Goals are Enough: Inducing AdHoc cooperation among unseen Multi-Agent systems in IMFs
2024 IEEE 21ST CONSUMER COMMUNICATIONS & NETWORKING CONFERENCE, CCNC(2024)
摘要
Intent-based management will play a critical role in achieving customers' expectations in the next-generation mobile networks. Traditional methods cannot perform efficient resource management since they tend to handle each expectation independently. Existing approaches, e.g., based on multi-agent reinforcement learning (MARL) allocate resources in an efficient fashion when there are conflicting expectations on the network slice. However, in reality, systems are often far more complex to be addressed by a standalone MARL formulation. Often there exists a hierarchical structure of intent fulfillment where multiple pre-trained, self-interested agents may need to be further orchestrated by a supervisor or controller agent. Such agents may arrive in the system adhoc, which then needs to be orchestrated along with other available agents. This is especially true for networks evolving with time as components can get added incrementally. Retraining the whole system every time is often infeasible given the associated time and cost. Given the challenges, such adhoc coordination of pre-trained systems could be achieved through an intelligent supervisor agent which incentivizes pre-trained RL/MARL agents through sets of dynamic contracts (goals or bonuses) and encourages them to act as a cohesive unit towards fulfilling a global expectation. Some approaches use a rule-based supervisor agent and deploy the hierarchical constituent agents sequentially, based on human-coded rules. In the current work, we propose a framework whereby pre-trained agents can be orchestrated in parallel leveraging an AI-based supervisor agent. For this, we propose to use Adhoc-Teaming approaches which assign optimal goals to the MARL agents and incentivize them to exhibit certain desired behaviours. Results on the network emulator show that the proposed approach results in faster and improved fulfilment of expectations when compared to rule-based approaches and even generalizes to changes in environments.
更多查看译文
关键词
multi-agent reinforcement learning,adhoc-teaming approach,goal assignment
AI 理解论文
溯源树
样例
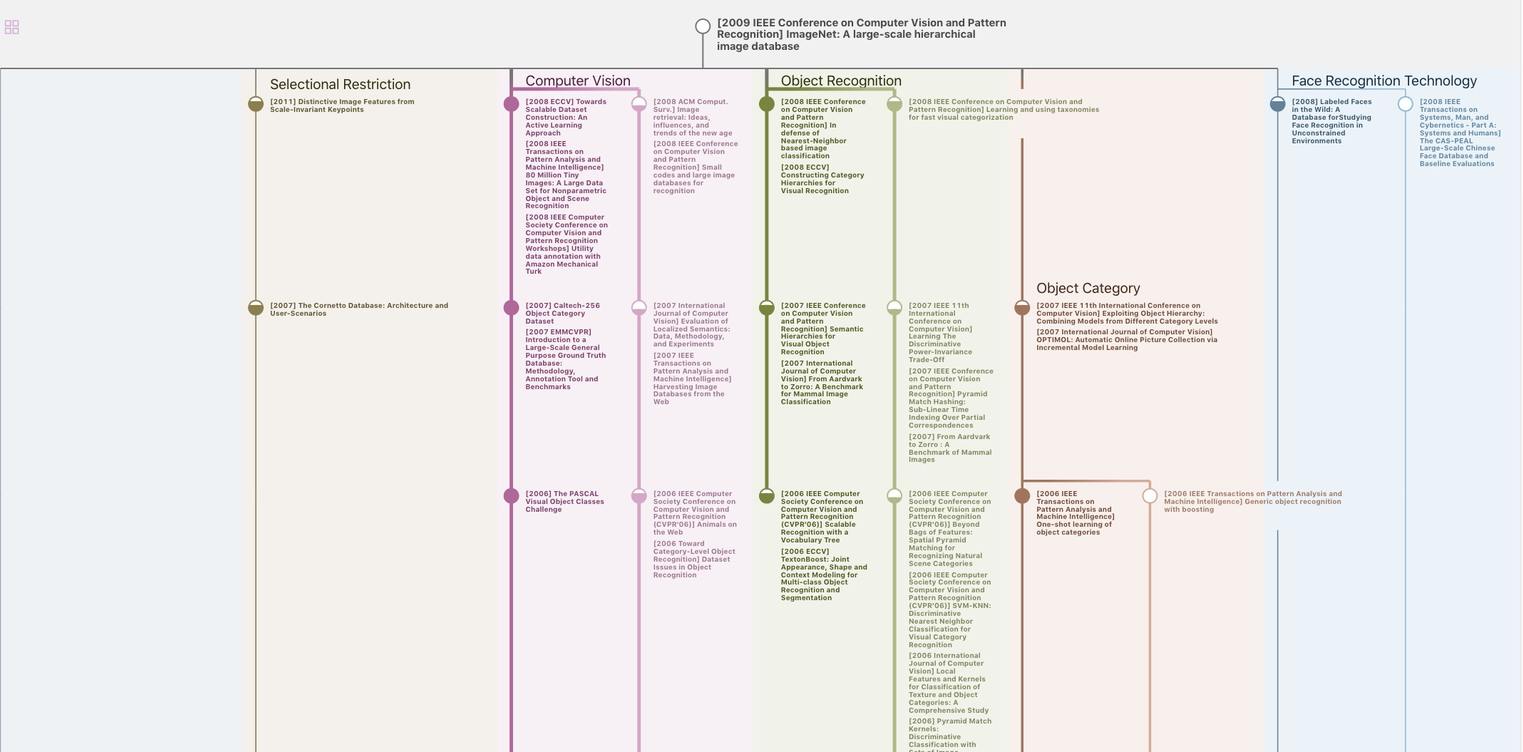
生成溯源树,研究论文发展脉络
Chat Paper
正在生成论文摘要