Generating Labeled Multiple Attribute Trajectory Data with Selective Partial Anonymization Based on Exceptional Conditional Generative Adversarial Network
IEEE access(2023)
Abstract
Trajectory data generated in location-based service environments contain highly sensitive personal information, making them a prime target for privacy attacks. At the same time, however, valuable statistical information can be obtained from such private data. Optimizing this tradeoff between utility and privacy presents a challenge. This study introduces a novel method for partially anonymizing sensitive areas using a conditional generative adversarial network. The proposed method enables the learning of complex spatial, temporal, and categorical features of the selected sensitive area through the utilization of our condition label structure and loss function. In this study, we evaluate and analyze the contents by considering the spatial-temporal characteristics and dividing them into spatial usability and temporal usability. The experimental results demonstrate that the proposed method outperforms related models that employ generative adversarial networks. We achieved high scores in a majority of spatial evaluation items while also discussing the aspects that obtained relatively low scores.
MoreTranslated text
Key words
Generative adversarial network,privacy protection,trajectory data,generating data,exceptional conditional
AI Read Science
Must-Reading Tree
Example
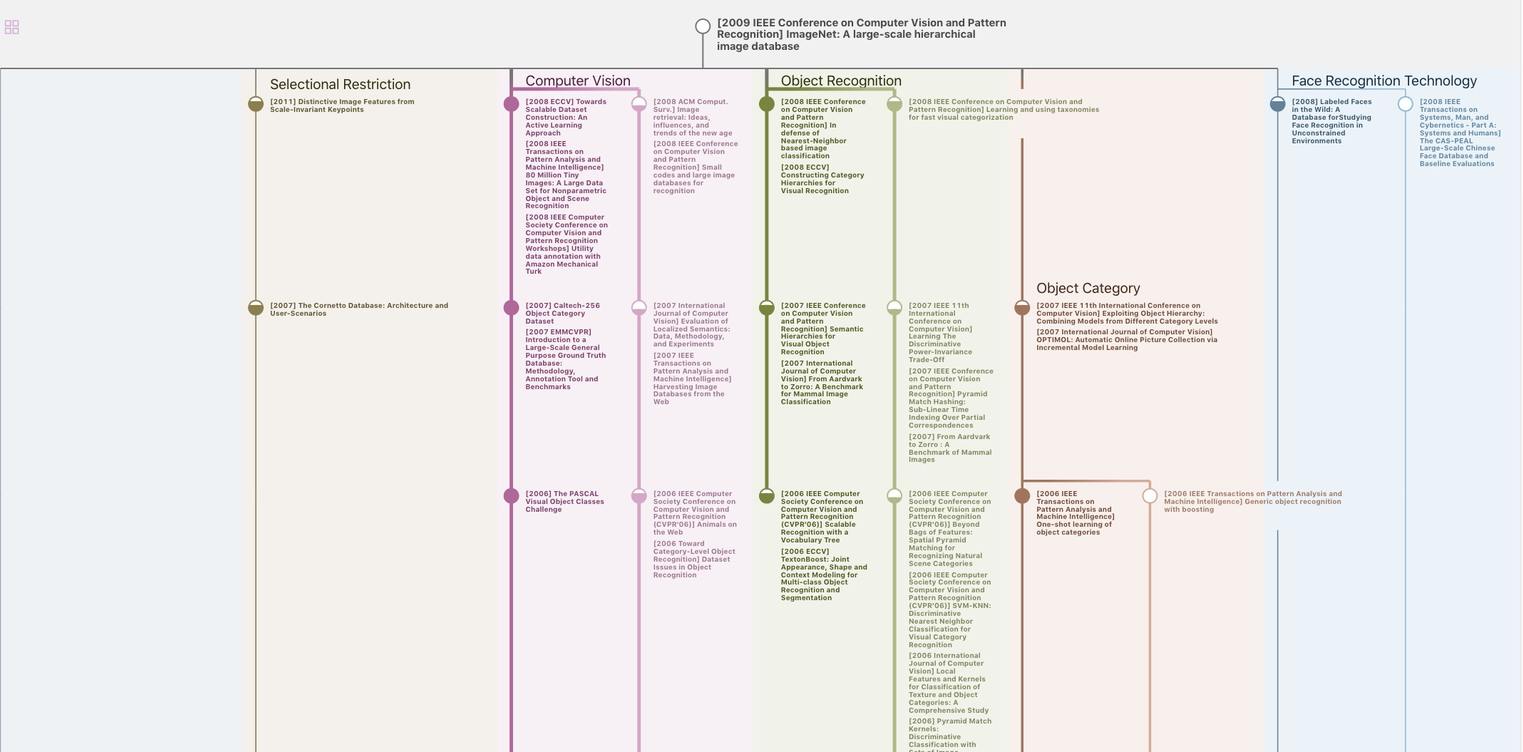
Generate MRT to find the research sequence of this paper
Chat Paper
Summary is being generated by the instructions you defined