Automated bacteria genera classification using histogram-oriented optimized capsule network
ENGINEERING SCIENCE AND TECHNOLOGY-AN INTERNATIONAL JOURNAL-JESTECH(2023)
摘要
Identifying the nature and type of bacteria is essential in diagnosing various fatal diseases and their treatments. Biologists classify bacteria using morphological characterization from microscopic images according to their color and shape information. Therefore, an automated bacterial recognition and classification approach is required compared to a challenging and time-consuming manual process. Much research has been carried out on bacteria classification using machine learning algorithms. However, the major weakness of these conventional machine learning algorithms is that they cannot differentiate pose and deformation, have significant trainable parameters, require extensive training time, and use trial-and-error-based hyperparameter selection. In addition, the choice of optimization function to reduce the loss is also equally important. This paper presents an efficient capsule network that encodes information from orientation as an alternative to these machine learning models. The proposed model is designed with histogram-based feature sets requiring minimal parameters. Various optimization algorithms are tested to find an appropriate optimizer. 33 categories of bacteria species are classified using the proposed method. A comprehensive analysis of popular gradient descent optimizers is presented with a capsule network to strengthen testing and validation and benchmark the performance. The extensive empirical study on DiBAS datasets demonstrates that the proposed network performs 95.08% efficiency among various machine learning algorithms, including KNN, Decision Trees, Naive Bayes and SVM. Furthermore, the proposed model achieves better accuracy with the least training parameters of 6.9 million.
更多查看译文
关键词
Bacteria classification,Machine learning,Deep learning,Capsule Network,Optimization
AI 理解论文
溯源树
样例
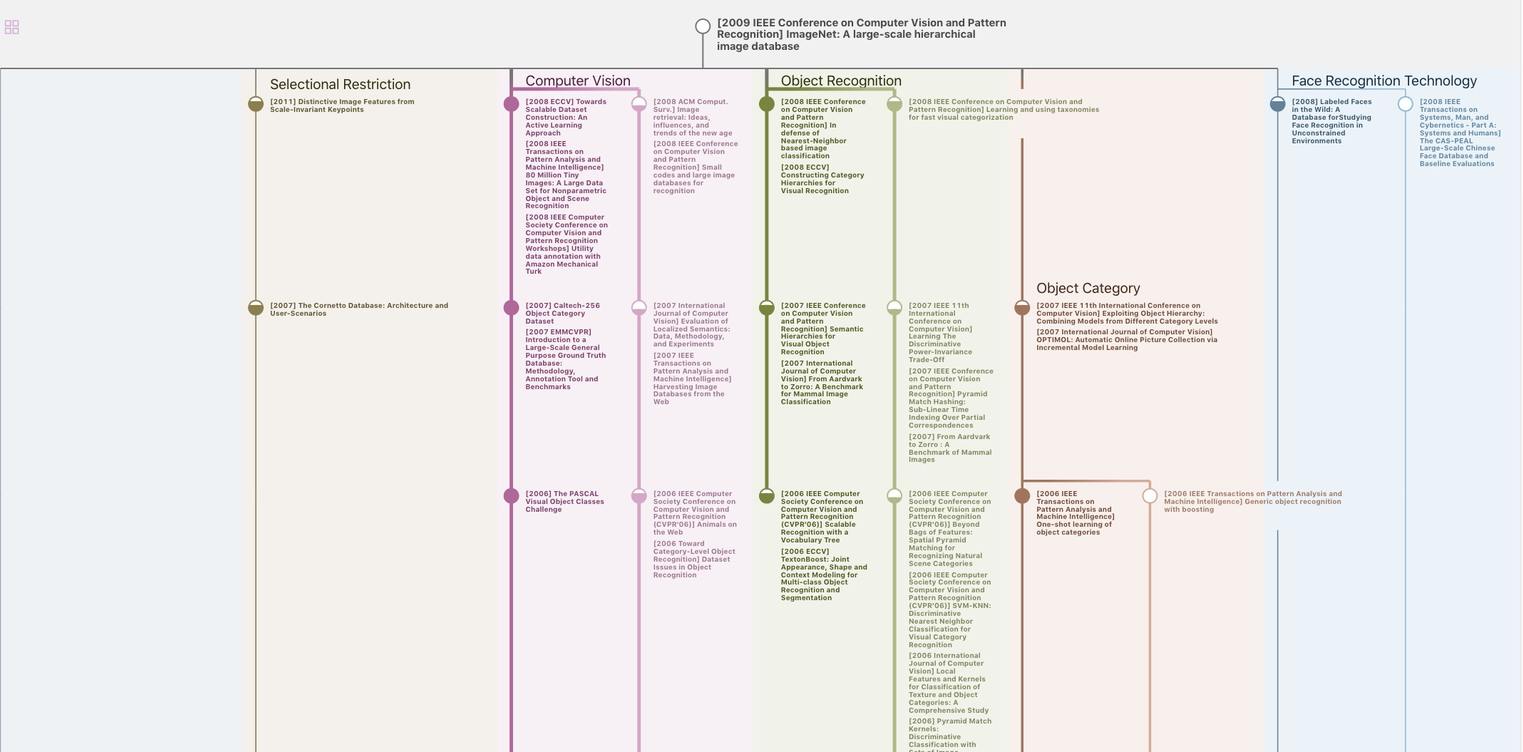
生成溯源树,研究论文发展脉络
Chat Paper
正在生成论文摘要