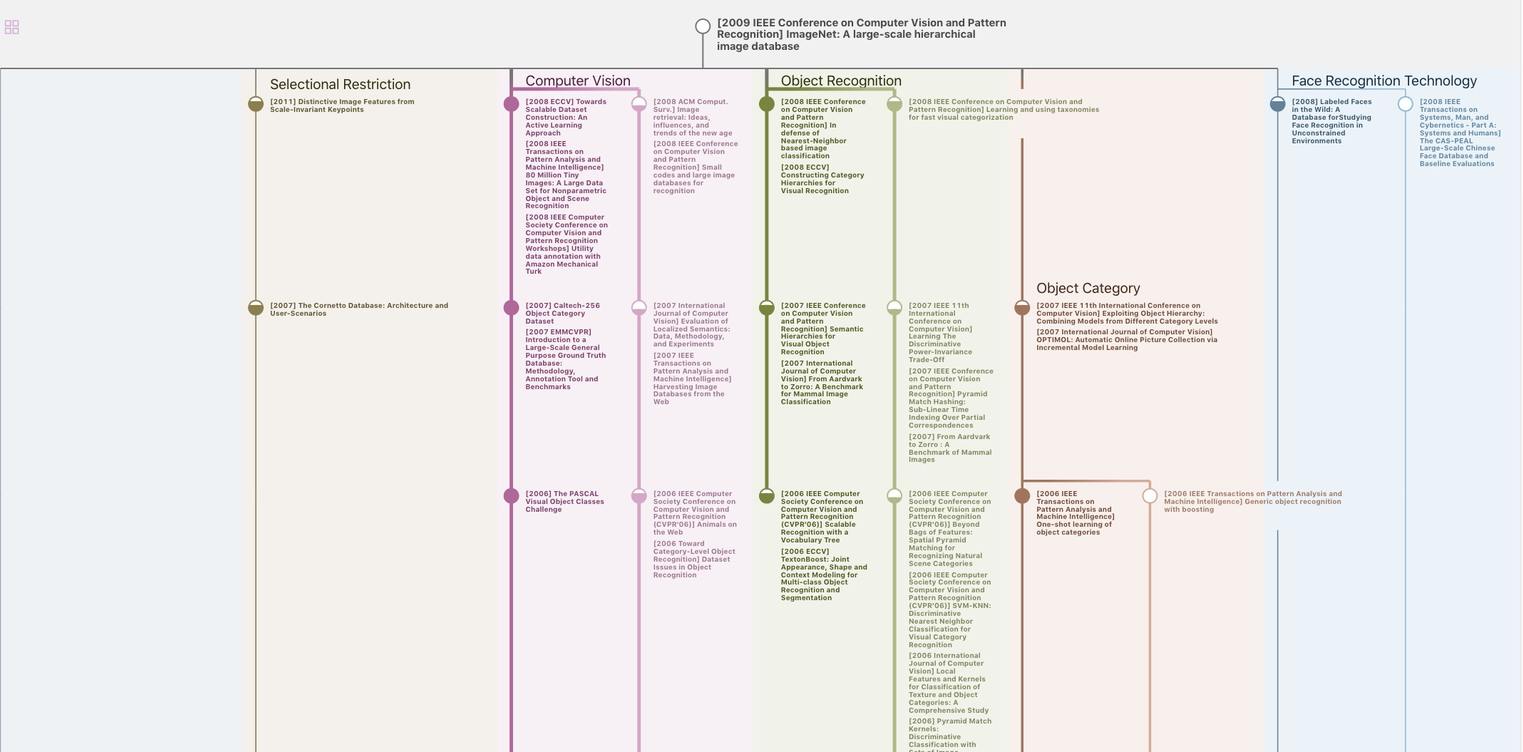
生成溯源树,研究论文发展脉络
Chat Paper
正在生成论文摘要