Two-stage support vector machine-enabled deep excavation settlement prediction considering class imbalance and multi-source uncertainties
RELIABILITY ENGINEERING & SYSTEM SAFETY(2024)
摘要
This paper proposes a robust ground settlement prediction framework that can cope with class imbalance and multi-source uncertainties within the practice of deep excavation. There are two main stages incorporated to achieve a reliable risk perception with high accuracy. The first stage involves the application of the Least Square Support Vector Machine (LSSVM) under a statistical learning process (SLP) for detecting settlement occurrences. The second stage utilizes the Least Square Support Vector Regression (LSSVR) under the coupled simulated annealing (CSA) optimizer to predict settlement evolution. It is followed by the construction of prediction intervals and a global sensitivity analysis (GSA) to facilitate deeper investigation. A real deep excavation project as part of Shanghai Metro is used as a case study to validate the effectiveness of the proposed framework, yielding high prediction accuracy in ground settlement prediction. Moreover, the prediction results can be expressed by two types of high-quality intervals as a promising description of uncertainties attributed to the intelligent model and collected data. Overall, the proposed two-stage LSSVM-based framework offers practical value as a decisionmaking support tool for stakeholders to understand and control the ground settlement as a reflection of risk status, contributing to enhancements of early risk perception and management in deep excavation engineering.
更多查看译文
关键词
Machine learning,Ground settlement prediction,Class imbalance,Multi-source uncertainty,Global sensitivity analysis
AI 理解论文
溯源树
样例
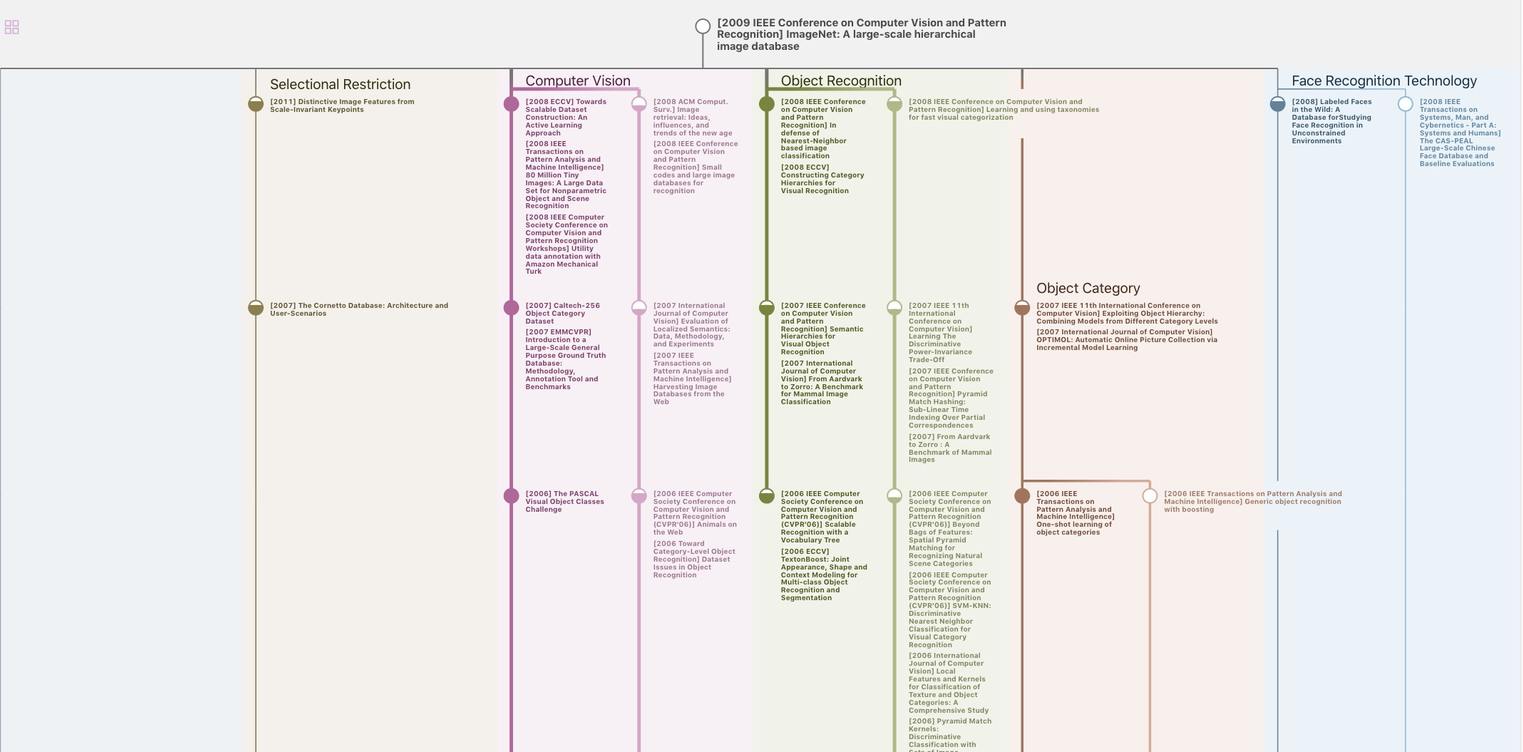
生成溯源树,研究论文发展脉络
Chat Paper
正在生成论文摘要