SenPred: A single-cell RNA sequencing-based machine learning pipeline to classify senescent cells for the detection of an in vivo senescent cell burden
bioRxiv (Cold Spring Harbor Laboratory)(2023)
摘要
Senescence classification is an acknowledged challenge within the field, as markers are cell-type and context dependent. Currently, multiple morphological and immunofluorescence markers are required for senescent cell identification. However, emerging scRNA-seq datasets have enabled increased understanding of the heterogeneity of senescence. Here we present SenPred, a machine-learning pipeline which can identify senescence based on single-cell transcriptomics. Using scRNA-seq of both 2D and 3D deeply senescent fibroblasts, the model predicts intra-experimental and inter-experimental fibroblast senescence to a high degree of accuracy (>99% true positives). We position this as a proof-of-concept study, with the goal of building a holistic model to detect multiple senescent subtypes. Importantly, utilising scRNA-seq datasets from deeply senescent fibroblasts grown in 3D refines our ML model leading to improved detection of senescent cells in vivo. This has allowed for detection of an in vivo senescent cell burden, which could have broader implications for the treatment of age-related morbidities.
### Competing Interest Statement
The authors have declared no competing interest.
更多查看译文
关键词
senescent cells,rna,single-cell,sequencing-based
AI 理解论文
溯源树
样例
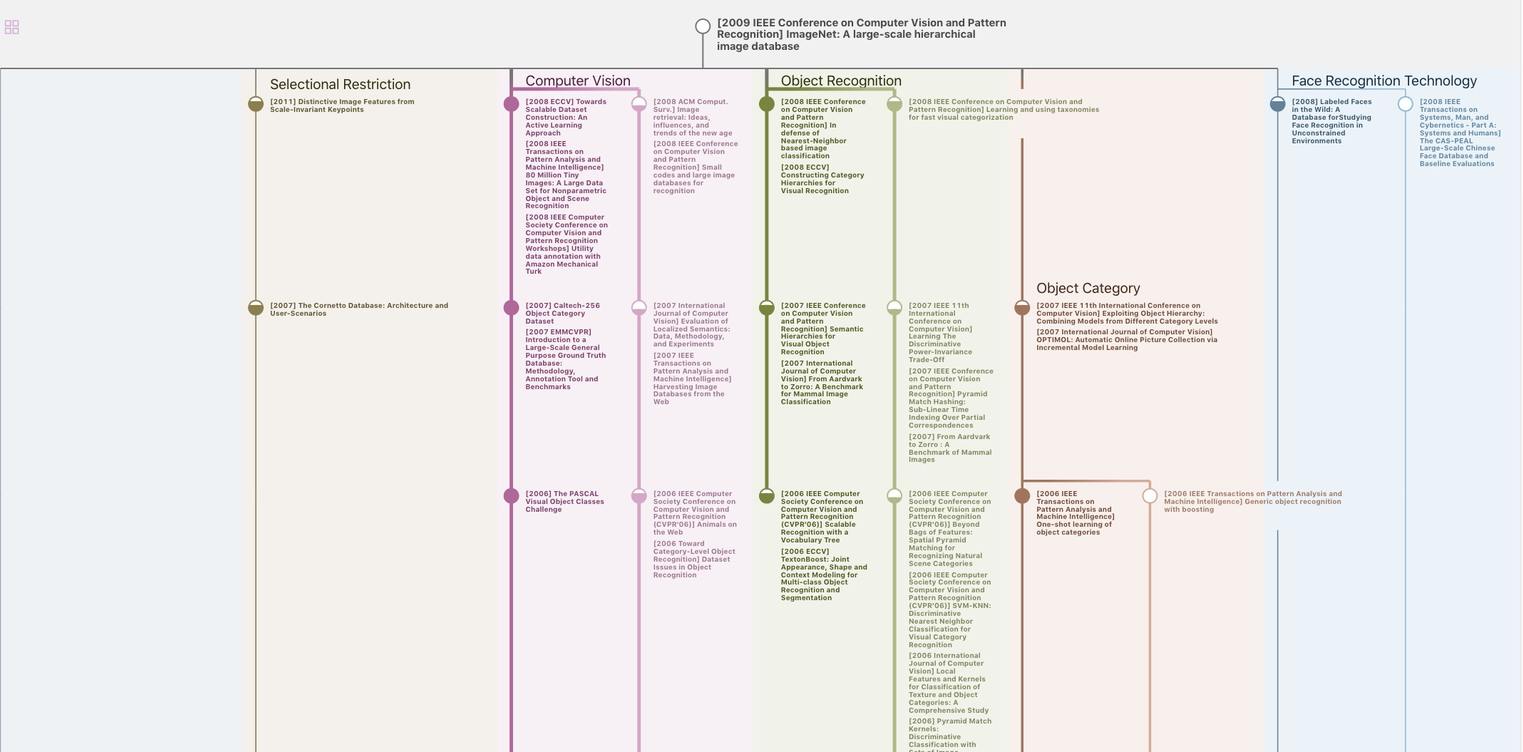
生成溯源树,研究论文发展脉络
Chat Paper
正在生成论文摘要