Two-stage coarse-to-fine image anomaly segmentation and detection model
Image and Vision Computing(2023)
摘要
Existing Convolutional Neural Network (CNN) based anomaly detection and segmentation approaches are overly sensitive or not sensitive enough to noise, resulting in anomaly patterns, partially detected in the testing stage. The previous methods may also differentiate normal and abnormal images, but they cannot identify the location of anomaly presented in test images with high accuracy. To address this issue, we propose a two-stage CNN model for coarse-to-fine anomaly segmentation and detection called (TASAD). In both stages of TASAD, we train our model on a mixture of normal and abnormal training samples. The abnormal images are obtained by inserting pseudo-anomaly patterns that are automatically generated from anomaly source images. We use a novel and sophisticated anomaly insertion technique to generate various anomalous samples. In the first stage, we design a coarse anomaly segmentation (CAS) model that takes a whole image as an input, while in the second stage, we train a fine anomaly segmentation (FAS) model on image patches. FAS model improves detection and segmentation performance by refining anomaly patterns partially detected by CAS model. We train our framework on MVTec dataset and compare it with state-of-the-art (SOTA) methods. The proposed architecture leads to a compact model size – four times smaller than the SOTA method, while exhibiting better pixel-level accuracy. TASAD can also be applied to SOTAs to further improve their anomaly detection performance. Our experiments demonstrate that when applied to the latest SOTAs, TASAD improves the average precision (AP) performance of previous methods by 6.2%. For reproducibility of the results, code is provided at https://github.com/RizwanAliQau/tasad.git.
更多查看译文
关键词
Anomaly detection and segmentation,Convolutional neural network,Pseudo anomaly insertion,Superpixel segmentation
AI 理解论文
溯源树
样例
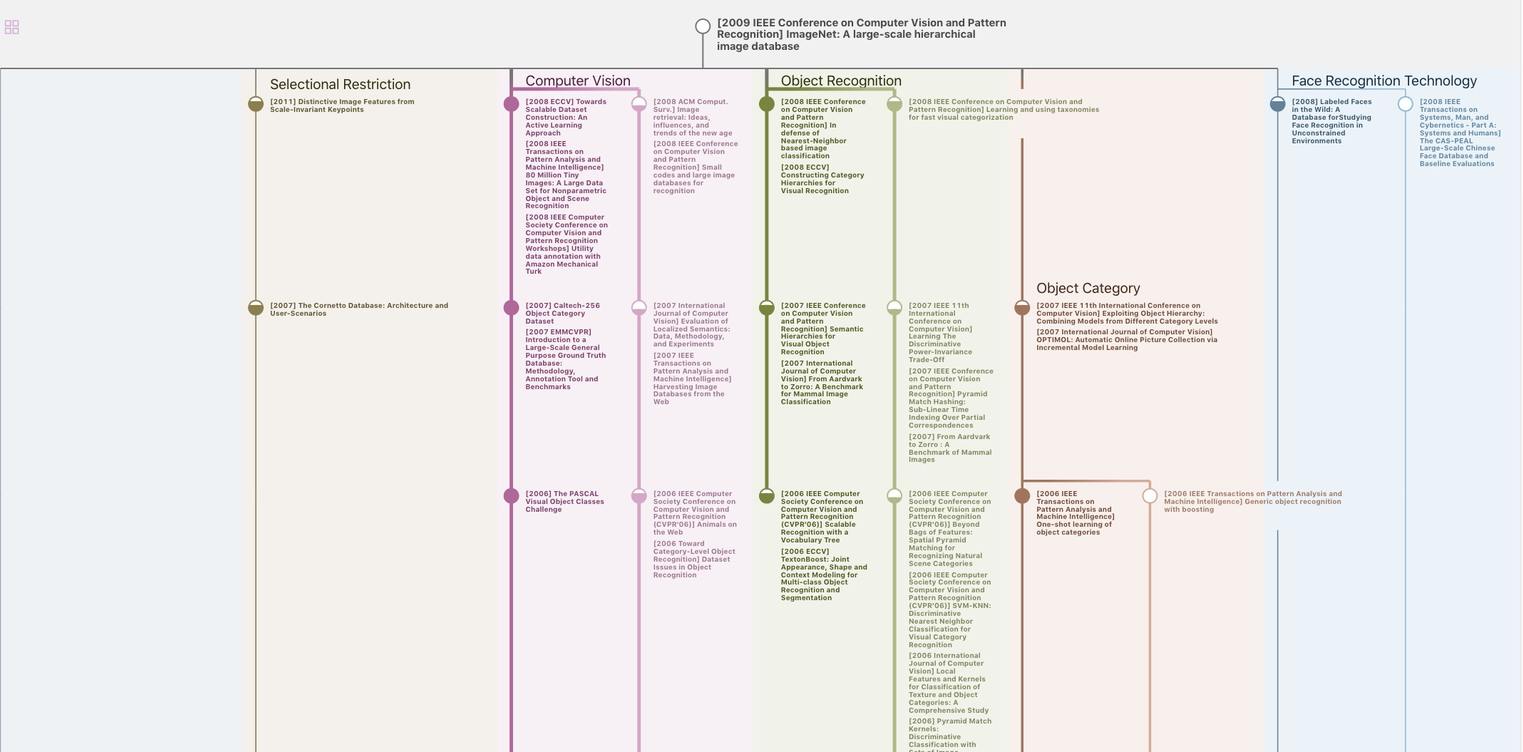
生成溯源树,研究论文发展脉络
Chat Paper
正在生成论文摘要