High-temperature high-cycle fatigue performance and machine learning-based fatigue life prediction of additively manufactured Hastelloy X
INTERNATIONAL JOURNAL OF FATIGUE(2024)
摘要
Uncertainties in fatigue life of laser powder bed fusion (L-PBF) additively manufactured parts arise from microstructural heterogeneities and randomly dispersed defects generated during the L-PBF. Stress-controlled fatigue performances of L-PBF-built Hastelloy X were examined in 400 degrees C and 600 degrees C high-temperature atmo-spheres. Given the inherent anisotropy of the L-PBF-built Hastelloy X, the fatigue tests were performed on the samples with distinct building orientations. Fatigue-induced damage evolution characteristics were meticulously analyzed after drawing the S-N curves with a 0.1 stress ratio, a 20 Hz loading frequency and varied stress am-plitudes. We established a database of the fatigue test results with material properties of the L-PBF-built Has-telloy X to build a machine learning (ML) framework for fatigue life prediction, skipping the traditional fatigue-life mathematical models that are less applicable to L-PBF-built metals. For capturing the intricate influence of diverse factors on the fatigue life accurately, deep neural network (DNN) and support vector machine (SVM) were utilized and optimized within the ML-based framework. By comparing our ML-based approach with traditional methods for life prediction, it is indicated that ML techniques can transcend the physical limitations associated with the fatigue processes while simultaneously offering a unified depiction thereof.
更多查看译文
关键词
Fatigue life,Laser powder bed fusion,Additive manufacturing,High temperature,Machine learning
AI 理解论文
溯源树
样例
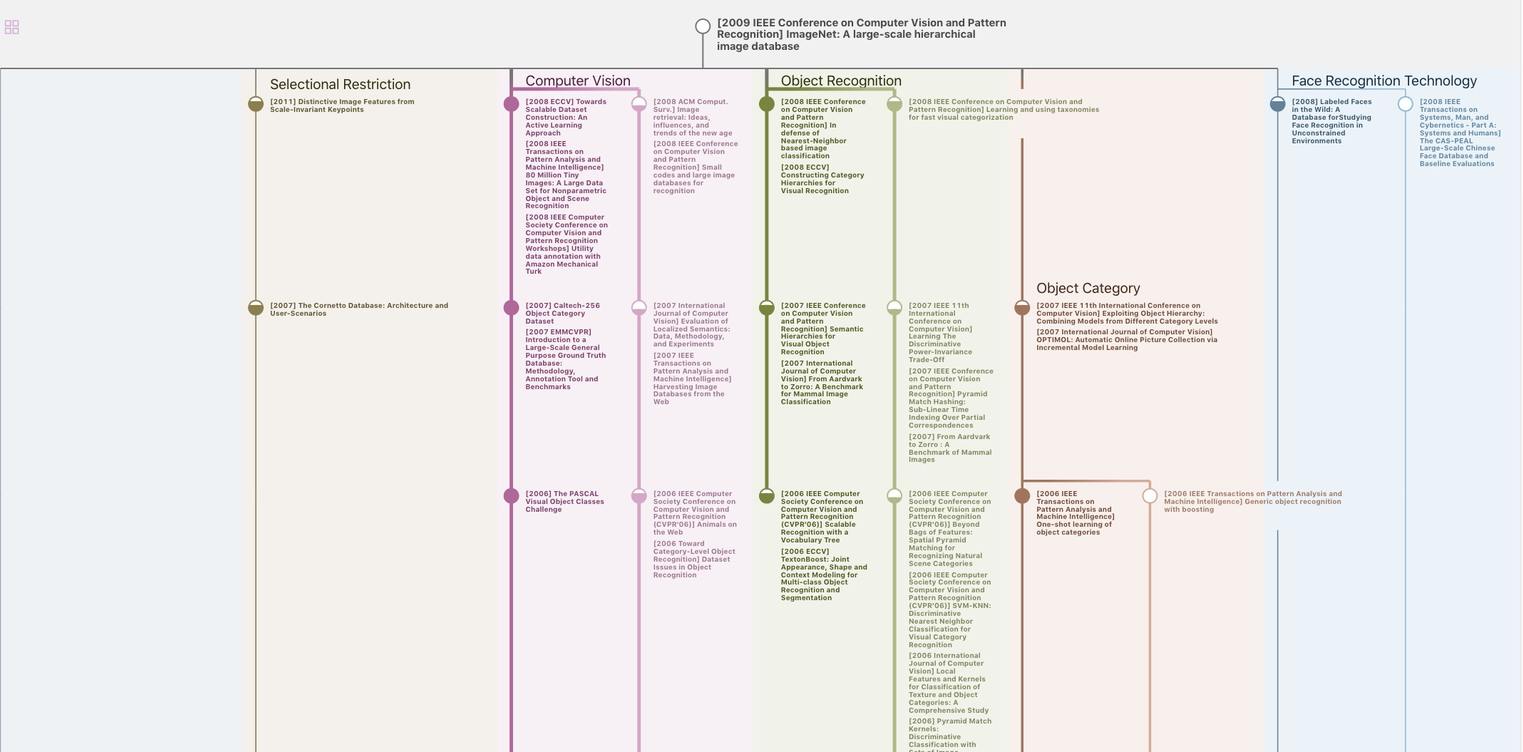
生成溯源树,研究论文发展脉络
Chat Paper
正在生成论文摘要