PETA: Evaluating the Impact of Protein Transfer Learning with Sub-word Tokenization on Downstream Applications
CoRR(2023)
摘要
Large protein language models are adept at capturing the underlying evolutionary information in primary structures, offering significant practical value for protein engineering. Compared to natural language models, protein amino acid sequences have a smaller data volume and a limited combinatorial space. Choosing an appropriate vocabulary size to optimize the pre-trained model is a pivotal issue. Moreover, despite the wealth of benchmarks and studies in the natural language community, there remains a lack of a comprehensive benchmark for systematically evaluating protein language model quality. Given these challenges, PETA trained language models with 14 different vocabulary sizes under three tokenization methods. It conducted thousands of tests on 33 diverse downstream datasets to assess the models' transfer learning capabilities, incorporating two classification heads and three random seeds to mitigate potential biases. Extensive experiments indicate that vocabulary sizes between 50 and 200 optimize the model, whereas sizes exceeding 800 detrimentally affect the model's representational performance. Our code, model weights and datasets are available at https://github.com/ginnm/ProteinPretraining.
更多查看译文
关键词
protein transfer learning,tokenization,sub-word
AI 理解论文
溯源树
样例
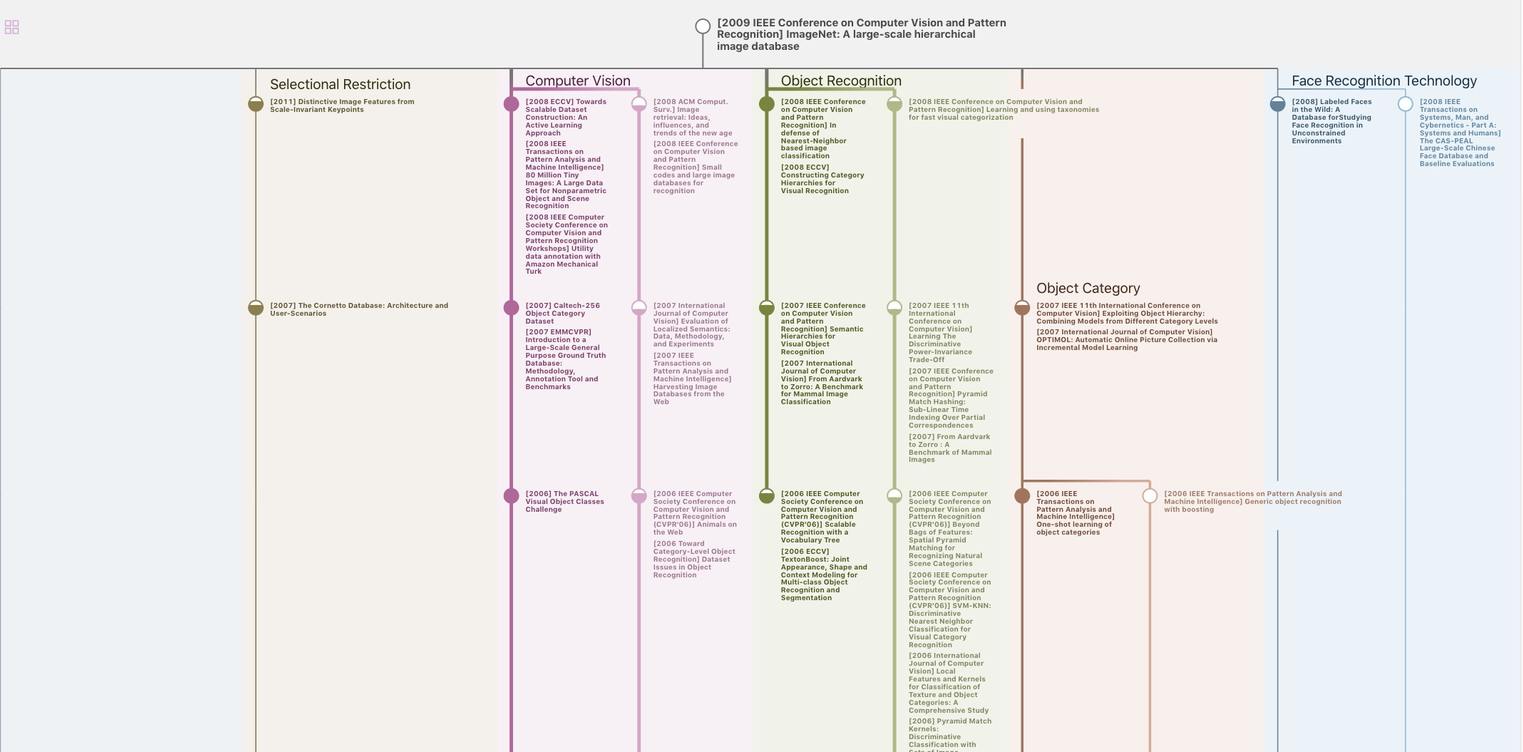
生成溯源树,研究论文发展脉络
Chat Paper
正在生成论文摘要