Semantic Face Segmentation Using Convolutional Neural Networks with a Supervised Attention Module
IEEE access(2023)
摘要
A self-attention module is often used in image segmentation tasks such as facial part segmentation. Because the self-attention module weights the features at each position using the weighted sum of features at all positions obtained by the middle layer of a convolutional neural network (CNN), the target regions for the segmentation might not be weighted sufficiently. The purpose of this study was to develop a semantic segmentation method for facial parts using a CNN with a supervised attention module that focuses on facial part enhancement. To improve the segmentation accuracy of the facial parts, we propose a new supervised attention module that can enhance features corresponding to pixels with the same class labels on input images and then incorporated it into the CNN. In this study, ResNet-FCN with skip connections was used as the baseline CNN model, and the CelebA Mask-HQ dataset was used for the network training and evaluation of the network. The mean intersection over union (IoU) and Dice index for the proposed network were greater than those for ResNet-FCN, SegNet, and PSPNet without the self-attention module, non-local neural networks with the traditional self-attention module, Segformer, and U-Segformer-Hyper with a Transformer. The proposed network achieved a high mean IoU and Dice index, and hence will be useful for segmenting facial parts.
更多查看译文
关键词
Supervised attention module,semantic segmentation,convolutional neural network,face image
AI 理解论文
溯源树
样例
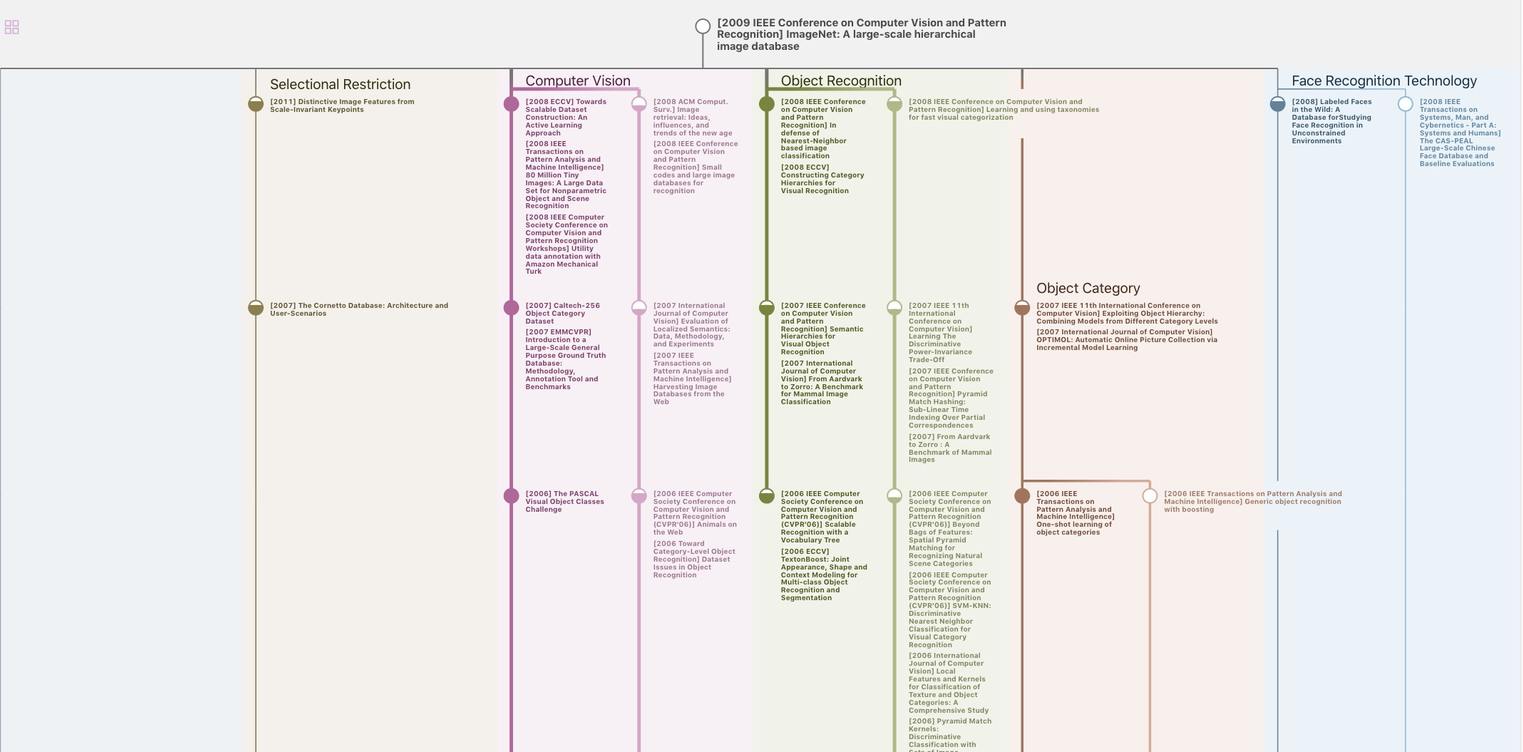
生成溯源树,研究论文发展脉络
Chat Paper
正在生成论文摘要