Gaussian dynamic recurrent unit for emitter classification
EXPERT SYSTEMS WITH APPLICATIONS(2024)
摘要
Emitter classification plays a crucial role in electronic support measurement systems. A data-driven model named Gaussian dynamic recurrent unit is proposed to accomplish the end-to-end emitter classification tasks, which is simplified based on the long short-term memory unit by using a Gaussian function to characterize the relationship between the input and the network state, and a learnable exponentially weighted average to update the states. The Gaussian function and the dynamic exponentially weighted average amplify the difference between the input signals from different classes. The parameter size and computational time of many emitter classification methods are measured. The results show that the model proposed in this paper has the highest parameter efficiency, and low computational and storage costs. The results on a real-world dataset show that the proposed model has the highest accuracy and stability. These advantages have important significance for deploying the model in practical applications.
更多查看译文
关键词
Emitter classification,Electronic support measurement,Gaussian dynamic recurrent unit,Recurrent neural network (RNN)
AI 理解论文
溯源树
样例
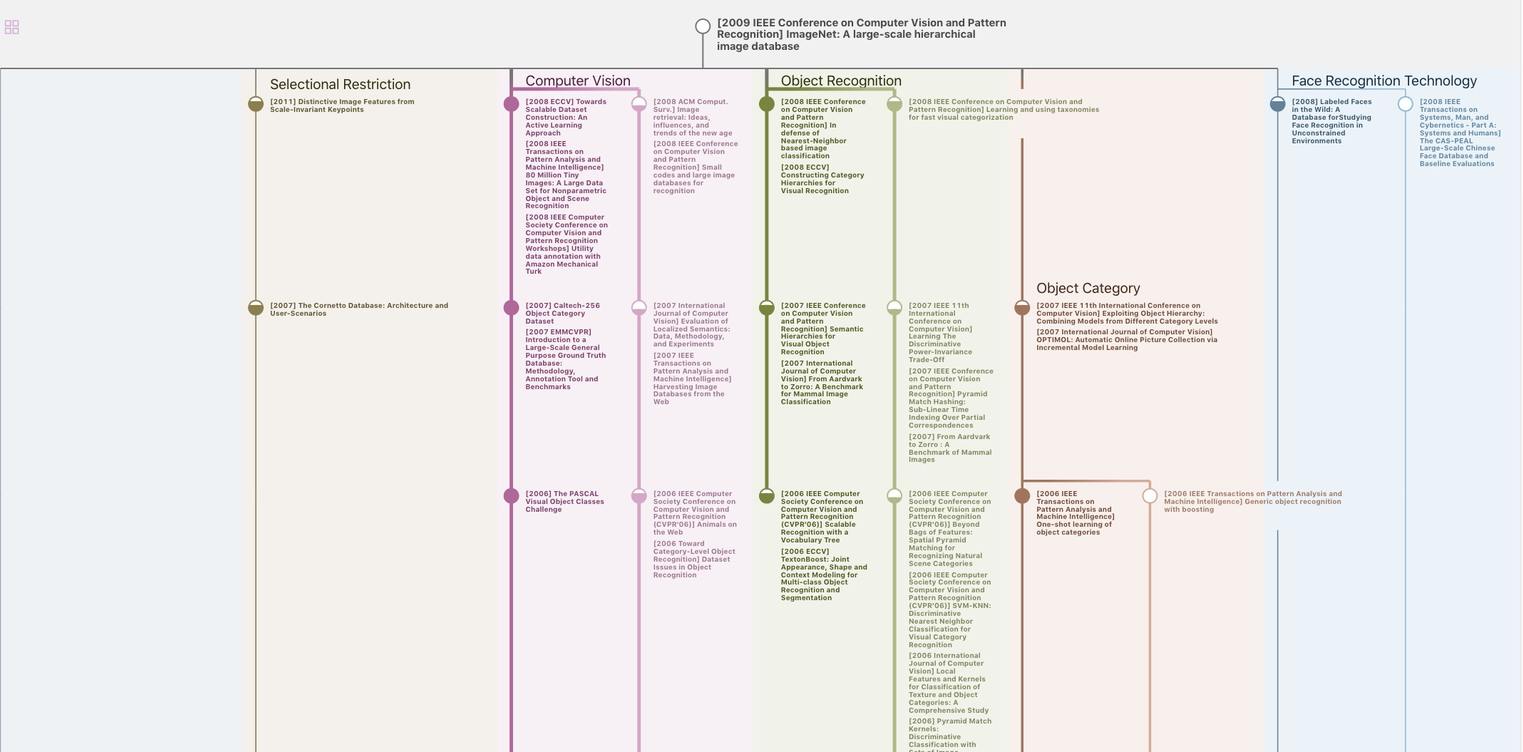
生成溯源树,研究论文发展脉络
Chat Paper
正在生成论文摘要