Performance of a deep-learning algorithm in detecting and discriminating nodules on chest radiographs
CHEST(2023)
摘要
SESSION TITLE: Lung Cancer Posters 1 SESSION TYPE: Original Investigation Posters PRESENTED ON: 10/10/2023 12:00 pm - 12:45 pm PURPOSE: Lung cancer was the third most common cancer in the UK, with 51,983 cases in 2020, and it was the leading cause of cancer deaths1. Nodules are a common radiographic appearance of lung cancer, and various observer errors have been reported in the literature. This study was conducted to evaluate the performance of an AI in detecting nodules and classifying their malignancy potential. METHODS: CXRs of 40 patients with biopsy-confirmed lung cancer and 104 patients with CT-confirmed non-cancer were obtained from NHS Leicester. All 40 cancer cases and 5 of the 104 controls had a detectable nodule. The control arm was enriched with the 31 non-malignant NLST nodule CXRs, which are publicly available 2. All images were processed with qXR lung nodule and a proprietary lung cancer model. Nodule output was binarized at the default manufacturer threshold, and the cancer score was binarized at the highest Youden’s index operating point. RESULTS: Overall, 40 cancer cases (all with nodules) and 135 non-cancer cases (36 with nodules) were included in the analysis. The sensitivity and specificity of qXR in nodule detection were 88.2% (79.0-93.6) and 85.6% (77.7-91.4), respectively. The AUC was 0.931 (0.889-0.973). The sensitivity and specificity of the cancer model in classifying cancer cases in the entire dataset were 92.5% (80.1-97.4) and 70.4% (62.2-77.4), respectively. When the analysis was restricted to only images with nodules (n = 76), the model had a high specificity of 97.7% and a sensitivity of 32.5%. CONCLUSIONS: This study demonstrated that AI could detect nodules with high accuracy. The cancer model also had high sensitivity and moderate specificity in detecting cancer cases. The drop in sensitivity of the model, when restricted to nodule alone population, is due to thresholding at the highest Youden’s index in a dataset that contained many suspicious lesions but did not turn out to be cancer in follow-up (NLST images). The cancer score can be calibrated to achieve the ideal sensitivity. CLINICAL IMPLICATIONS: Although LDCT screening increases survival in the screened population, it is not implemented universally. These screenings do not cover never-smokers; 15% of male and 50% of female lung cancer patients are never-smokers3. Identifying nodules in CXR for other indications and appropriate management can improve survival, and AI can act as a safety net in these cases. This is particularly more important for resource constrained areas where CT might not be easily available. Reference: 1. International Agency for Research on Cancer. United Kingdom Fact Sheet. World Health Organization; 2021. https://gco.iarc.fr/today/data/factsheets/populations/826-united-kingdom-fact-sheets.pdf 2. Homayounieh F, Digumarthy S, Ebrahimian S, et al. An Artificial Intelligence-Based Chest X-ray Model on Human Nodule Detection Accuracy From a Multicenter Study. JAMA Netw Open. 2021;4(12):e2141096. doi:10.1001/jamanetworkopen.2021.41096 3. Parkin DM, Bray F, Ferlay J, Pisani P. Global cancer statistics, 2002. CA Cancer J Clin. 2005;55(2):74-108. doi:10.3322/canjclin.55.2.74 DISCLOSURES: No relevant relationships by Rohitashva Agrawal No relevant relationships by Subhankar Chattoraj No disclosure on file for Sai Kiran No relevant relationships by Ashish Mittal Employee relationship with Qure.ai Please note: Current employee by Preetham Putha, value=Salary Employee relationship with Qure.ai Please note: Current Employee by Saigopal Sathyamurthy, value=Salary No relevant relationships by Anshul Singh No disclosure on file for Manoj Tadepalli
更多查看译文
关键词
deep-learning deep-learning algorithm,nodules,deep-learning deep-learning
AI 理解论文
溯源树
样例
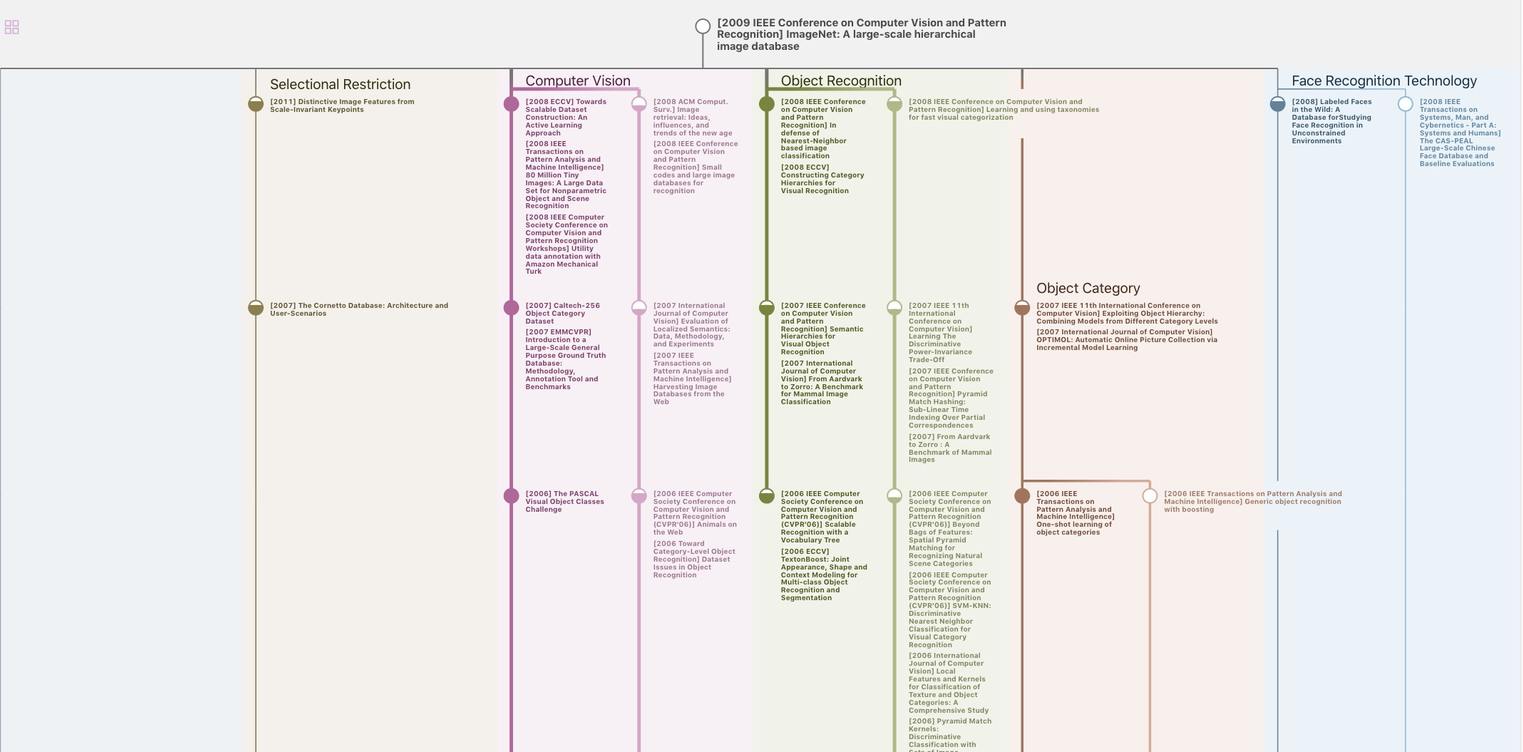
生成溯源树,研究论文发展脉络
Chat Paper
正在生成论文摘要