Deep Learning for Detection of Pneumothorax and Pleural Effusion on Chest Radiographs
Journal of Thoracic Imaging(2023)
摘要
Purpose: To study the performance of artificial intelligence (AI) for detecting pleural pathology on chest radiographs (CXRs) using computed tomography as ground truth. Patients and Methods: Retrospective study of subjects undergoing CXR in various clinical settings. Computed tomography obtained within 24 hours of the CXR was used to volumetrically quantify pleural effusions (PEfs) and pneumothoraxes (Ptxs). CXR was evaluated by AI software (INSIGHT CXR; Lunit) and by 3 second-year radiology residents, followed by AI-assisted reassessment after a 3-month washout period. We used the area under the receiver operating characteristics curve (AUROC) to assess AI versus residents’ performance and mixed-model analyses to investigate differences in reading time and interreader concordance. Results: There were 96 control subjects, 165 with PEf, and 101 with Ptx. AI-AUROC was noninferior to aggregate resident-AUROC for PEf (0.82 vs 0.86, P < 0.001) and Ptx (0.80 vs 0.84, P = 0.001) detection. AI-assisted resident-AUROC was higher but not significantly different from the baseline. AI-assisted reading time was reduced by 49% (157 vs 80 s per case, P = 0.009), and Fleiss kappa for Ptx detection increased from 0.70 to 0.78 (P = 0.003). AI decreased detection error for PEf (odds ratio = 0.74, P = 0.024) and Ptx (odds ratio = 0.39, P < 0.001). Conclusion: Current AI technology for the detection of PEf and Ptx on CXR was noninferior to second-year resident performance and could help decrease reading time and detection error.
更多查看译文
关键词
pneumothorax,pleural effusion,deep learning,detection
AI 理解论文
溯源树
样例
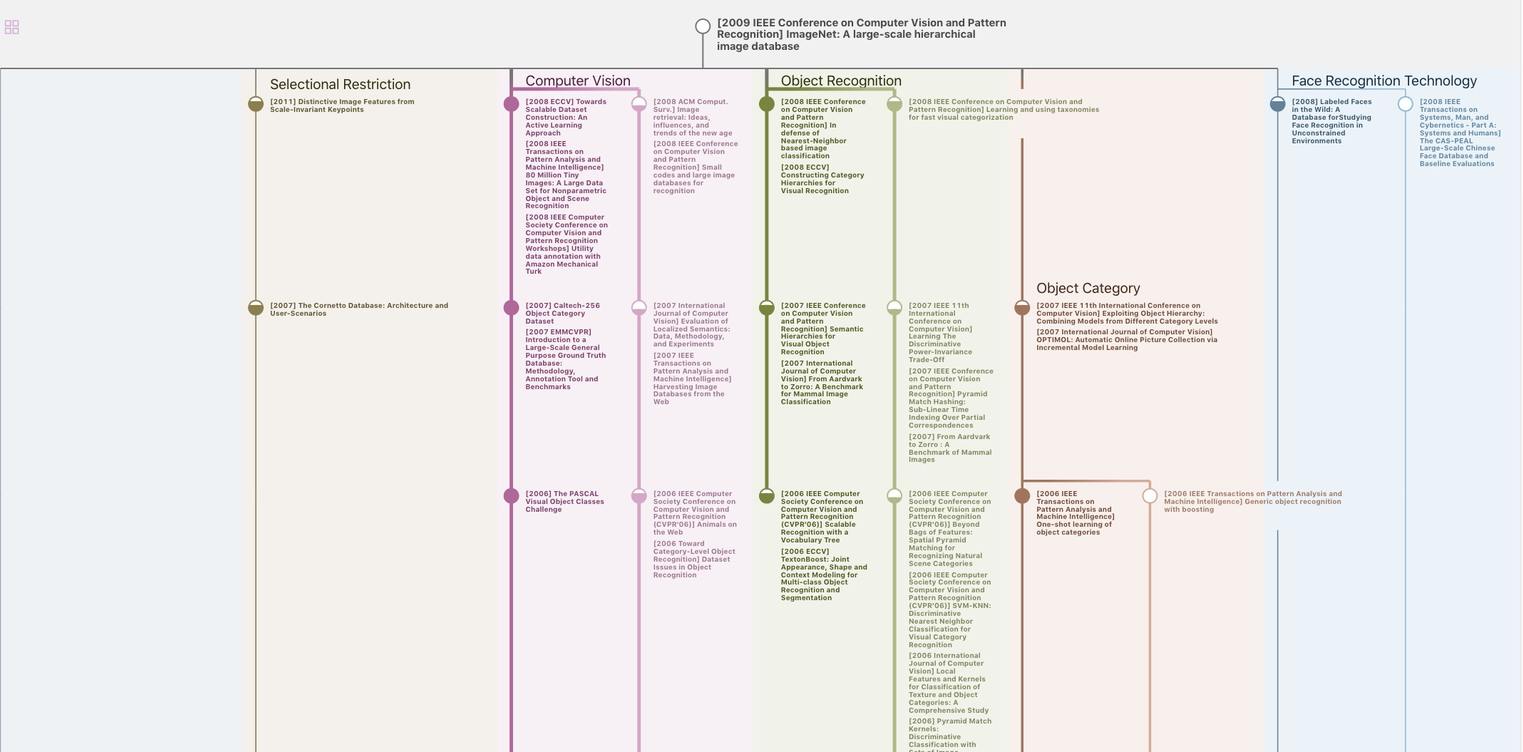
生成溯源树,研究论文发展脉络
Chat Paper
正在生成论文摘要