A fully convolutional neural network for comprehensive compartmentalization of abdominal adipose tissue compartments in MRI
COMPUTERS IN BIOLOGY AND MEDICINE(2023)
摘要
Background: Existing literature has highlighted structural, physiological, and pathological disparities among abdominal adipose tissue (AAT) sub-depots. Accurate separation and quantification of these sub-depots are crucial for advancing our understanding of obesity and its comorbidities. However, the absence of clear boundaries between the sub-depots in medical imaging data has challenged their separation, particularly for internal adipose tissue (IAT) sub-depots. To date, the quantification of AAT sub-depots remains challenging, marked by a time-consuming, costly, and complex process.Purpose: To implement and evaluate a convolutional neural network to enable granular assessment of AAT by compartmentalization of subcutaneous adipose tissue (SAT) into superficial subcutaneous (SSAT) and deep subcutaneous (DSAT) adipose tissue, and IAT into intraperitoneal (IPAT), retroperitoneal (RPAT), and paraspinal (PSAT) adipose tissue.Material and methods: MRI datasets were retrospectively collected from Singapore Preconception Study for LongTerm Maternal and Child Outcomes (S-PRESTO: 389 women aged 31.4 +/- 3.9 years) and Singapore Adult Metabolism Study (SAMS: 50 men aged 28.7 +/- 5.7 years). For all datasets, ground truth segmentation masks were created through manual segmentation. A Res-Net based 3D-UNet was trained and evaluated via 5-fold cross-validation on S-PRESTO data (N symbolscript 300). The model's final performance was assessed on a hold-out (N symbolscript 89) and an external test set (N symbolscript 50, SAMS).Results: The proposed method enabled reliable segmentation of individual AAT sub-depots in 3D MRI volumes with high mean Dice similarity scores of 98.3%, 97.2%, 96.5%, 96.3%, and 95.9% for SSAT, DSAT, IPAT, RPAT, and PSAT respectively.Conclusion: Convolutional neural networks can accurately sub-divide abdominal SAT into SSAT and DSAT, and abdominal IAT into IPAT, RPAT, and PSAT with high accuracy. The presented method has the potential to significantly contribute to advancements in the field of obesity imaging and precision medicine.
更多查看译文
关键词
Water-fat MRI,Abdominal fat segmentation,Deep learning,Convolutional neural network
AI 理解论文
溯源树
样例
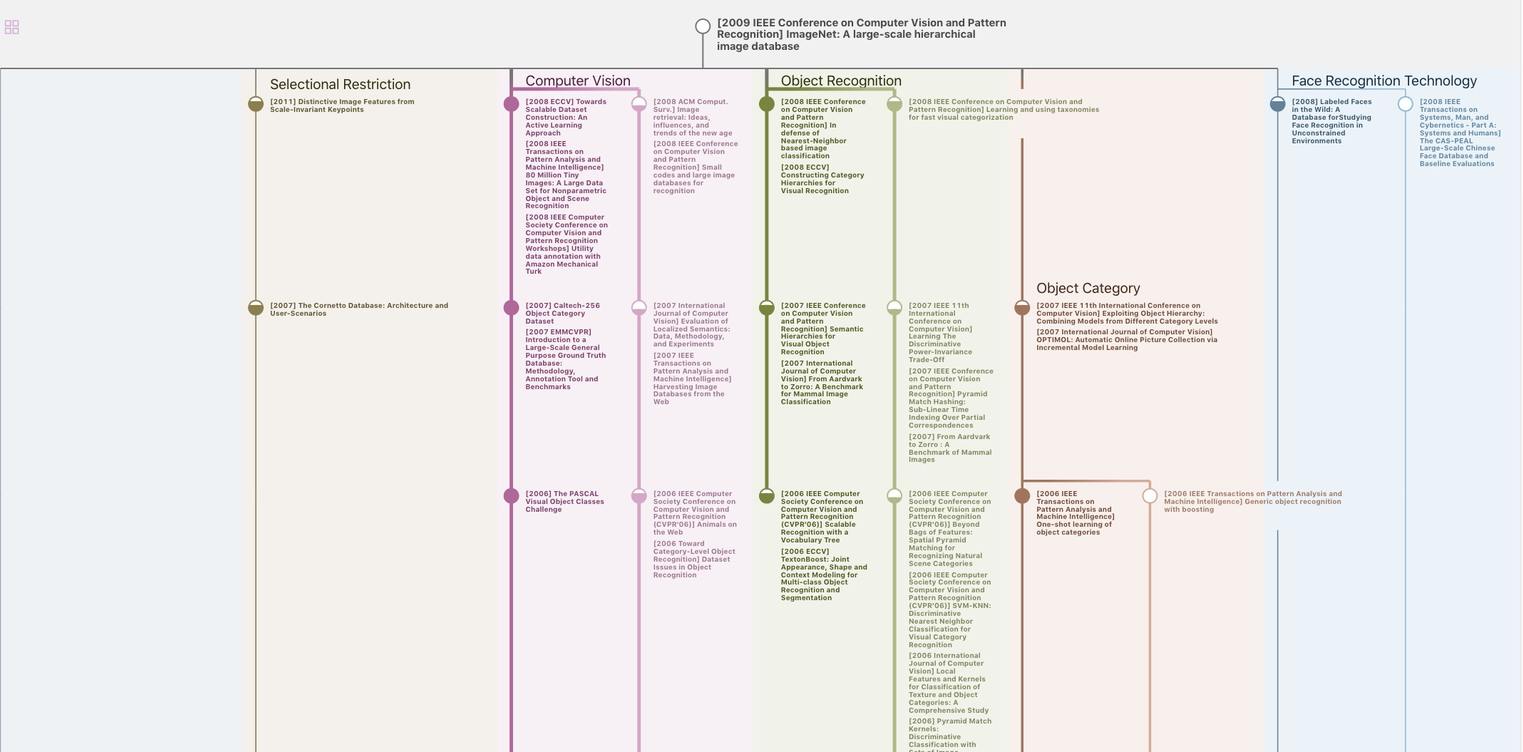
生成溯源树,研究论文发展脉络
Chat Paper
正在生成论文摘要