Frequency-domain Volterra kernel-based adaptation: Formulations and algorithms
SIGNAL PROCESSING(2024)
摘要
For the correlated input, the Volterra kernel-based least mean-square (LMS) algorithm in the time-domain exhibits a slow learning rate caused by the large eigenvalue spread of the input covariance matrix. To tackle such an issue, this paper develops a novel frequency-domain Volterra kernel-based filter, resulting in the periodic update constrained frequency-domain second-order Volterra normalized LMS (named as P-CFDSOV-NLMS1) algorithm. Subsequently, by using one-and two-dimensional discrete Fourier transforms (DFTs) simultaneously, another frequency-domain implementation and corresponding P-CFDSOV-NLMS2 algorithm are constructed. In contrast, the P-CFDSOV-NLMS1 scheme only requires one-dimensional DFT operations and takes advantage of the joint information between the block input vectors. Then, the mean and mean -square convergence behaviors of the P-CFDSOV-NLMS1 algorithm are investigated. Furthermore, the designed frequency-domain method is extended to three different widely complex-valued Volterra kernel-based models. Finally, computer simulations reveal that the suggested algorithms outperform the previously reported frequency-domain techniques in terms of convergence speed and tracking ability.
更多查看译文
关键词
Adaptive filter,Volterra kernel,Joint-normalized matrix,Frequency-domain,Mean-square analysis,Widely complex-valued model
AI 理解论文
溯源树
样例
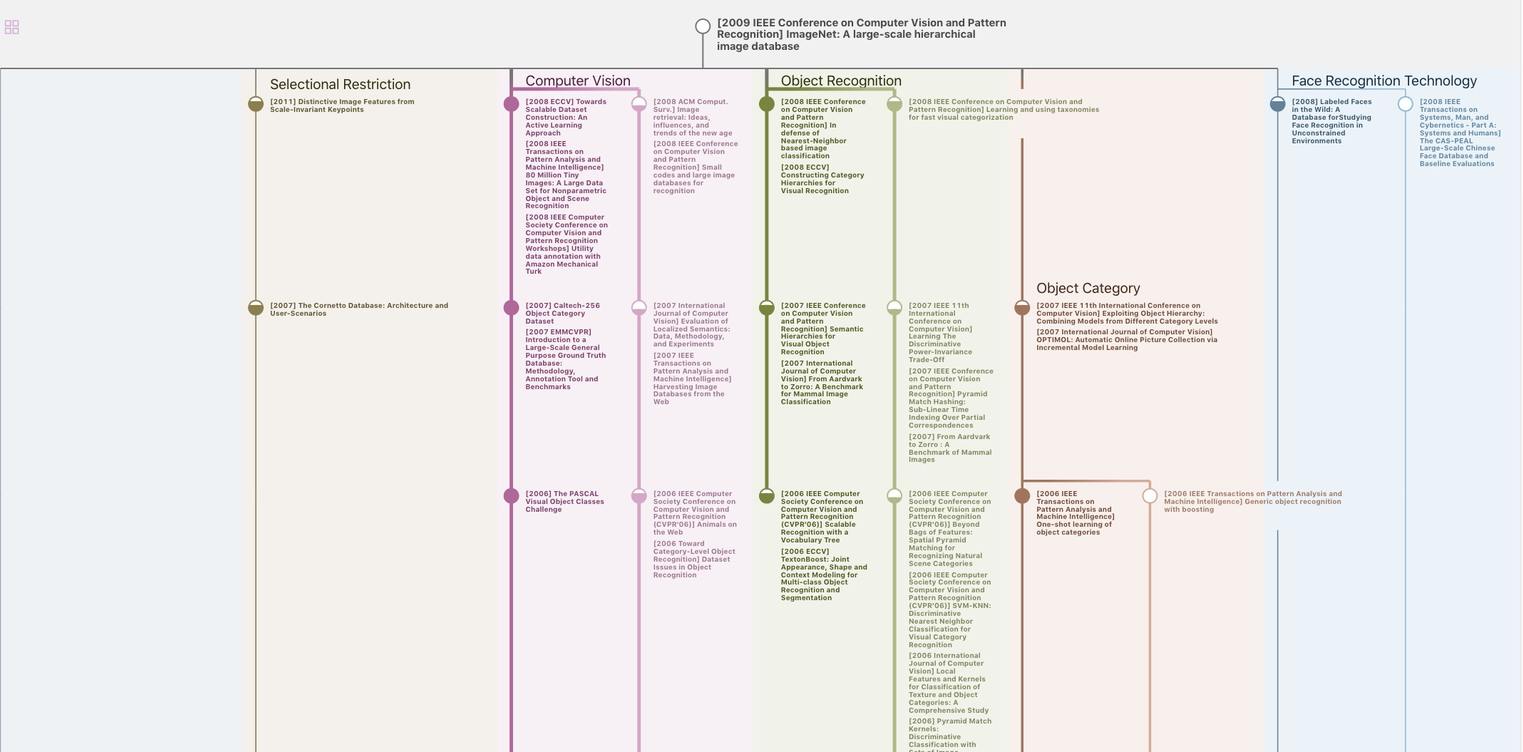
生成溯源树,研究论文发展脉络
Chat Paper
正在生成论文摘要