Bayesian Optimization for Personalized Dose-Finding Trials with Combination Therapies
arXiv (Cornell University)(2023)
摘要
Identification of optimal dose combinations in early phase dose-finding
trials is challenging, due to the trade-off between precisely estimating the
many parameters required to flexibly model the possibly non-monotonic
dose-response surface, and the small sample sizes in early phase trials. This
difficulty is even more pertinent in the context of personalized dose-finding,
where patient characteristics are used to identify tailored optimal dose
combinations. To overcome these challenges, we propose the use of Bayesian
optimization for finding optimal dose combinations in standard ("one size fits
all") and personalized multi-agent dose-finding trials. Bayesian optimization
is a method for estimating the global optima of expensive-to-evaluate objective
functions. The objective function is approximated by a surrogate model,
commonly a Gaussian process, paired with a sequential design strategy to select
the next point via an acquisition function. This work is motivated by an
industry-sponsored problem, where focus is on optimizing a dual-agent therapy
in a setting featuring minimal toxicity. To compare the performance of the
standard and personalized methods under this setting, simulation studies are
performed for a variety of scenarios. Our study concludes that taking a
personalized approach is highly beneficial in the presence of heterogeneity.
更多查看译文
关键词
optimization,therapies,dose-finding
AI 理解论文
溯源树
样例
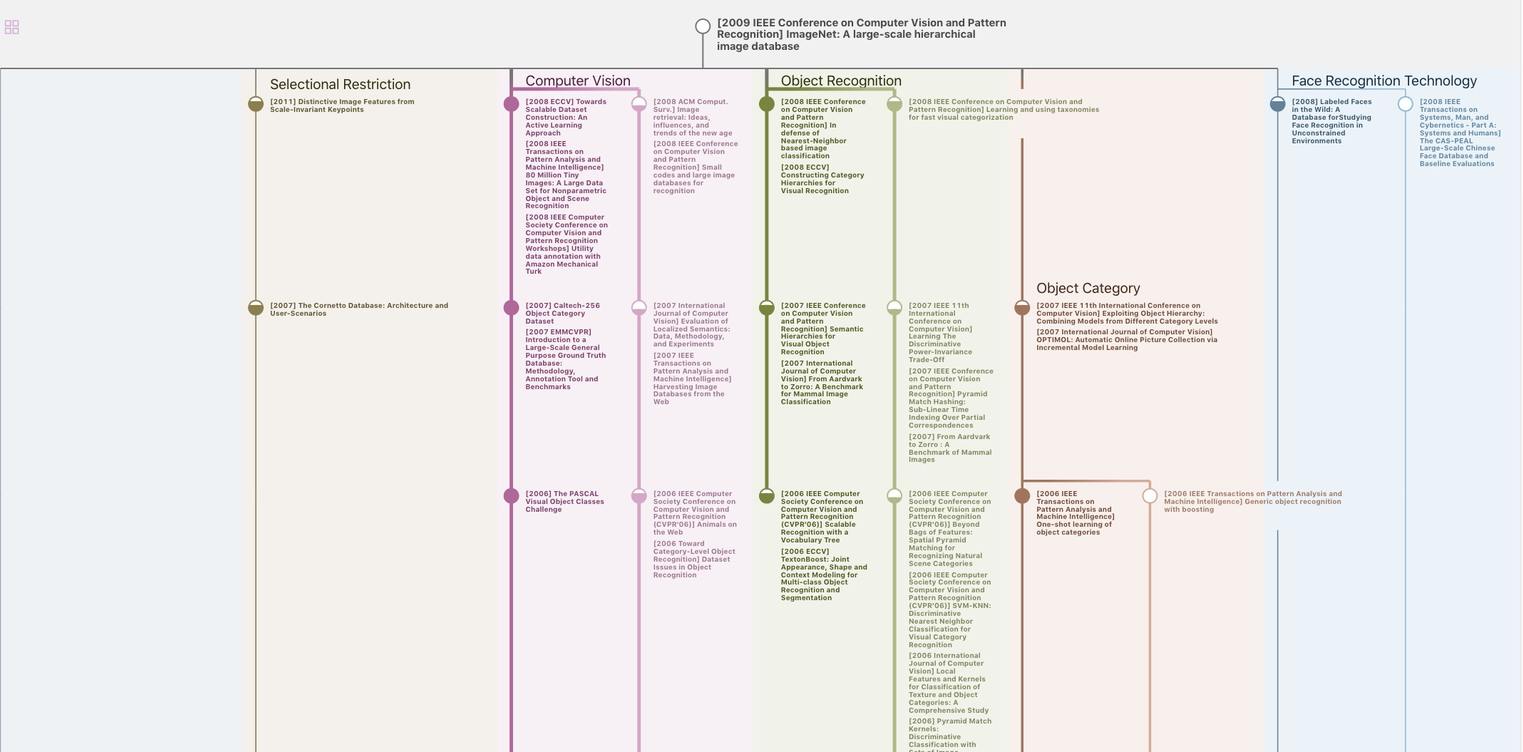
生成溯源树,研究论文发展脉络
Chat Paper
正在生成论文摘要