Dynamic-controlled principal component analysis for fault detection and automatic recovery
RELIABILITY ENGINEERING & SYSTEM SAFETY(2024)
摘要
To effectively implement the prognostic and health management for industrial processes, a dynamic-controlled principal component analysis (DCPCA) for pattern extraction and deviation diagnosis is proposed under the framework of multivariate statistical modelling, which can accurately detect and automatically rectify the faults. Significantly, the geometric properties of DCPCA are analysed, revealing the spatial structure relationships of different variables and how the data space is partitioned. In addition, the model relationships in DCPCA are explored, including the dynamic characteristics of time-series variables and the algebraic ones of static variables. Based on these results, statistics are derived for monitoring both the dynamic and static relationships of the process, and under the abnormal circumstance, by diagnosing the deviations between the fault pattern and the setpoint, a fault regulator for automatic recovery is designed. The case study of prognostic and health management for an industrial distillation column illustrates the advantages of DCPCA in fully extracting the process dynamics into pattern, as well as fault detection and automatic recovery.
更多查看译文
关键词
Prognostic and health management,Dynamic controlled principal component,analysis,Geometric properties,Model relationships,Fault detection,Fault automatic recovery
AI 理解论文
溯源树
样例
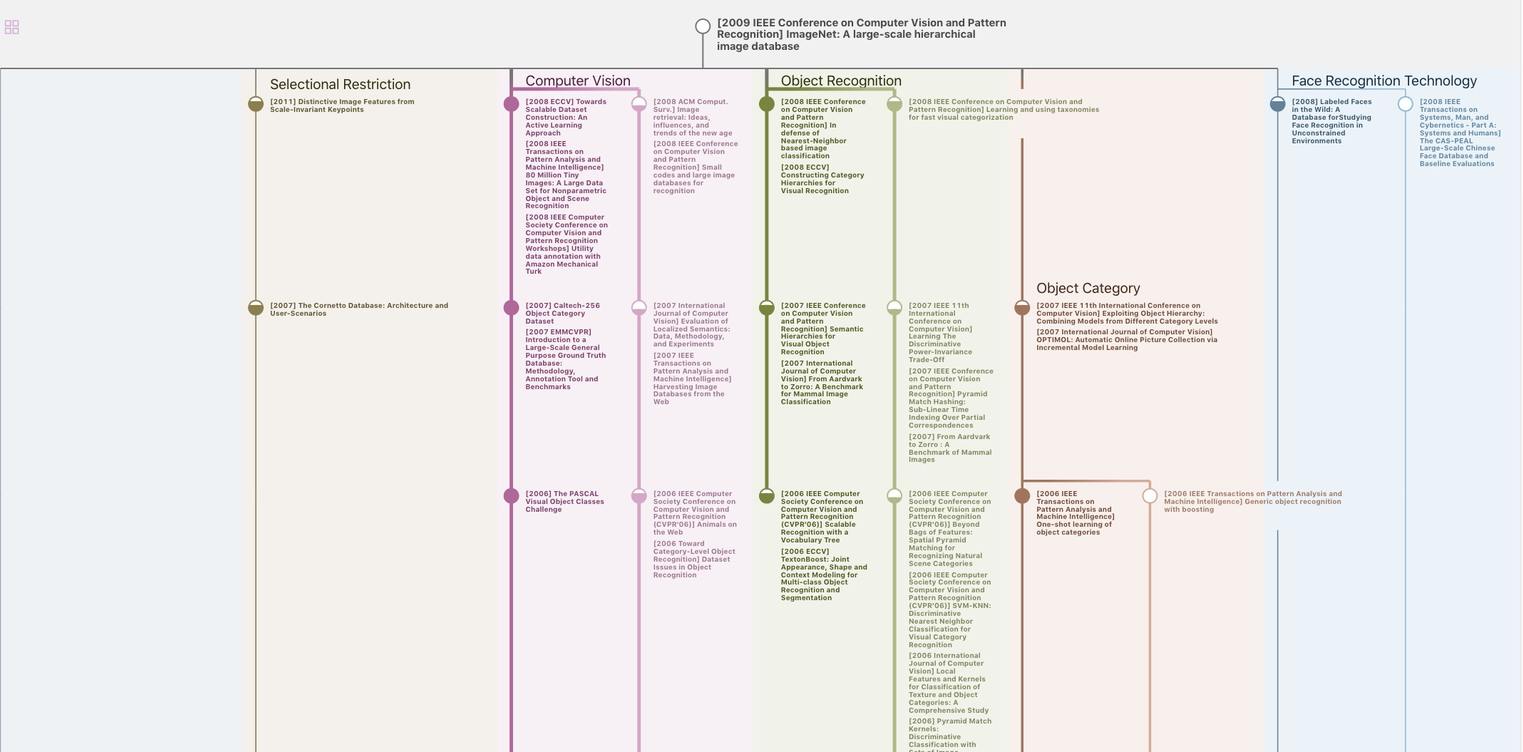
生成溯源树,研究论文发展脉络
Chat Paper
正在生成论文摘要