Document-level denoising relation extraction with false-negative mining and reinforced positive-class knowledge distillation
INFORMATION PROCESSING & MANAGEMENT(2024)
摘要
Many datasets for document-level relation extraction (RE) suffer from incomplete labeling, par-ticularly the false negative problem, which induces improper biases during training. However, existing denoising methods are either limited to the scale of dataset or primarily focus on sentence-level RE. To tackle this prevalent issue of false negatives, we propose a denoising framework called FM-RKD for document-level RE. Firstly, a false-negative mining mechanism is introduced to identify and re-annotate false negative samples (FNs) within the original corpus, thereby producing a higher-quality pseudo corpus. Then, we propose a reinforced positive-class knowledge distillation method, where a teacher network trained with positive samples provides soft labels for a student network. This approach enables the student network to learn complete positive-class patterns and mitigate the overfitting issue caused by FNs. Extensive experiments on the Re-DocRED dataset show that FM-RKD outperforms the current state-of-the-art method by 1.36% in F1 score and 1.24% in Ign F1 score when the training data is incompletely annotated. Moreover, FM-RKD consistently achieves new peak performance with an F1 score of 78.38% even when the training data is well-annotated.
更多查看译文
关键词
Document-level relation extraction,Incomplete labeling data,False-negative mining mechanism,Reinforced positive-class knowledge distillation
AI 理解论文
溯源树
样例
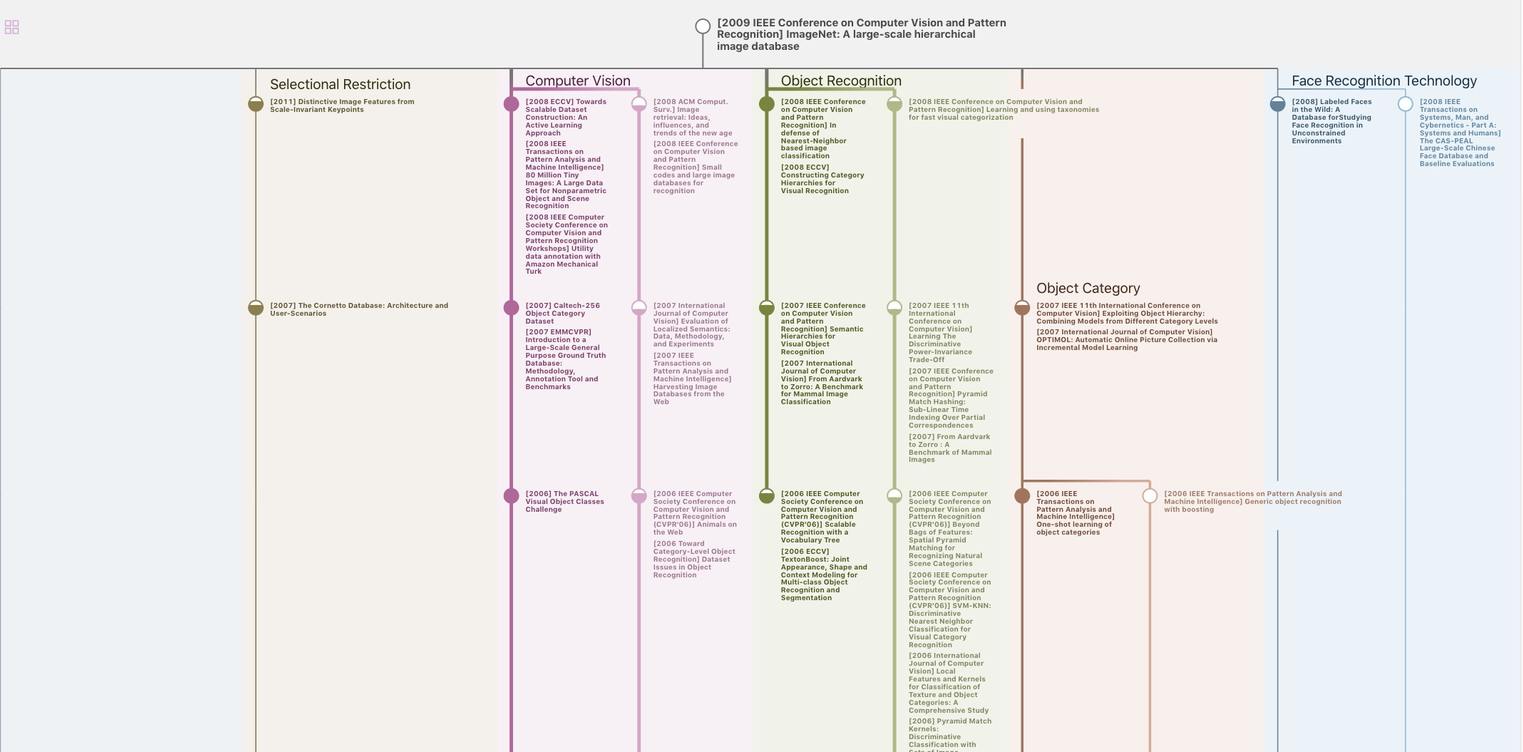
生成溯源树,研究论文发展脉络
Chat Paper
正在生成论文摘要