Joint maintenance and spare part ordering from multiple suppliers for multicomponent systems using a deep reinforcement learning algorithm
RELIABILITY ENGINEERING & SYSTEM SAFETY(2024)
摘要
This paper investigates the joint optimization of maintenance and spare part ordering from multiple suppliers for systems consisting of multiple components. When components degrade to poor conditions, they are replaced with spare parts if available. Spare parts can be purchased from multiple suppliers, each with distinct lead times, unit prices, and setup costs. To determine the optimal replacement and ordering decisions, this paper establishes a model through a Markov decision process and designs a value iteration algorithm to solve the model. However, the value iteration algorithm takes too much time to solve problems involving large numbers of components and suppliers. Thus, we design a hybrid deep reinforcement learning algorithm (HDRL) based on the reinforcement learning algorithm to solve large-scale problems. Numerical experiments are conducted to validate the effectiveness of the HDRL algorithm and analyze the joint decisions. The results show that, compared with the value iteration algorithm, the average cost gap is 3.86%, and the solving time can be reduced by at least 95.99% for systems with more than 2 suppliers and more than 3 components under the HDRL algorithm.
更多查看译文
关键词
Joint optimization,Maintenance,Multiple suppliers,Reinforcement learning,Value iteration
AI 理解论文
溯源树
样例
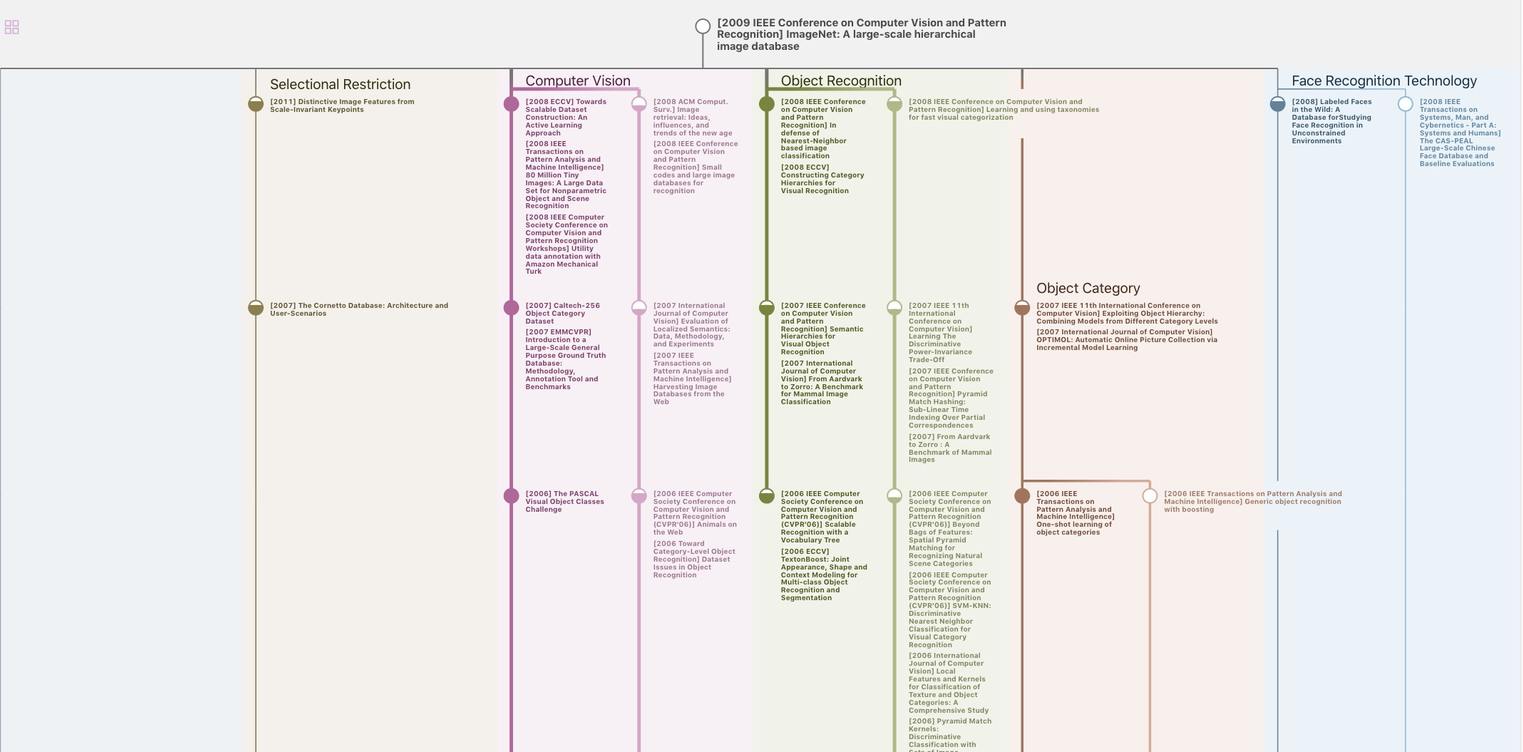
生成溯源树,研究论文发展脉络
Chat Paper
正在生成论文摘要